Reduced proteasome activity in the aging brain results in ribosome stoichiometry loss and aggregation
Molecular Systems Biology(2020)
摘要
Article18 June 2020Open Access Transparent process Reduced proteasome activity in the aging brain results in ribosome stoichiometry loss and aggregation Erika Kelmer Sacramento Erika Kelmer Sacramento orcid.org/0000-0002-3336-982X Leibniz Institute on Aging-Fritz Lipmann Institute (FLI), Jena, Germany Search for more papers by this author Joanna M Kirkpatrick Joanna M Kirkpatrick Leibniz Institute on Aging-Fritz Lipmann Institute (FLI), Jena, Germany Search for more papers by this author Mariateresa Mazzetto Mariateresa Mazzetto Leibniz Institute on Aging-Fritz Lipmann Institute (FLI), Jena, Germany [email protected], Scuola Normale Superiore, Pisa, Italy Search for more papers by this author Mario Baumgart Mario Baumgart Leibniz Institute on Aging-Fritz Lipmann Institute (FLI), Jena, Germany Search for more papers by this author Aleksandar Bartolome Aleksandar Bartolome Leibniz Institute on Aging-Fritz Lipmann Institute (FLI), Jena, Germany Search for more papers by this author Simone Di Sanzo Simone Di Sanzo Leibniz Institute on Aging-Fritz Lipmann Institute (FLI), Jena, Germany Search for more papers by this author Cinzia Caterino Cinzia Caterino Leibniz Institute on Aging-Fritz Lipmann Institute (FLI), Jena, Germany [email protected], Scuola Normale Superiore, Pisa, Italy Search for more papers by this author Michele Sanguanini Michele Sanguanini orcid.org/0000-0002-7142-3807 Centre for Misfolding Diseases, Department of Chemistry, University of Cambridge, Cambridge, UK Search for more papers by this author Nikoletta Papaevgeniou Nikoletta Papaevgeniou Institute of Chemical Biology, National Hellenic Research Foundation, Athens, Greece Search for more papers by this author Maria Lefaki Maria Lefaki Institute of Chemical Biology, National Hellenic Research Foundation, Athens, Greece Search for more papers by this author Dorothee Childs Dorothee Childs European Molecular Biology Laboratory, Heidelberg, Germany Search for more papers by this author Sara Bagnoli Sara Bagnoli [email protected], Scuola Normale Superiore, Pisa, Italy Search for more papers by this author Eva Terzibasi Tozzini Eva Terzibasi Tozzini [email protected], Scuola Normale Superiore, Pisa, Italy Search for more papers by this author Domenico Di Fraia Domenico Di Fraia Leibniz Institute on Aging-Fritz Lipmann Institute (FLI), Jena, Germany Search for more papers by this author Natalie Romanov Natalie Romanov European Molecular Biology Laboratory, Heidelberg, Germany Search for more papers by this author Peter H Sudmant Peter H Sudmant orcid.org/0000-0002-9573-8248 University of California Berkeley, Berkeley, CA, USA Search for more papers by this author Wolfgang Huber Wolfgang Huber European Molecular Biology Laboratory, Heidelberg, Germany Search for more papers by this author Niki Chondrogianni Niki Chondrogianni Institute of Chemical Biology, National Hellenic Research Foundation, Athens, Greece Search for more papers by this author Michele Vendruscolo Michele Vendruscolo orcid.org/0000-0002-3616-1610 Centre for Misfolding Diseases, Department of Chemistry, University of Cambridge, Cambridge, UK Search for more papers by this author Alessandro Cellerino Corresponding Author Alessandro Cellerino [email protected] orcid.org/0000-0003-3834-0097 Leibniz Institute on Aging-Fritz Lipmann Institute (FLI), Jena, Germany [email protected], Scuola Normale Superiore, Pisa, Italy Search for more papers by this author Alessandro Ori Corresponding Author Alessandro Ori [email protected] orcid.org/0000-0002-3046-0871 Leibniz Institute on Aging-Fritz Lipmann Institute (FLI), Jena, Germany Search for more papers by this author Erika Kelmer Sacramento Erika Kelmer Sacramento orcid.org/0000-0002-3336-982X Leibniz Institute on Aging-Fritz Lipmann Institute (FLI), Jena, Germany Search for more papers by this author Joanna M Kirkpatrick Joanna M Kirkpatrick Leibniz Institute on Aging-Fritz Lipmann Institute (FLI), Jena, Germany Search for more papers by this author Mariateresa Mazzetto Mariateresa Mazzetto Leibniz Institute on Aging-Fritz Lipmann Institute (FLI), Jena, Germany [email protected], Scuola Normale Superiore, Pisa, Italy Search for more papers by this author Mario Baumgart Mario Baumgart Leibniz Institute on Aging-Fritz Lipmann Institute (FLI), Jena, Germany Search for more papers by this author Aleksandar Bartolome Aleksandar Bartolome Leibniz Institute on Aging-Fritz Lipmann Institute (FLI), Jena, Germany Search for more papers by this author Simone Di Sanzo Simone Di Sanzo Leibniz Institute on Aging-Fritz Lipmann Institute (FLI), Jena, Germany Search for more papers by this author Cinzia Caterino Cinzia Caterino Leibniz Institute on Aging-Fritz Lipmann Institute (FLI), Jena, Germany [email protected], Scuola Normale Superiore, Pisa, Italy Search for more papers by this author Michele Sanguanini Michele Sanguanini orcid.org/0000-0002-7142-3807 Centre for Misfolding Diseases, Department of Chemistry, University of Cambridge, Cambridge, UK Search for more papers by this author Nikoletta Papaevgeniou Nikoletta Papaevgeniou Institute of Chemical Biology, National Hellenic Research Foundation, Athens, Greece Search for more papers by this author Maria Lefaki Maria Lefaki Institute of Chemical Biology, National Hellenic Research Foundation, Athens, Greece Search for more papers by this author Dorothee Childs Dorothee Childs European Molecular Biology Laboratory, Heidelberg, Germany Search for more papers by this author Sara Bagnoli Sara Bagnoli [email protected], Scuola Normale Superiore, Pisa, Italy Search for more papers by this author Eva Terzibasi Tozzini Eva Terzibasi Tozzini [email protected], Scuola Normale Superiore, Pisa, Italy Search for more papers by this author Domenico Di Fraia Domenico Di Fraia Leibniz Institute on Aging-Fritz Lipmann Institute (FLI), Jena, Germany Search for more papers by this author Natalie Romanov Natalie Romanov European Molecular Biology Laboratory, Heidelberg, Germany Search for more papers by this author Peter H Sudmant Peter H Sudmant orcid.org/0000-0002-9573-8248 University of California Berkeley, Berkeley, CA, USA Search for more papers by this author Wolfgang Huber Wolfgang Huber European Molecular Biology Laboratory, Heidelberg, Germany Search for more papers by this author Niki Chondrogianni Niki Chondrogianni Institute of Chemical Biology, National Hellenic Research Foundation, Athens, Greece Search for more papers by this author Michele Vendruscolo Michele Vendruscolo orcid.org/0000-0002-3616-1610 Centre for Misfolding Diseases, Department of Chemistry, University of Cambridge, Cambridge, UK Search for more papers by this author Alessandro Cellerino Corresponding Author Alessandro Cellerino [email protected] orcid.org/0000-0003-3834-0097 Leibniz Institute on Aging-Fritz Lipmann Institute (FLI), Jena, Germany [email protected], Scuola Normale Superiore, Pisa, Italy Search for more papers by this author Alessandro Ori Corresponding Author Alessandro Ori [email protected] orcid.org/0000-0002-3046-0871 Leibniz Institute on Aging-Fritz Lipmann Institute (FLI), Jena, Germany Search for more papers by this author Author Information Erika Kelmer Sacramento1,‡, Joanna M Kirkpatrick1,7,‡, Mariateresa Mazzetto1,2,‡, Mario Baumgart1, Aleksandar Bartolome1, Simone Di Sanzo1, Cinzia Caterino1,2, Michele Sanguanini3, Nikoletta Papaevgeniou4, Maria Lefaki4, Dorothee Childs5, Sara Bagnoli2, Eva Terzibasi Tozzini2, Domenico Di Fraia1, Natalie Romanov5,8, Peter H Sudmant6, Wolfgang Huber5, Niki Chondrogianni4, Michele Vendruscolo3, Alessandro Cellerino *,1,2 and Alessandro Ori *,1 1Leibniz Institute on Aging-Fritz Lipmann Institute (FLI), Jena, Germany 2[email protected], Scuola Normale Superiore, Pisa, Italy 3Centre for Misfolding Diseases, Department of Chemistry, University of Cambridge, Cambridge, UK 4Institute of Chemical Biology, National Hellenic Research Foundation, Athens, Greece 5European Molecular Biology Laboratory, Heidelberg, Germany 6University of California Berkeley, Berkeley, CA, USA 7Present address: Proteomics Science Technology Platform, The Francis Crick Institute, London, UK 8Present address: Max Planck Institute of Biophysics, Frankfurt am Main, Germany ‡These authors contributed equally to this work *Corresponding author. Tel: +39 0503 152756; E-mail: [email protected] *Corresponding author. Tel: +49 3641 65 6808; E-mail: [email protected] Molecular Systems Biology (2020)16:e9596https://doi.org/10.15252/msb.20209596 [Correction added on 28 September 2020, after first online publication: Projekt Deal funding statement has been added.] PDFDownload PDF of article text and main figures. Peer ReviewDownload a summary of the editorial decision process including editorial decision letters, reviewer comments and author responses to feedback. ToolsAdd to favoritesDownload CitationsTrack CitationsPermissions ShareFacebookTwitterLinked InMendeleyWechatReddit Figures & Info Abstract A progressive loss of protein homeostasis is characteristic of aging and a driver of neurodegeneration. To investigate this process quantitatively, we characterized proteome dynamics during brain aging in the short-lived vertebrate Nothobranchius furzeri combining transcriptomics and proteomics. We detected a progressive reduction in the correlation between protein and mRNA, mainly due to post-transcriptional mechanisms that account for over 40% of the age-regulated proteins. These changes cause a progressive loss of stoichiometry in several protein complexes, including ribosomes, which show impaired assembly/disassembly and are enriched in protein aggregates in old brains. Mechanistically, we show that reduction of proteasome activity is an early event during brain aging and is sufficient to induce proteomic signatures of aging and loss of stoichiometry in vivo. Using longitudinal transcriptomic data, we show that the magnitude of early life decline in proteasome levels is a major risk factor for mortality. Our work defines causative events in the aging process that can be targeted to prevent loss of protein homeostasis and delay the onset of age-related neurodegeneration. Synopsis Analyses of proteome dynamics delineate a timeline of molecular events underlying brain aging in the vertebrate Nothobranchius furzeri. Early-in-life decline of proteasome activity is associated with loss of stoichiometry of protein complexes and predicts lifespan. Progressive loss of stoichiometry affects multiple protein complexes. Ribosomes aggregate in old brains. Partial reduction of proteasome activity is sufficient to induce loss of stoichiometry. Reduced proteasome levels are a major risk factor for early death in killifish. Introduction Although age is the primary risk factor for cognitive decline and dementia (Assoc, 2018), the associated age-dependent molecular changes are still not known in detail. Despite the presence of clear functional impairments (Buckner, 2004), physiological brain aging is characterized by limited loss of neurons (Schmitz & Hof, 2007) and specific morphological changes of synaptic contacts (Dickstein et al, 2013). Large collections of data for transcript dynamics in human and animal brains indicate that systematic, age-dependent changes in gene expression are also relatively minor (Cellerino & Ori, 2017), although some shared transcriptional signatures have been identified, including a chronic activation of cellular inflammatory response (Aramillo Irizar et al, 2018), reactive changes in glial cells (Clarke et al, 2018), and reduced expression of neuronal and synaptic genes (Lu et al, 2004; Somel et al, 2010). Since the vast majority of human neurons are generated during fetal and perinatal life and neuronal turnover is limited in the post-natal human brain (Sorrells et al, 2018), neurons are particularly prone to accumulate misfolded proteins that are not properly processed by the cellular proteolytic mechanisms (proteasomal and autophagic pathways), thus forming aberrant deposits. Indeed, neurodegenerative diseases are characterized by the prominent presence of protein aggregates, in particular due to mutations that facilitate misfolding and aggregation, and impairment of cellular quality control systems (Soto & Pritzkow, 2018). Accumulation of protein aggregates occurs also during physiological aging, as demonstrated by the presence of lipofuscin (Glees & Hasan, 1976) and ubiquitinated cellular inclusions (Zeier et al, 2011; Matsui et al, 2019). However, the exact composition of these spontaneous aggregates and the mechanisms triggering their formation during brain aging remain unknown. Although age-dependent transcript changes in the brain have been studied extensively (Blalock et al, 2003; Lu et al, 2004; Loerch et al, 2008; Colantuoni et al, 2011; Wood et al, 2013), we are just beginning to understand the corresponding global regulation of the proteome during aging (Somel et al, 2010; Ori et al, 2015; Walther et al, 2015). Substantial post-transcriptional regulation takes place in the aging brain, with a sizeable proportion of proteins being up- or down-regulated in the absence of changes in the levels of the corresponding transcripts (Ori et al, 2015), resulting a progressive mRNA-protein decoupling (Janssens et al, 2015; Wei et al, 2015). Protein aggregation could play a role in generating an imbalance between protein and transcript levels, but these aspects have not yet been investigated systematically in vertebrate brains. To address this challenge, we studied the annual killifish Nothobranchius furzeri, which is the shortest-lived vertebrate that can currently be bred in captivity. With a median lifespan of 3–7 months (Valdesalici & Cellerino, 2003; Terzibasi et al, 2008; Ripa et al, 2017; Hu & Brunet, 2018), it has emerged as a convenient model organism to investigate genetic and non-genetic interventions on aging (Harel et al, 2015; Cellerino et al, 2016; Kim et al, 2016; Platzer & Englert, 2016; Ripa et al, 2017), since it replicates many typical aspects of vertebrate brain aging at the levels of behavior (Valenzano et al, 2006a,b), neuroanatomy (Tozzini et al, 2012), and global gene expression (Baumgart et al, 2014; Aramillo Irizar et al, 2018). Age-dependent processes are enhanced in this species, thus facilitating the detection of differentially expressed genes as compared to other model organisms (Wood et al, 2013; Baumgart et al, 2014; Frahm et al, 2017). Importantly, an age-dependent formation of inclusion bodies containing α-synuclein and spontaneous degeneration of dopaminergic neurons has been recently described in killifish (Matsui et al, 2019). This phenotype closely mimics human pathologies and make killifish an extremely attractive vertebrate system to study age-related neurodegenerative disorders and therapeutic strategy against them. In this work, we applied RNA-seq, mass spectrometry-based proteomics, and analysis of protein aggregates in killifish of different ages to delineate a timeline of molecular events responsible for loss of proteome homeostasis during brain aging. In particular, we set to identify the nature and biophysical properties of proteins that preferentially aggregate in old brains, to comprehensively investigate the loss of stoichiometry of protein complexes and their assembly state, and the role played by the proteasome as an early driver of protein homeostasis collapse using in vivo pharmacological experiments. Finally, we tested whether interindividual differences in proteasome decline influence mortality. Results Transcript and protein levels become progressively decoupled during brain aging We initially analyzed whole brains from animals of three different age groups by liquid chromatography–tandem mass spectrometry using a label-free method. Based on previous phenotypic data, we chose to compare young, sexually mature fish (5 weeks post-hatching, wph), adult fish (12 wph) that do not show aging phenotypes (Terzibasi et al, 2008), and old fish (39 wph) that display neurodegeneration (Di Cicco et al, 2011; Tozzini et al, 2012) (Fig 1A and Dataset EV1). Principal component analysis separated samples according to the age groups (Fig 1B). In order to achieve higher proteome coverage, we split the age groups into two separate experiments based on tandem mass tag (TMT) multiplexing, where we compared adult vs young fish and old vs adult fish (Fig EV1A). This was necessary because of the limited number of channels available (10 per experiment) and to do not reduce the number of animals analyzed per age group. A total of 8,885 protein groups were quantified with at least two proteotypic peptides, of which 7,200 were quantified in both experiments (Fig EV1B and Table EV1). Almost half of the quantified protein groups (4,179/8,885) was significantly affected by aging in at least one of the age comparisons (Dataset EV2). Functionally related proteins showed different patterns of abundance change between age groups, and pathways affected by aging in other species, including inflammation-related pathways (Aramillo Irizar et al, 2018), the complement, and coagulation cascade (Clarke et al, 2018), were affected in killifish already in the transition from young to adult (Fig EV1C and Dataset EV2). Figure 1. Transcript and protein levels become decoupled during Nothobranchius furzeri brain aging A. Survival curve of N. furzeri in the FLI facility. Recording of deaths starts at age of 5 wph, which corresponds to sexual maturity, and the colored dashed lines indicate the three age groups analyzed in this study (five animals/group), namely 5 weeks post-hatching (wph, young, sexual maturity), 12 wph (adult), and 39 wph (old, past median lifespan) of a wild-derived strain that exhibits a median lifespan of 7–8 months. B. Principal component analysis (PCA) of brain samples based on the abundance of all proteins identified by label-free mass spectrometry. The smaller dots represent individual samples and the larger dots the centroids of each age-matched group. Ellipses represent 95% confidence intervals. The percentage of variance explained by the first two PC axes is reported in the axis titles. C. Global protein–transcript correlation for each sample, grouped by age. RPKM and iBAQ values were used to estimate transcript and protein levels from matched RNA-seq and TMT-based proteomics data obtained from the same animal. An ANOVA test was performed to evaluate significance among the age groups (mean correlation at 5 wph: 0.48; at 12 wph: 0.43; and at 39 wph: 0.33; P = 3.05e−07, n = 5 per age group). In boxplots, the horizontal line represents the median, the bottom, and top of the box the 25th and 75th percentile, respectively, and the whiskers extend 1.5-fold the interquartile range. D, E. Scatter plot of log2 fold changes for genes differentially expressed both at transcript and protein levels (adj. P < 0.05). The color gradients indicate gene density in the regions where individual points overlap. Numbers of genes in each quadrant and the value of Pearson's coefficient of correlation, r, are reported for each graph. Solid lines represent a spline fit (r = 0.505 for genes significantly affected at both transcript and protein levels, P < 2.2 × 10−16, D; r = 0.126, P = 0.007, E). F. Mechanisms affecting protein abundance during aging. Barplots are based on all the proteins affected in either one of the age comparisons (adj. P < 0.05). Proteins were divided into following five groups: (i) proteins and transcripts with significant and consistent changes (dark brown), (ii) proteins with significant changes, and with consistent changes of the transcripts (light brown), (iii) proteins with no transcripts detected (dark gray), (iv) proteins with transcripts whose translation is potentially regulated by miRNAs (light gray), as assessed by the workflow displayed in Fig EV2D, and (v) all the remaining proteins that we classified as regulated by other post-transcriptional mechanisms (violet). pgs, protein groups. G, H. Barplots representing enriched KEGG pathways among genes that showed significant changes at both transcript and protein levels in aging. Genes were grouped according to the four possible patterns of transcript and protein regulation, as visualized by their positions in the four quadrants shown in (D) and (E), respectively. Only pathways significantly enriched (FDR < 0.05) are shown. The complete list of enriched pathways is reported in Dataset EV4. Data information; Related to Figs EV1 and EV2, Table EV1, and Dataset EV1–EV4. Download figure Download PowerPoint Click here to expand this figure. Figure EV1. Proteome analysis of killifish brain aging and comparison of transcriptome dataset Principal component analysis (PCA) of brain samples based on proteome profiles obtained by tandem mass tag (TMT) quantification for the 12 vs 5 wph and 39 vs 12 wph comparisons. For each sample group, the four samples displaying the highest within group correlation were selected and used for differential expression analysis. The smaller dots represent individual samples and the larger dots the centroids of each age-matched group. Ellipses represent 95% confidence intervals. The percentage of variance explained by the first two PC axes is reported in the axis titles. Overlap between the quantified protein groups across the two TMT experiments. Only proteins quantified with at least two unique (proteotypic) peptides were considered. Barplots representing enriched KEGG pathways among proteins affected by aging in killifish brain. Pathway enrichment was performed using gage (Luo et al, 2009). Significant pathways enriched among up-regulated (red) or down-regulated proteins (blue) are shown (P < 0.05). The complete list of enriched pathways is reported in Dataset EV2. Principal component analysis (PCA) of brain samples based on total RNA (“this study”, same sample used for proteome analysis) or polyA+ RNA sequencing (Baumgart et al, 2014)). The smaller dots represent individual samples and the larger dots the centroids of each age-matched group. Ellipses represent 95% confidence intervals. The percentage of variance explained by the first two PC axes is reported in the axis titles. Correlation between transcript and protein during aging. RPKM and iBAQ values were used to estimate transcript and protein levels from RNA-seq (Baumgart et al, 2014) and TMT-based proteomics data (this study). Since in this case samples were not matched, proteomic data from each individual sample were compared against combined RPKM values obtained from the average of the samples for each group. The ANOVA test was performed to evaluate significance among the age groups (mean correlation at 5 wph: 0.43; at 12 wph: 0.38; and at 39 wph: 0.32; P = 2.02e−12, n = 5 per age group). In boxplots, the horizontal line represents the median, the bottom, and top of the box the 25th and 75th percentile, respectively, and the whiskers extend 1.5-fold the interquartile range. Comparison between RNA-seq data obtained in this work and previous data obtained from (Baumgart et al, 2014). Fold changes (log2) were compared and plotted for both the aging comparisons (12 wph vs 5 wph and 39 wph vs 12 wph) for significantly affected transcripts in both comparisons (adj. P < 0.01). Statistics of differentially expressed genes in the two RNA-seq datasets. The same datasets used for G were analyzed. Differentially expressed genes obtained for three different age comparisons were compared between the datasets. Dark gray boxes correspond to not-affected transcripts, while yellow (adj. P < 0.01) and orange (adj. P < 0.01 and absolute log2 fold change > 0.5) boxes to differentially expressed genes. The complete list of quantified transcripts is reported in Dataset EV3. Download figure Download PowerPoint Total RNA-seq after rRNA depletion and microRNA-seq were obtained from the same samples (Fig EV1D, Table EV1 and Dataset EV3). For each sample, absolute protein abundances estimated from peptide intensities (iBAQ values, (Schwanhäusser et al, 2011)) were correlated with the corresponding transcript levels obtained by RNA-seq (RPKM values), obtaining global protein–transcript correlation values for each sample separately. We observed a progressive age-dependent reduction of protein–transcript correlation values (Fig 1C), consistent with a decoupling between RNA transcripts and proteins during brain aging (Wei et al, 2015). Decoupling was observed also when analyzing an independent RNA-seq dataset from polyA+ RNA for animals of the same age groups (Baumgart et al, 2014) (Fig EV1E). Fold changes of genes differentially expressed in the two RNA-seq datasets were strongly correlated (Fig EV1F). For further analysis, we then focused on the dataset with higher sequencing depth and larger number of replicates, for which the absolute number of differentially expressed genes was higher (polyA+ RNA dataset, Fig EV1G). Direct comparison of protein and mRNA fold changes across age groups revealed discrepancies between RNA and protein regulation (Fig 1D and E and Dataset EV4). Protein and transcript changes were significantly correlated in the adult vs young fish comparison (Fig 1D), but the correlation was reduced in the old vs adult comparison (Fig 1E), further supporting a progressive decoupling between transcript and protein regulation. For validation, we analyzed proteins previously identified to be very long-lived in rodent brain (Toyama et al, 2013), including histones, collagens, and myelin proteins. For these proteins, we found that transcript, but not protein levels, were generally decreased in old fish, indicating that protein stability might contribute to the observed discrepancies between transcripts and proteins (Fig EV2A). In contrast, protein levels estimated by mass spectrometry and immunoreactivity for the glial fibrillary acidic protein (GFAP) were shown to increase significantly in the aging brain (Tozzini et al, 2012), while RNA levels remained unchanged (Fig EV2B). To exclude biases deriving from changes in cellular composition of the brain with aging, we analyzed the regulation of established cell markers (Sharma et al, 2015) in the age group comparisons. We found only minor changes that were consistent at the protein and transcript level (Fig EV2C), thus excluding that the observed decoupling between transcript and protein levels is due to changes in cellular composition. Click here to expand this figure. Figure EV2. Validation of discordant transcript and protein changes during aging Analysis of age-related changes of abundance for long-lived proteins. Average fold changes (log2) are displayed as bars for transcripts (light blue) and proteins (dark blue), for a subset of extremely long-lived proteins identified by (Toyama et al, 2013). Validation of an increased level of glial fibrillary acidic protein (GFAP) in old killifish brain that manifests exclusively at the protein level. Double immunostaining for S100 (green) and GFAP (red) in the central region of the optic tectum of 7 wph fish (upper panels) vs 30 wph fish (lower panels). Scale bar = 100 μm. Average transcript and protein fold changes (log2) are displayed as bars for transcript (light blue) and protein (dark blue) levels of GFAP. Age-related changes of markers for specific cell types in the brain of Nothobranchius furzeri. Fold changes for different age pairwise comparisons (12 vs 5 wph in white; 39 vs 5 wph in blue) were plotted using the cell-type markers from (Sharma et al, 2015) (in particular neuronal, oligodendrocytes, microglia, and astrocytes markers). For each cell type, the top 100 marker genes (ranked according to adj. P value) were plotted. Both transcriptome and proteome fold changes are displayed. In boxplots, the horizontal line represents the median, the bottom, and top of the box the 25th and 75th percentile, respectively, and the whiskers extend 1.5-fold the interquartile range. Workflow for the identification of proteins potentially affected by miRNA regulation during aging. For this analysis, proteins were divided into two groups: (i) proteins affected by aging (adj. P < 0.05) whose abundance change could be explained by transcript expression change and (ii) proteins affected by aging whose transcript was either regulated but with opposite fold change (discordant cases) or not regulated at all. miRNA analysis was performed only on the latter. To obtain a list of miRNAs affected by aging, differential expression was performed on both the age comparisons (12 vs 5 wph and 39 vs 12 wph) separately. The resulting list of miRNAs affected by aging is available in Dataset EV3. Download figure Download PowerPoint To find out whether microRNAs could contribute to transcript-protein decoupling, we first analyzed miRNA expression levels across the same three age groups (Dataset EV3) and then mapped the targets of age-affected miRNAs to our proteome data (Fig EV2D). By considering potential regulation mediated by miRNAs, we defined a subset of proteins whose abundance is affected by aging via mechanisms independent of both transcript level and miRNA-mediated post-transcriptional regulation (Fig 1F). This subset accounted for 30% of the affected proteins in the adult vs young fish comparison and increased up to 43% in the old vs adult comparison. To clarify whether the transcript-protein decoupling preferentially affects some specific pathways, we classified age-affected genes according to their respective transcript and protein fold changes, and performed pathway overrepresentation analysis (Dataset EV4). In the comparison adult vs young fish, pathways related to the complement coagulation cascade and synaptic function/plasticity were overrepresented in concordantly increased transcripts and
更多查看译文
关键词
ribosome stoichiometry loss,proteasome activity,aging
AI 理解论文
溯源树
样例
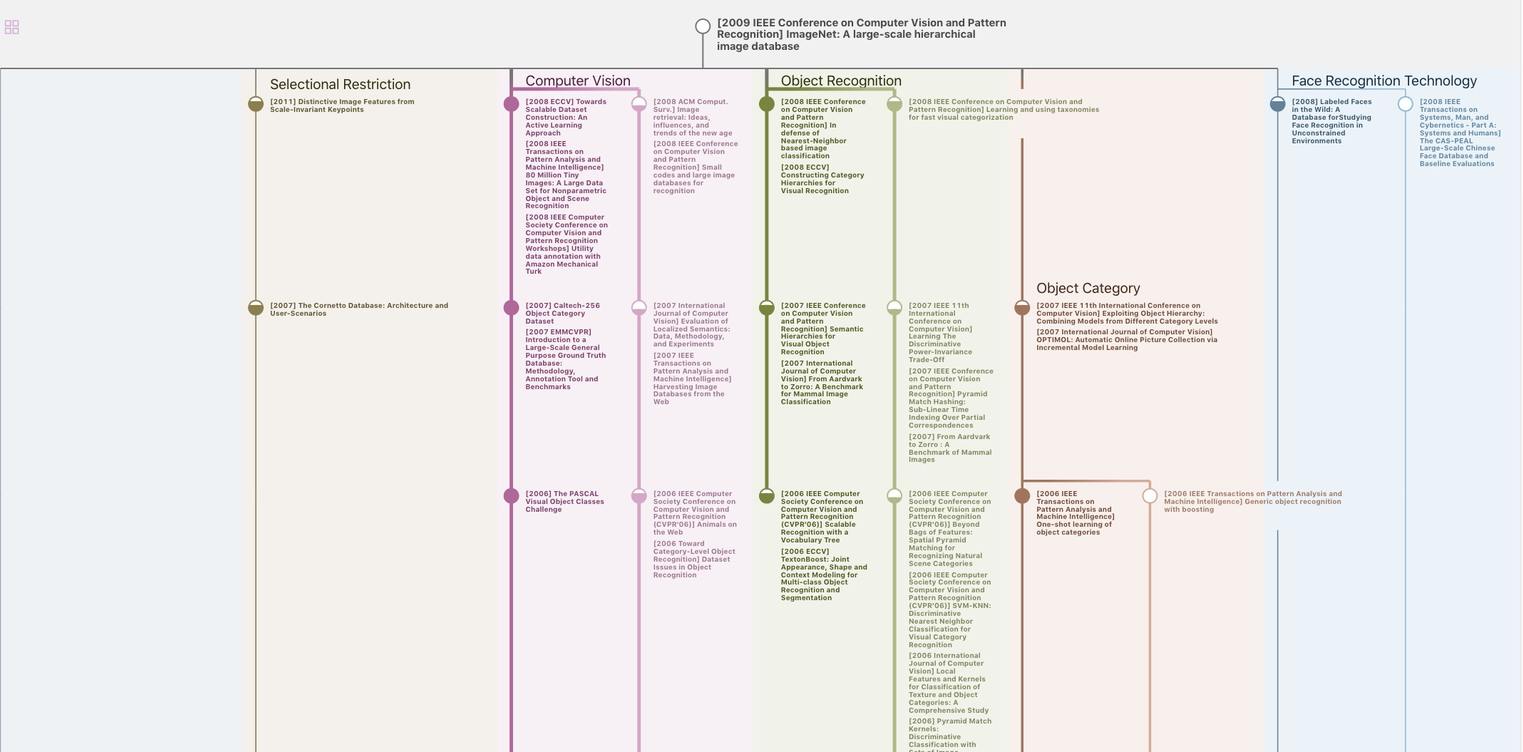
生成溯源树,研究论文发展脉络
Chat Paper
正在生成论文摘要