Downscaling Land Surface Temperature at Farm-Scale Using Multi-Model Approaches over Diverse Agro Climatic Zones
crossref(2024)
摘要
Land surface temperature (LST) is a critical parameter for land surface and atmospheric interactions. However, the applicability of current land surface temperature estimates for field-level hydrological, agricultural, and ecological operations is still challenging due to their coarse spatiotemporal resolution. In the current article, we have downscaled 100 m LST to 10 m by using high-resolution Sentinel-1,2 optical-microwave data and three different models- 1) Thermal Sharpening (TsHARP); 2) Thin Plate Spline (TPS); and 3) Random Forest (RF). The extensive analysis was performed at agricultural farms in the semi-arid (IARI) region of India during the winter and summer seasons of 2020-21 and 2021-22, arid (CAZRI) and per-humid (UBKV) region (2021-2022). The calibration accuracy of the RF model was shown to better agreement with the coefficient of determination (R2) of 0.982, root means square error (RMSE) 0.181 and normalized root means square error (RMSE) 0.061 with their lower values of standard errors over three diverse agro-climatic zones. The findings demonstrate that the validation accuracy of models' varied according to the agroclimatic zones, although RF and TPS consistently outperformed as compared to the TsHARP models.
更多查看译文
AI 理解论文
溯源树
样例
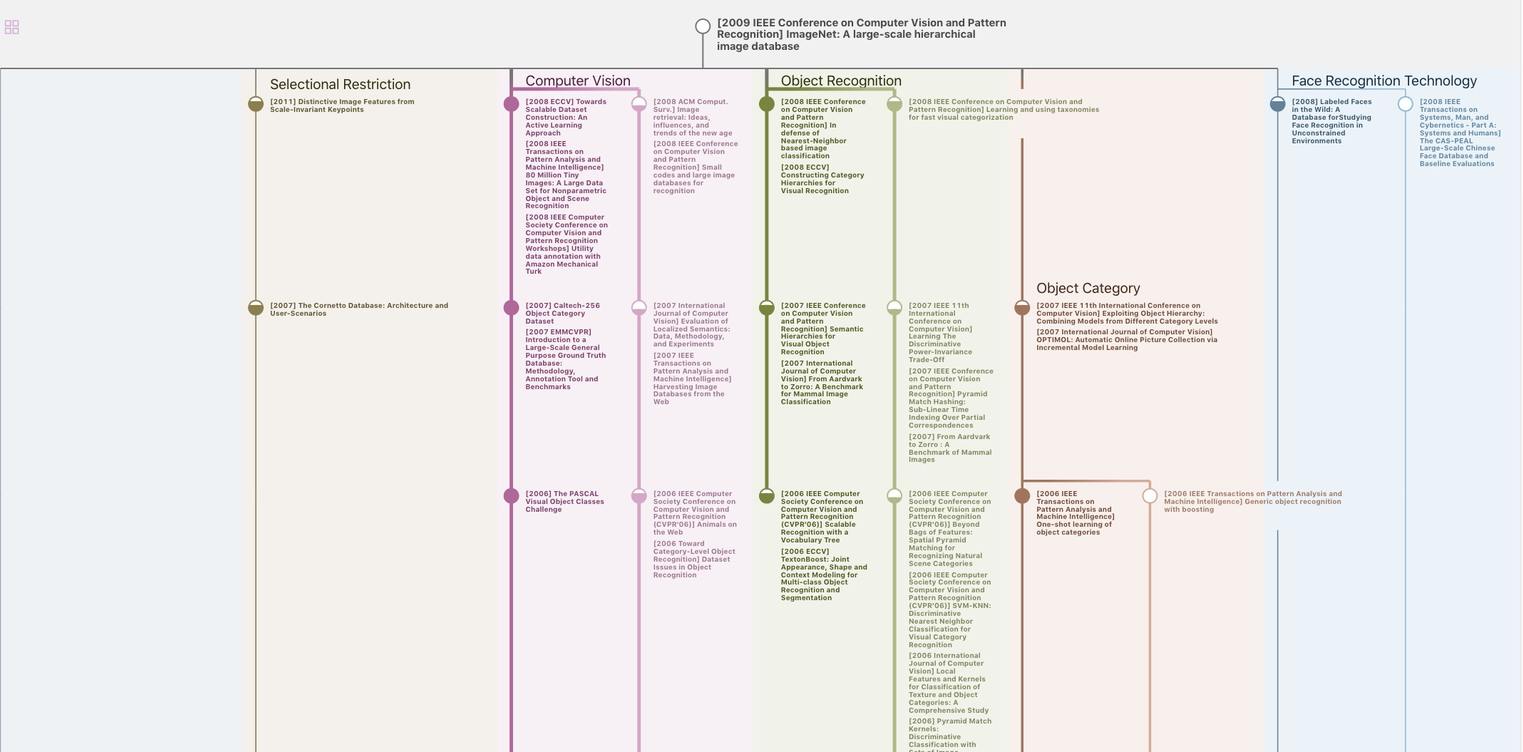
生成溯源树,研究论文发展脉络
Chat Paper
正在生成论文摘要