Pedestrian safety in an automated driving environment: Calibrating and evaluating the responsibility-sensitive safety model
ACCIDENT ANALYSIS AND PREVENTION(2023)
摘要
The severity of vehicle-pedestrian crashes has prompted authorities worldwide to concentrate on improving pedestrian safety. The situation has only become more urgent with the approach of automated driving scenarios. The Responsibility-Sensitive Safety (RSS) model, introduced by Mobileye & REG;, is a rigorous mathematical model developed to facilitate the safe operation of automated vehicles. The RSS model has been calibrated for several vehicle conflict scenarios; however, it has not yet been tested for pedestrian safety. Therefore, this study calibrates and evaluates the RSS model for pedestrian safety using data from the Shanghai Naturalistic Driving Study. Nearly 400 vehicle-pedestrian conflicts were extracted from 8,000 trips by the threshold and manual check method, and then divided into 16 basic scenarios in three categories. Because crossing conflicts were the most serious and frequent, they were reproduced in MATLAB's Simulink with each vehicle replaced with a virtual automated vehicle loaded with the RSS controller module. With the objectives of maximizing safety and minimizing conservativeness, the non-dominated sorting genetic algorithm II was applied to calibrate the RSS model for vehicle-pedestrian conflicts. The safety performance of the RSS model was then compared with that of the commonly used active safety function, autonomous emergency braking (AEB), and with human driving. Findings verified that the RSS model was safer in vehicle-pedestrian conflicts than both the AEB model and human driving. Its performance also yielded the best test results in producing smooth and stable driving. This study provides a reliable reference for the safe control of automated vehicles with respect to pedestrians.
更多查看译文
关键词
Naturalistic driving data,Vehicle-pedestrian conflict,Responsibility-sensitive safety,Model calibration
AI 理解论文
溯源树
样例
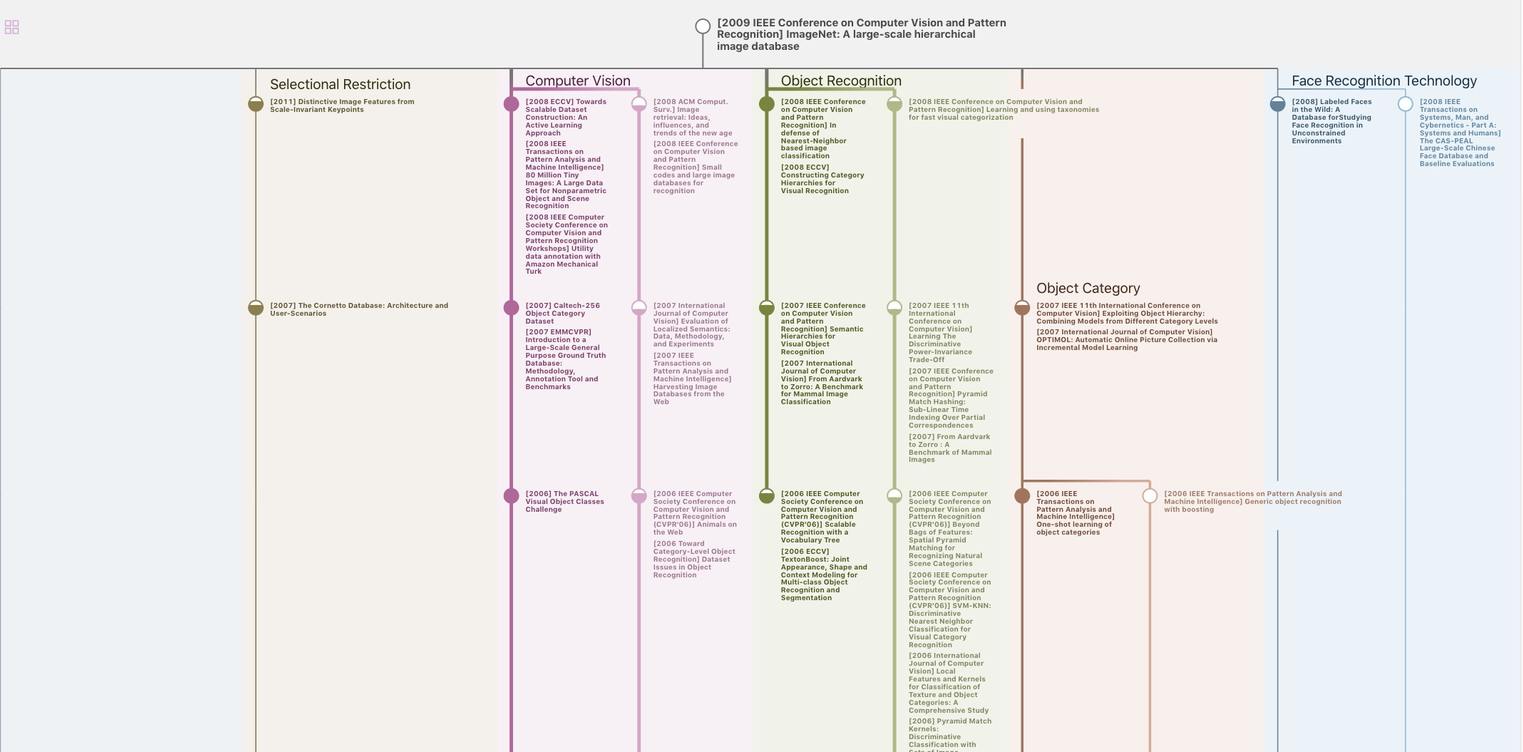
生成溯源树,研究论文发展脉络
Chat Paper
正在生成论文摘要