Deep learning and deep transfer learning-based OPM for FMF systems.
Phys. Commun.(2023)
摘要
Optical performance monitoring (OPM) is indispensable to guarantee stable and reliable operation in few-mode fiber (FMF)-based transmission. OPM consists of measuring optical phenomena such as generalized signal-to-noise ratio (GSNR) based on analytical models. GSNR comprises nonlinear interference (NLI) noise which can be calculated either by exact analytical models e.g., enhanced Gaussian noise (EGN) model which is accurate but computationally complex, or asymptotic analytical models e.g., closed-form EGN model which are approximate but computationally fast. In this paper, we employ deep learning (DL) as an accurate and fast alternative for OPM in FMF-based transmission. However, DL-based OPM requires a large dataset to achieve proper performance whilst it is very difficult and time-consuming to obtain a large field or synthetic dataset. Regarding this issue, we develop deep transfer learning (DTL) for OPM in FMF to realize a fast response requiring a small training dataset and a few training epochs despite various changes in system/link parameters such as launched power, fiber type, and the number of modes. Results show root mean squared error of GSNR estimation is less than 0.02 dB for DL and DTL-based OPM methods. Compared to DL-based OPM, DTL-based OPM records 3 and 5 times reduction in required training dataset size and the number of epochs, respectively which is beneficial for real-time applications & COPY; 2023 Elsevier B.V. All rights reserved.
更多查看译文
关键词
fmf systems,deep learning,learning-based
AI 理解论文
溯源树
样例
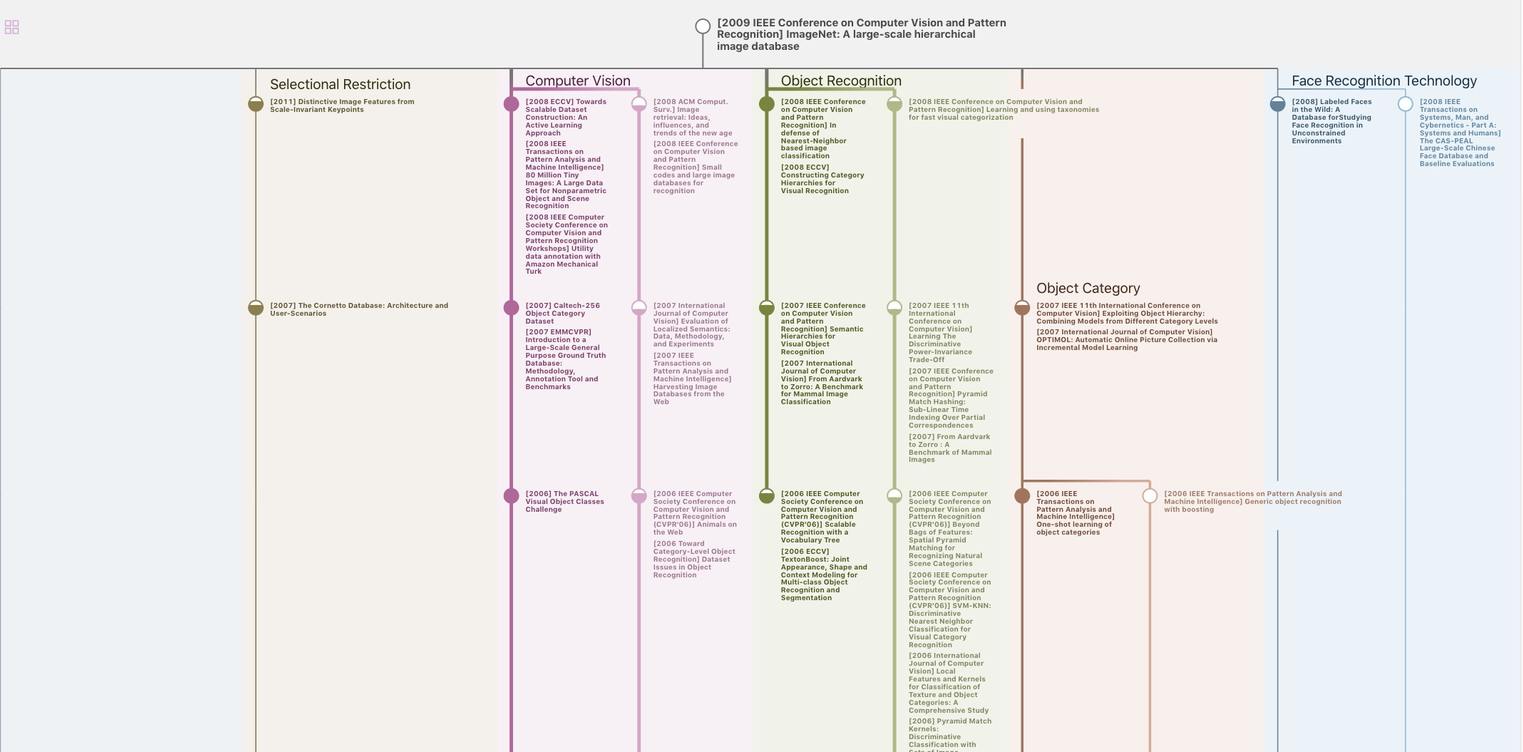
生成溯源树,研究论文发展脉络
Chat Paper
正在生成论文摘要