Ensembled Prediction Intervals for Causal Outcomes Under Hidden Confounding.
Conference on Causal Learning and Reasoning(2024)
摘要
Causal inference of exact individual treatment outcomes in the presence of hidden confounders is rarely possible. Recent work has extended prediction intervals with finite-sample guarantees to partially identifiable causal outcomes, by means of a sensitivity model for hidden confounding. In deep learning, predictors can exploit their inductive biases for better generalization out of sample. We argue that the structure inherent to a deep ensemble should inform a tighter partial identification of the causal outcomes that they predict. We therefore introduce an approach termed Caus-Modens, for characterizing causal outcome intervals by modulated ensembles. We present a simple approach to partial identification using existing causal sensitivity models and show empirically that Caus-Modens gives tighter outcome intervals, as measured by the necessary interval size to achieve sufficient coverage. The last of our three diverse benchmarks is a novel usage of GPT-4 for observational experiments with unknown but probeable ground truth.
更多查看译文
AI 理解论文
溯源树
样例
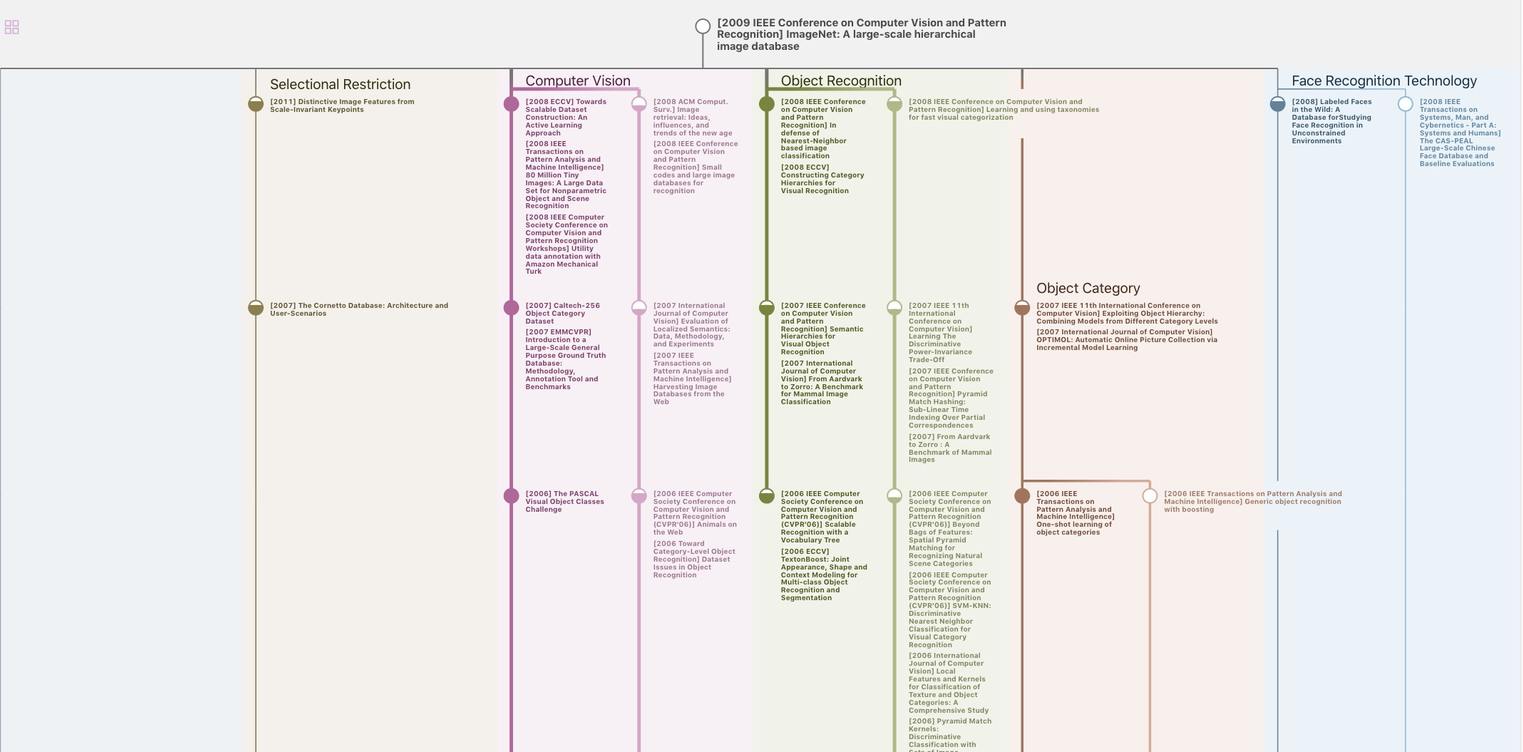
生成溯源树,研究论文发展脉络
Chat Paper
正在生成论文摘要