Review of using machine learning in secure IoT healthcare
Elsevier eBooks(2023)
摘要
The healthcare industry is experiencing a digital transformation through telehealth. As a result, users’ information is at risk of being compromised by intruders. Machine learning can provide the sector with reliable protection against potential threats to address security and privacy concerns. In this chapter, we explore possible machine-learning solutions to two security challenges in the telehealth system: continuous authentication and detecting insider attacks. Authentication is the process of confirming the identity of a device or a person before connecting to the system. One of the most effective authentication techniques in telehealth is to verify IoT devices constantly to enhance cybersecurity protection on an ongoing basis. Continuous authentication uses machine learning algorithms to monitor all telehealth network activities, from users and devices, and utilizes classification techniques to detect unauthorized activities. This method relies on the verification of the identity of registered users to avoid unauthorized access as a proactive approach to mitigate the security risks. The main advantage of using machine learning over traditional authentication methods is that it does not need the user's attention while being authenticated continuously over time. Another advantage is higher reliability and accuracy. The second security challenge is insider attacks. Insider attacks occur when a user is authenticated legitimately to the system and aims to perform malicious activities such as stealing confidential information. Machine learning-based outlier detection methods are often used to identify users’ abnormal behaviors and prevent further access to confidential information.
更多查看译文
关键词
iot,machine learning
AI 理解论文
溯源树
样例
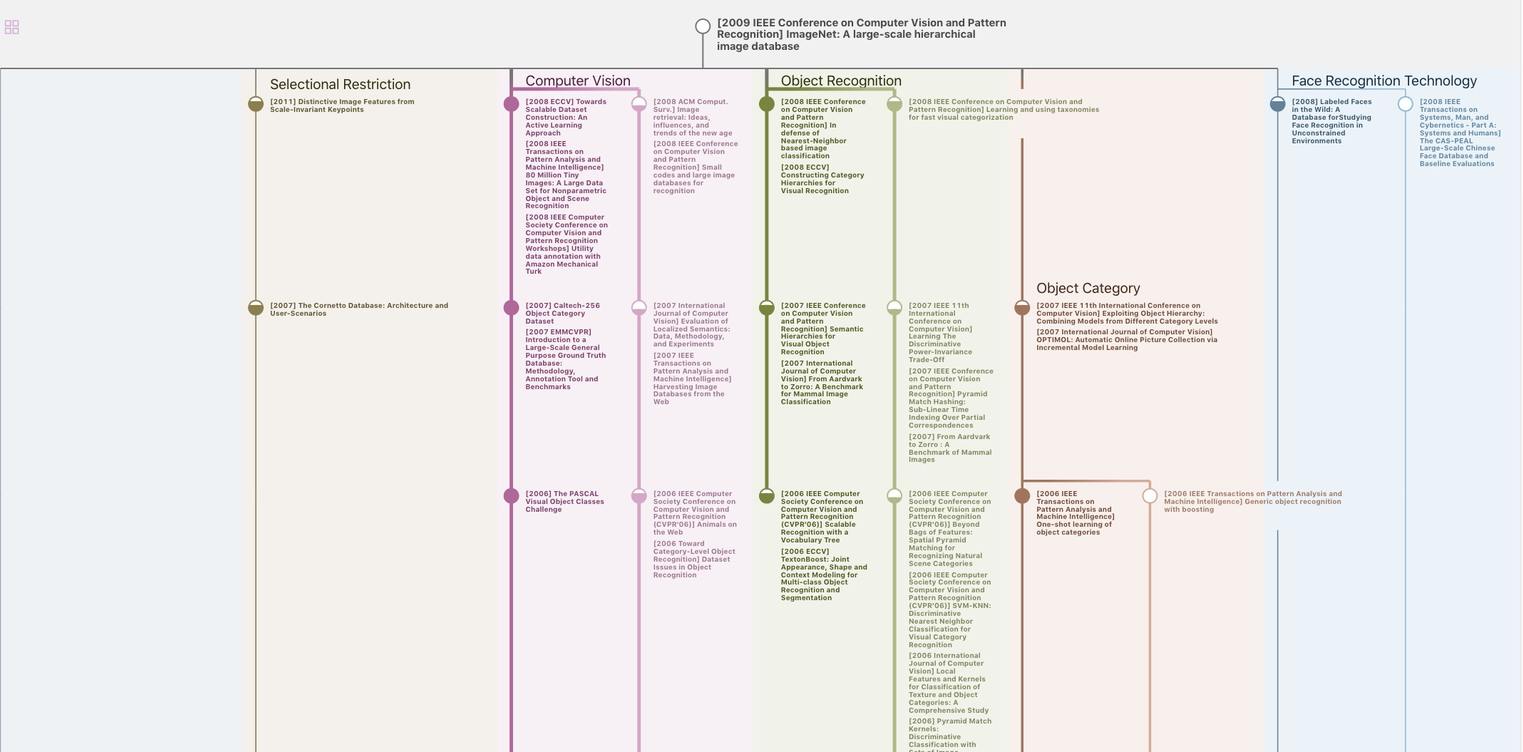
生成溯源树,研究论文发展脉络
Chat Paper
正在生成论文摘要