Importance-Based Neuron Selective Distillation for Interference Mitigation in Multilingual Neural Machine Translation.
KSEM (4)(2023)
摘要
Multilingual neural machine translation employs a single model to translate multiple languages, enabling efficient cross-lingual transferability through shared parameters. However, multilingual training suffers from negative language interference, especially interference with high-resource languages. Existing approaches generally use language-specific modules to distinguish heterogeneous characteristics among different languages but suffer from the parameter explosion problem. In this paper, we propose a “divide and conquer” multilingual translation training method based on the importance of neurons that can mitigate negative language interference effectively without adding additional parameters. The key technologies can be summarized as estimation, pruning, distillation, and fine-tuning. Specifically, we estimate the importance of existing pre-trained model neurons, dividing them into the important ones representing general knowledge of each language and the unimportant ones representing individual knowledge of each low-resource language. Then, we prune the pre-trained model, retaining only the important neurons, and train the pruned model supervised by the original complete model via selective distillation to compensate for some performance loss due to unstructured pruning. Finally, we restore the pruned neurons and only fine-tune them. Experimental results on several language pairs demonstrate the effectiveness of the proposed method.
更多查看译文
关键词
neuron selective distillation,multilingual neural machine translation,importance-based
AI 理解论文
溯源树
样例
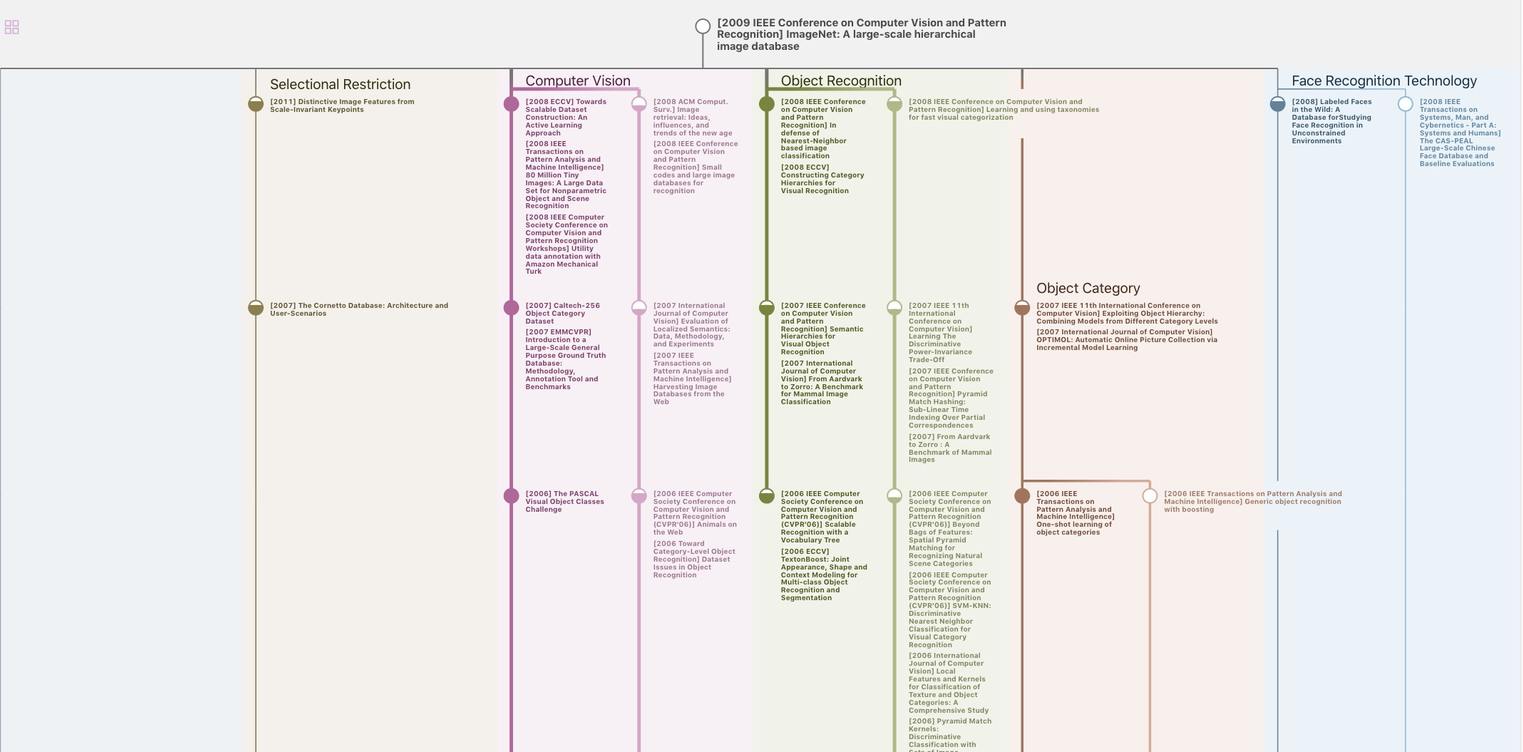
生成溯源树,研究论文发展脉络
Chat Paper
正在生成论文摘要