Abstract 2091: Open-source single-cell spatial image analysis pipeline for ten-plex immunofluorescence of histological prostate cancer tissue sections
Cancer Research(2023)
摘要
Abstract Introduction: Multiplexed fluorescence immunohistochemistry (mfIHC) allows the detection of multiple protein markers on the same histological tissue section, generating valuable information on specific cell subsets and cellular states in their spatial tissue context. Here, we demonstrate a proof-of-concept study for profiling different tumor microenvironment cell subsets in formalin-fixed paraffin-embedded prostate cancer tissue microarrays. Materials and methods: We applied automated mfIHC simultaneous staining of 31 slides with 3.5-µm sections using an Autostainer (Epredia, Kalamazoo, USA). The protocol included four rounds of cyclic staining and scanning of the slides with AxioScanZ.1 (Zeiss, Germany). Each cycle included DAPI for nuclear staining. Here, we applied this pipeline to detect the following targets: D2-40 (lymphatics), CD3 (T lymphocytes), CD56 (NK cells/neural cells/neuroendocrine cells), CD11b+ myeloid cells, PDGFRB (fibroblasts/pericytes), CD20 (B lymphocytes), CD31 (endothelium), CD45 (leukocytes), HLADR+ antigene-presenting cells, as well as a cocktail with PanCK and ECADH to detect epithelial cells. Three different prostate cancer TMA cohorts with n=907 patient samples were analyzed here using this approach.The scanned images were exported as Big TIFF individual channel whole-slide images. Individual TMA spot images were marked with Fiji ROI1 1-Click Tool macro (ImageJ 1.53t), and separate rounds were registered with Python (version 3.11.0, Python Software Foundation) using DAPI channel as reference. Nuclear segmentation was created with nucleAIzer trained modified Mask R-CNN model (https://github.com/lopaavol/nuclei_segmentation). All TMA spot images were run through image curation, where artefacts are excluded by machine learning using ilastik software (version 1.3.3post3). Then, CellProfiler (version 4.2.1) was applied to combine image curated tissue masks and segmented nuclei for cell-based channel intensity measurements. Finally, Jupyter Notebook was applied to classify cell subsets into specific phenotypes using intensity-based thresholding for each channel. Results and Conclusions: We present a detailed pipeline for 10-plex mfIHC with open-source single-cell image analysis tools. The approach can be applied to histological laboratories when combined with a standard five fluorescent channel scanner or imager and with basic computing skills. As the system applies secondary antibodies for the detection, it is flexible for optimizing new antibody panels, and the user is not limited to pre-labelled antibody panels often connected with commercial staining and imaging systems. The approach is especially suited for large-scale high-throughput tissue profiling studies when high n-values are needed for linking associated patient clinical data with spatial phenotype data. Citation Format: Jenni Säilä, Annabrita Schoonenberg, Katja Välimäki, Timo-Pekka Lehto, Carolin Stürenberg, Andrew Erickson, Antti Rannikko, Olli Kallioniemi, Lassi Paavolainen, Tuomas Mirtti, Teijo Pellinen. Open-source single-cell spatial image analysis pipeline for ten-plex immunofluorescence of histological prostate cancer tissue sections [abstract]. In: Proceedings of the American Association for Cancer Research Annual Meeting 2023; Part 1 (Regular and Invited Abstracts); 2023 Apr 14-19; Orlando, FL. Philadelphia (PA): AACR; Cancer Res 2023;83(7_Suppl):Abstract nr 2091.
更多查看译文
关键词
prostate cancer,spatial image analysis pipeline,single-cell single-cell,open-source,ten-plex
AI 理解论文
溯源树
样例
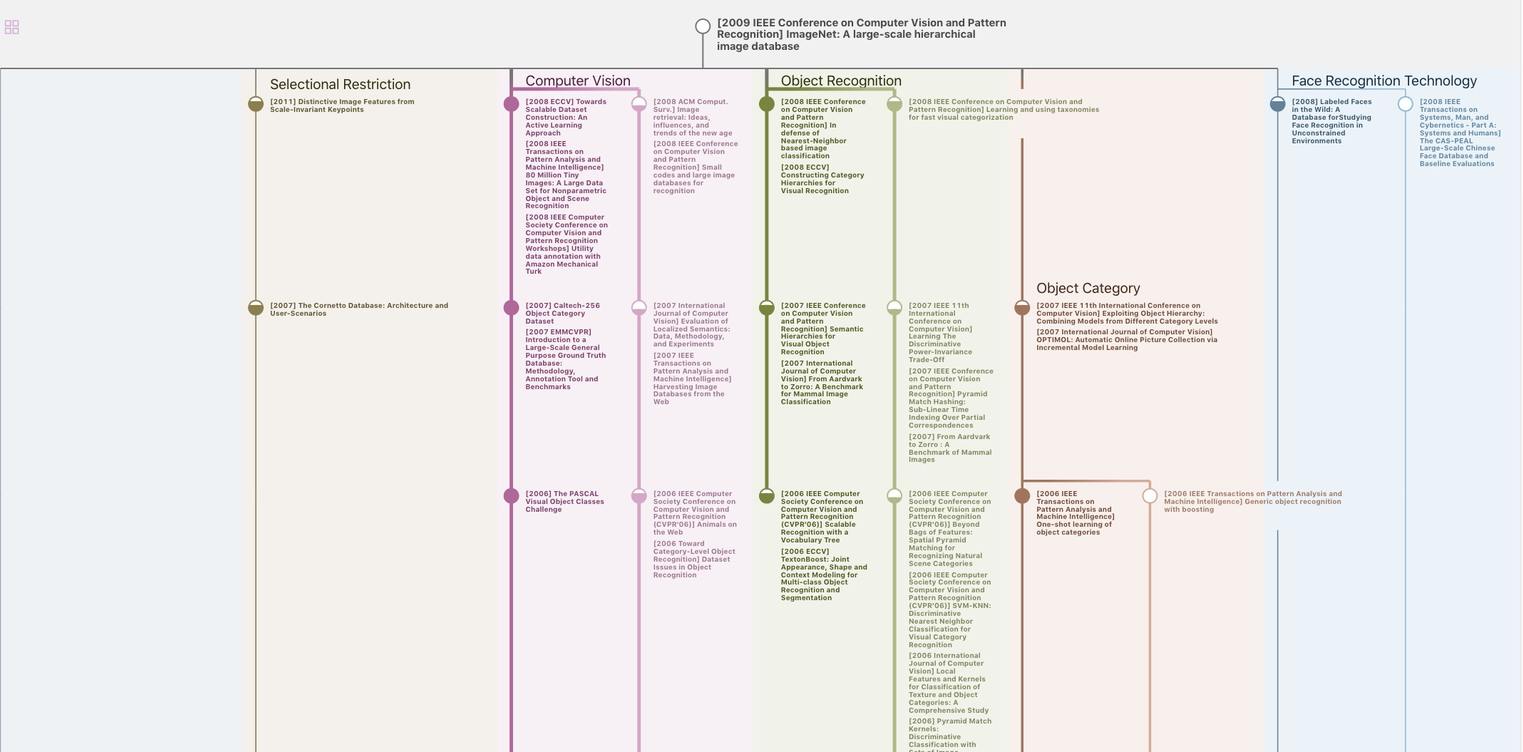
生成溯源树,研究论文发展脉络
Chat Paper
正在生成论文摘要