Context-aware and part alignment for visible-infrared person re-identification
Image and Vision Computing(2023)
摘要
Visible-infrared person re-identification (VI-ReID) is a challenging problem of matching a person from visible and infrared modalities. Existing researches adopt the last convolutional layer features of the off-the-shelf backbone network as the representation to refine, which is unable to represent the heterogeneous cross-modality features with discriminant information. In this paper, we propose a novel graph-based aggregation learning network (GALNet) with visual Transformer embedding to mine both multi-layer features and part-level contextual cues for VI-ReID. We propose a novel feature memory module (FMM) to reserve global discriminative features of the low layers with the correlation modeling ability of the graph convolution network (GCN) which is supplemented to the final person representation. To learn more discriminant part-level features, an attentive part aggregation module (PAM) is designed to mine part relationships, leveraging the self-attention mechanism of the Transformer. By fusing these components, the global-level, and part-level discriminant information can be utilized. Extensive experiments on SYSU-MM01 and RegDB benchmarks demonstrate the effectiveness of our proposed methods compared with several state-of-the-art methods.
更多查看译文
关键词
Cross-modality person re-identification,Attention mechanism,Image retrieval,Part-based model
AI 理解论文
溯源树
样例
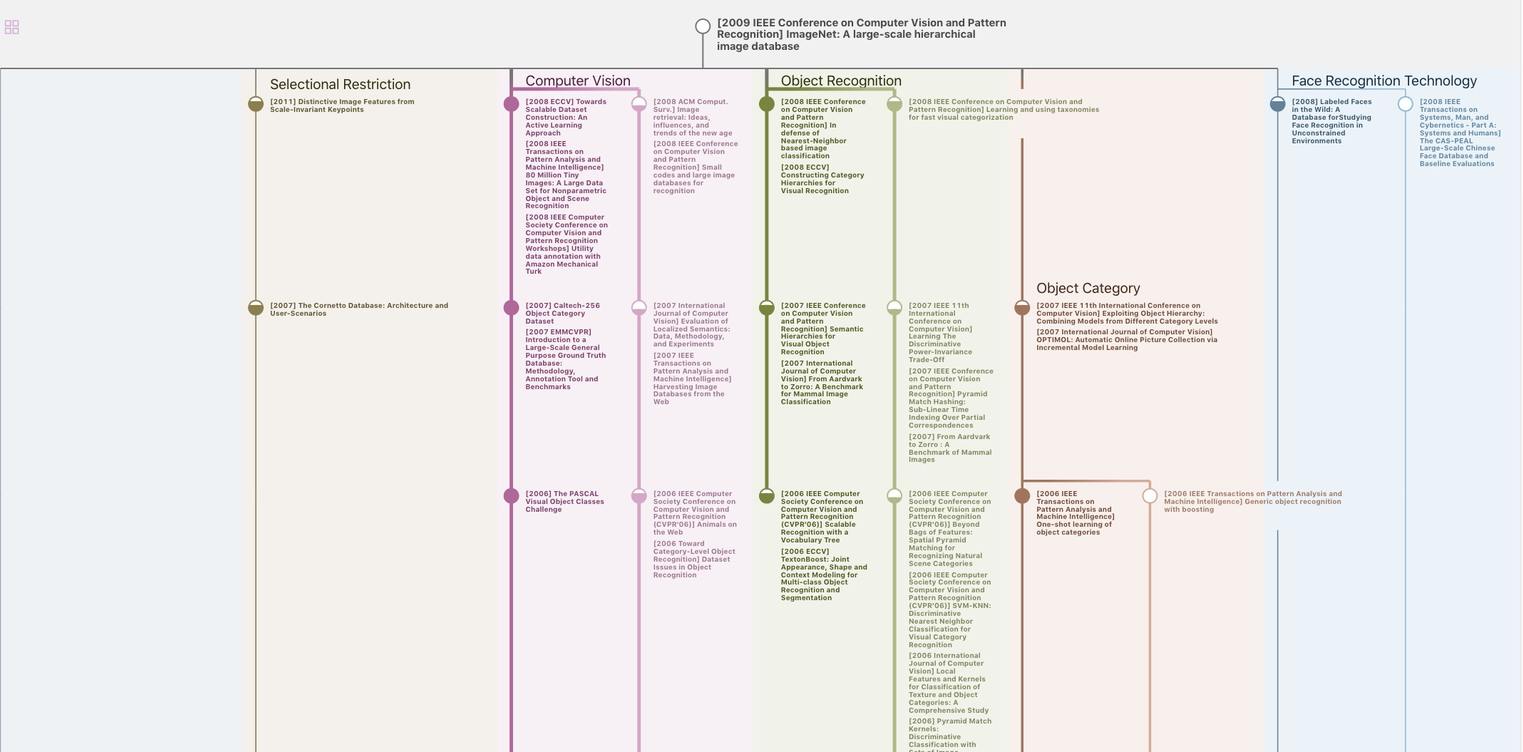
生成溯源树,研究论文发展脉络
Chat Paper
正在生成论文摘要