Correlation analysis of modern analytical data - a chemometric dissection of spectral and chromatographic variables
ANALYTICAL METHODS(2023)
摘要
The Standard Practices for Infrared Multivariate Quantitative Analysis (ASTM E1655) provide a guide for determining physicochemical properties of materials using multivariate calibration techniques applied to chemical sources that have high multicollinearity and correlated information. Partial least squares (PLS) is the most widely used multivariate regression method due to its excellent prediction capabilities and easy optimization. Initially applied to chromatographic data, PLS has also shown great results in near-infrared (NIR) and mid-infrared (MIR) spectroscopies. However, complex chemical matrices with low correlation may not be efficiently modeled using PLS or other multivariate analyses limited by grouping similar information (such as latent variables or principal components). Therefore, this study aims to evaluate the multicollinearity of different analytical techniques, such as high-temperature gas chromatography (HTGC), NIR, MIR, hydrogen nuclear magnetic resonance (H-1 NMR), carbon-13 nuclear magnetic resonance (C-13 NMR), and Fourier transform ion cyclotron resonance mass spectrometry coupled to the electrospray source in positive and negative ionization modes (ESI(& PLUSMN;)FT-ICR). Descriptive statistics (coefficient of determination, R-2) and principal component analysis (PCA) were used to identify the distribution of correlated information. Results showed that NIR and MIR spectroscopies exhibited a higher percentage of correlated variables, while C-13 NMR and ESI(& PLUSMN;)FT-ICR MS had more discrete profiles. Therefore, PLS development may be more effectively applied to NIR, MIR, and H-1 NMR data, while C-13 NMR and mass spectra may require other algorithms or variable selection methods in combination with PLS.
更多查看译文
关键词
modern analytical data,chemometric dissection,correlation,spectral
AI 理解论文
溯源树
样例
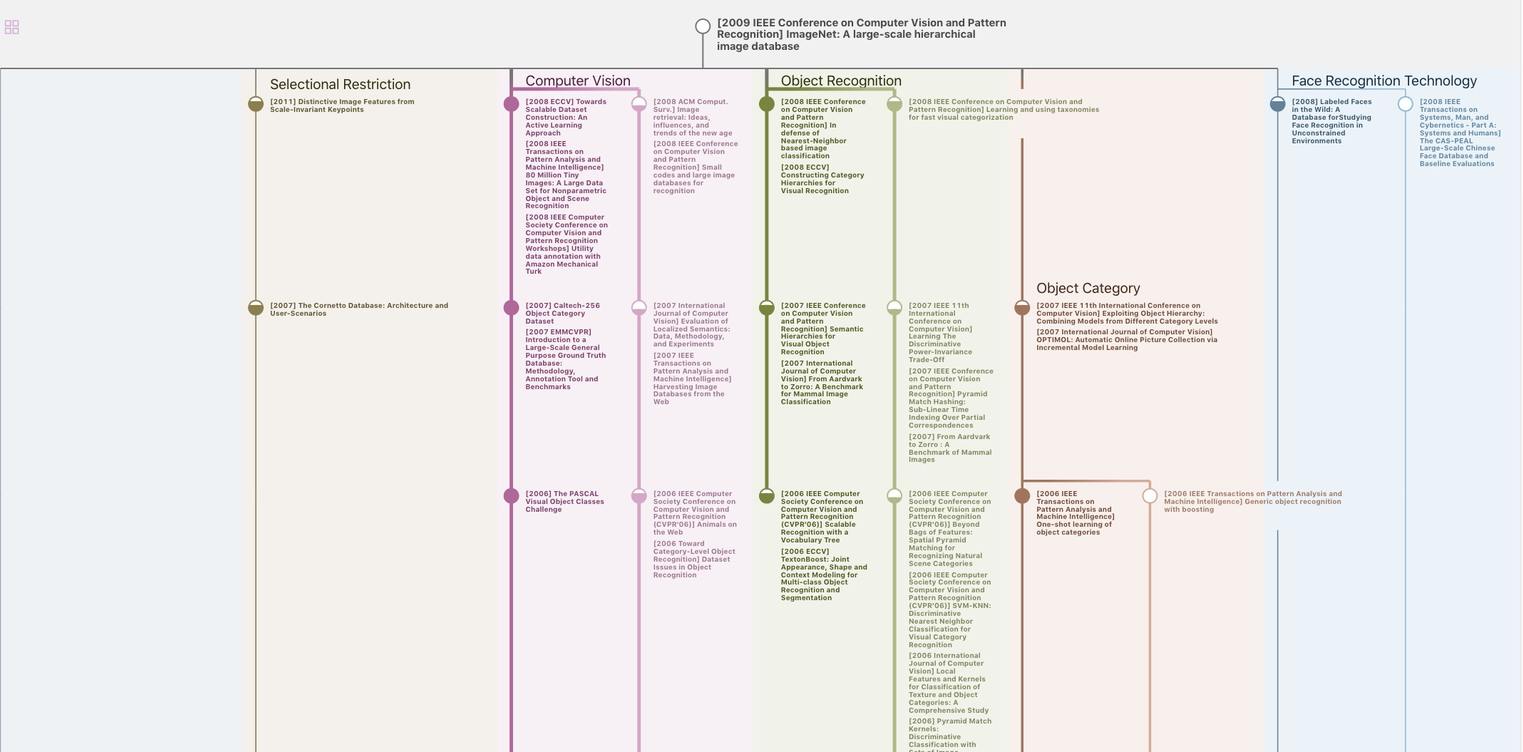
生成溯源树,研究论文发展脉络
Chat Paper
正在生成论文摘要