Active learning based on similarity level histogram and adaptive-scale sampling for very high resolution image classification
Neural Networks(2023)
摘要
In remote sensing image classification, active learning aims to obtain an excellent classification model by selecting informative or representative training samples. However, due to the complexity of remote sensing images, the same class of ground objects usually have different spectral representations. The existing active learning methods may not take into account diverse representations of the same targets, which leads to a possible lack of intra-class diversity in the collected samples. To alleviate this problem, we propose an active learning method based on similarity level histogram (SLH) and adaptive-scale sampling to improve very high resolution remote sensing image classification. Specifically, we construct a SLH for each class of ground objects to effectively consider the intra-class diversity of the same target. To avoid the problem of sample imbalance caused by over-sampling or under-sampling, we design an adaptive-scale sampling strategy. Then, we utilize active learning to mine representative samples from each SLH warehouse according to adaptive-scale sampling strategies until the iteration condition is satisfied. Experiments show that the proposed algorithm can achieve better classification performance with limited training samples and is competitive with other methods based on four sets of publicly available data.
更多查看译文
关键词
Remote sensing,Classification,Active learning,Intra-class diversity
AI 理解论文
溯源树
样例
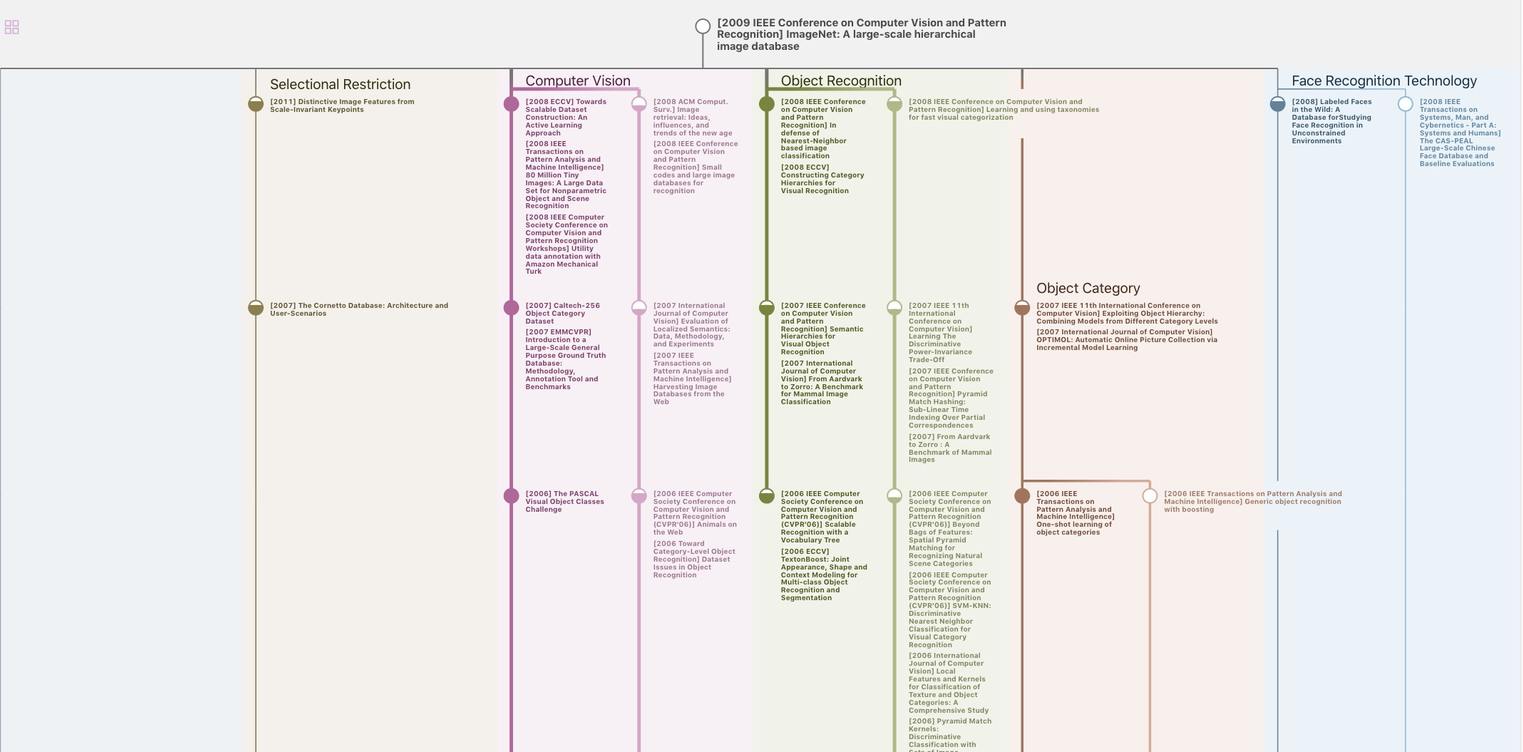
生成溯源树,研究论文发展脉络
Chat Paper
正在生成论文摘要