Geographically weighted regression modelling of the spatial association between malaria cases and environmental factors in Cameroon.
Research Square (Research Square)(2019)
摘要
Abstract Background: Studies have illustrated the association of malaria cases with environmental factors in Cameroon but limited in addressing how these factors vary in space for timely public health interventions. Thus, we want to find the spatial variability between malaria hotspot cases and environmental predictors using Geographically weighted regression (GWR) spatial modelling technique. Methods: The global Ordinary least squares(OLS) in the modelling spatial relationships tool in ArcGIS 10.3. was used to select candidate explanatory environmental variables for a properly specified GWR model. The local GWR model used the global OLS candidate variables to examine, predict and explore the spatial variability between environmental factors and malaria hotspot cases generated from Getis-Ord Gi* statistical analysis. Results: The OLS candidate environmental variable coefficients were statistically significant ( adjusted R 2 = 22.3% and p < 0.01) for a properly specified GWR model. The GWR model identified a strong spatial association between malaria cases and rainfall, vegetation index, population density, and drought episodes in most hotspot areas and a weak correlation with aridity and proximity to water with an overall model performance of 0.243 (adjusted R 2 = 24.3%). Conclusion: The generated GWR maps suggest that for policymakers to eliminate malaria in Cameroon, there should be the creation of malaria outreach programs and further investigations in areas where the environmental variables showed strong spatial associations with malaria hotspot cases . Keywords: Geographically weighted regression, Ordinary least squares, malaria, spatial statistics, mapping, Geographical information systems.
更多查看译文
关键词
malaria cases,cameroon,spatial association,environmental factors
AI 理解论文
溯源树
样例
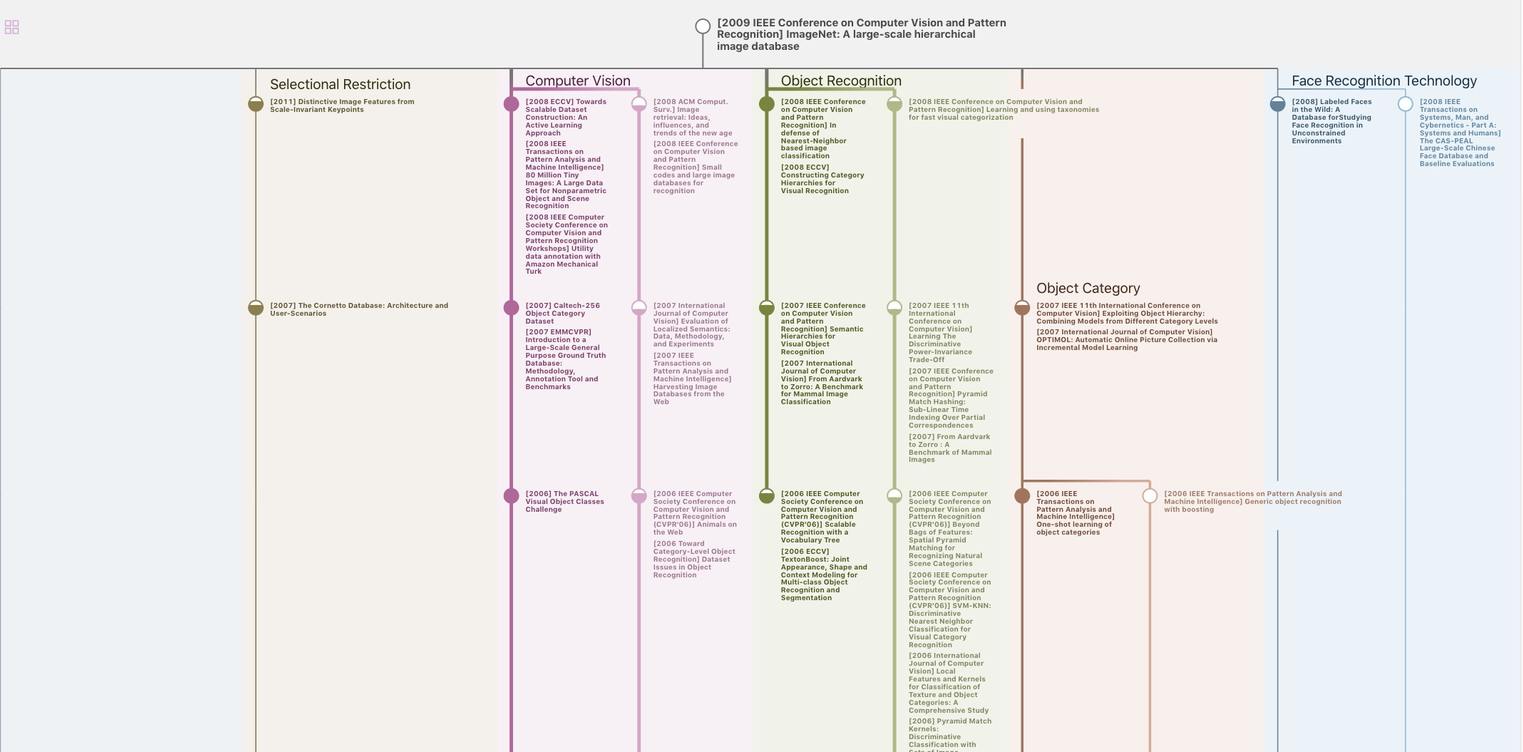
生成溯源树,研究论文发展脉络
Chat Paper
正在生成论文摘要