Short-term forecasts to inform the response to the Covid-19 epidemic in the UK
medRxiv (Cold Spring Harbor Laboratory)(2020)
摘要
Abstract Background Short-term forecasts of infectious disease can aid situational awareness and planning for outbreak response. Here, we report on multi-model forecasts of Covid-19 in the UK that were generated at regular intervals starting at the end of March 2020, in order to monitor expected healthcare utilisation and population impacts in real time. Methods We evaluated the performance of individual model forecasts generated between 24 March and 14 July 2020, using a variety of metrics including the weighted interval score as well as metrics that assess the calibration, sharpness, bias and absolute error of forecasts separately. We further combined the predictions from individual models into ensemble forecasts using a simple mean as well as a quantile regression average that aimed to maximise performance. We compared model performance to a null model of no change. Results In most cases, individual models performed better than the null model, and ensembles models were well calibrated and performed comparatively to the best individual models. The quantile regression average did not noticeably outperform the mean ensemble. Conclusions Ensembles of multi-model forecasts can inform the policy response to the Covid-19 pandemic by assessing future resource needs and expected population impact of morbidity and mortality.
更多查看译文
关键词
forecasts,epidemic,short-term
AI 理解论文
溯源树
样例
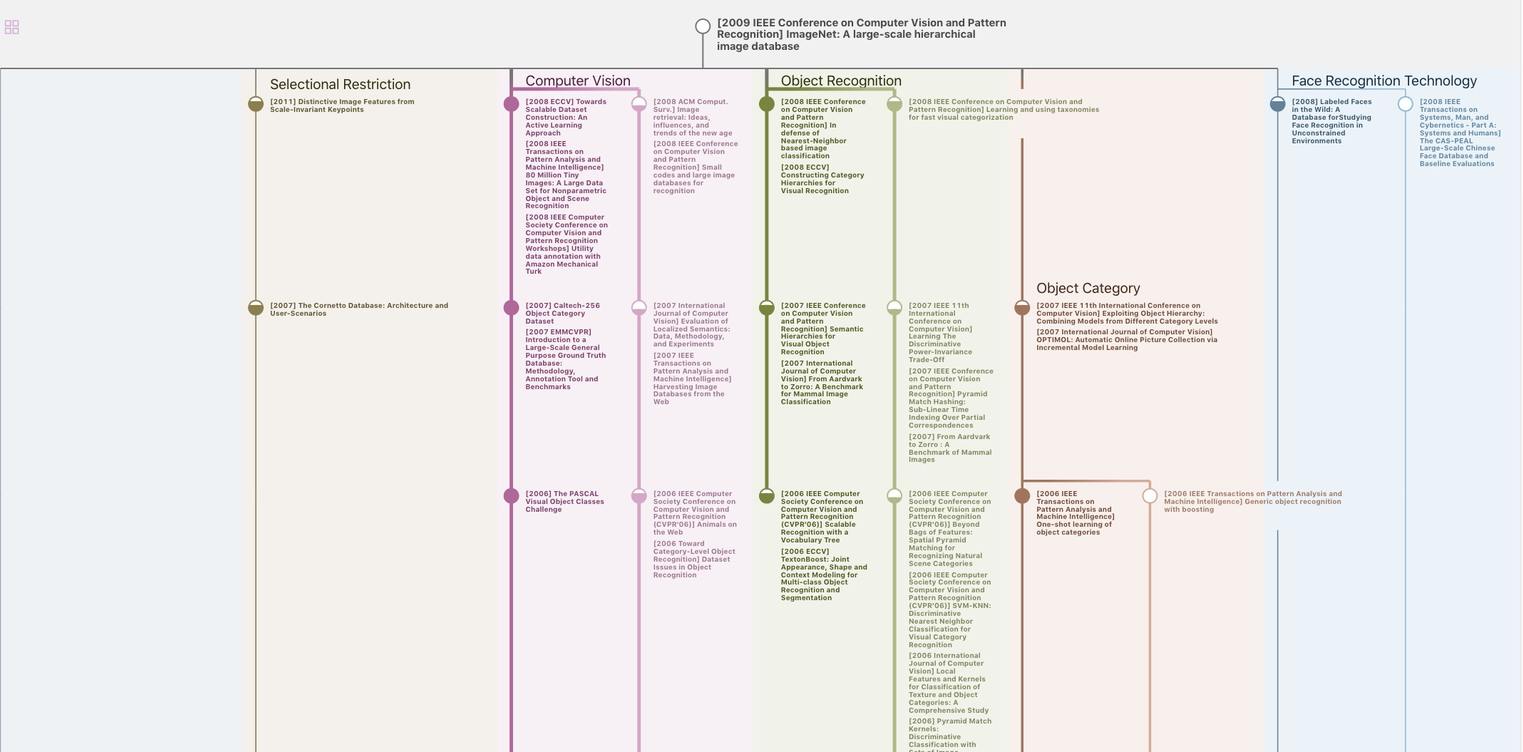
生成溯源树,研究论文发展脉络
Chat Paper
正在生成论文摘要