Deep Sigma Point Processes-Assisted Chance-Constrained Power System Transient Stability Preventive Control
IEEE TRANSACTIONS ON POWER SYSTEMS(2024)
摘要
This paper proposes a deep sigma point processes (DSPP)-assisted chance-constrained power system transient stability preventive control method to deal with uncertain renewable energy and loads-induced stability risk. The traditional transient stability-constrained preventive control is reformulated as a chance-constrained optimization problem. To deal with the computational bottleneck of the time-domain simulation-based probabilistic transient stability assessment, the DSPP is developed. DSPP is a parametric Bayesian approach that allows us to predict system transient stability with high computational efficiency while accurately quantifying the confidence intervals of the predictions that can be used to inform system instability risk. To this end, with a given preset confidence probability, we embed DSPP into the primal dual interior point method to help solve the chance-constrained preventive control problem, where the corresponding Jacobian and Hessian matrices are derived. Comparison results with other existing methods show that the proposed method can significantly speed up preventive control while maintaining high accuracy and convergence.
更多查看译文
关键词
Power system stability,Transient analysis,Stability criteria,Probabilistic logic,Thermal stability,Generators,Wind farms,Deep sigma point processes,power system stability,probabilistic transient stability prediction,chance-constrained optimization,renewable energy
AI 理解论文
溯源树
样例
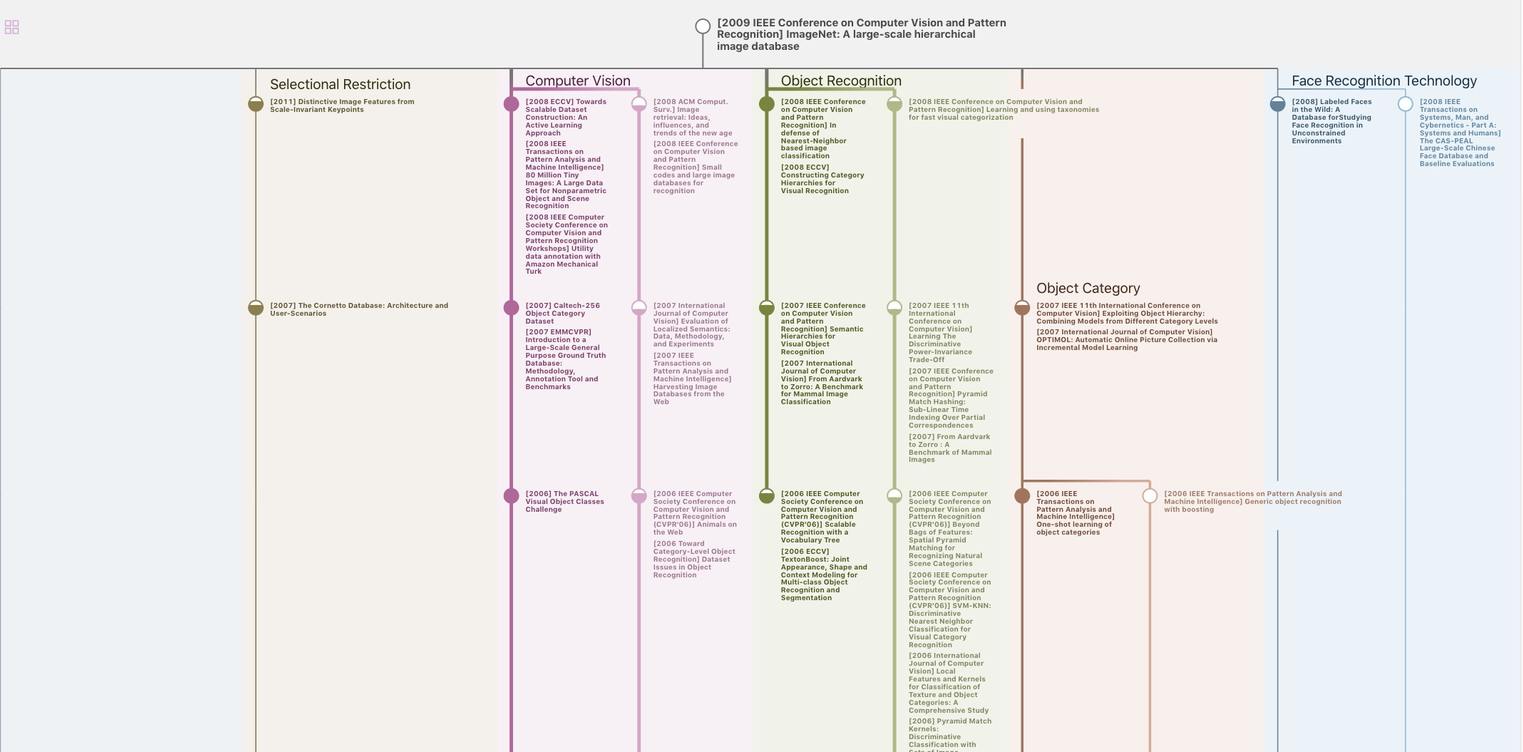
生成溯源树,研究论文发展脉络
Chat Paper
正在生成论文摘要