On Attacking Out-Domain Uncertainty Estimation in Deep Neural Networks
Proceedings of the Thirty-First International Joint Conference on Artificial Intelligence(2022)
摘要
In many applications with real-world consequences, it is crucial to develop reliable uncertainty estimation for the predictions made by the AI decision systems. Targeting at the goal of estimating uncertainty, various deep neural network (DNN) based uncertainty estimation algorithms have been proposed. However, the robustness of the uncertainty returned by these algorithms has not been systematically explored. In this work, to raise the awareness of the research community on robust uncertainty estimation, we show that state-of-the-art uncertainty estimation algorithms could fail catastrophically under our proposed adversarial attack despite their impressive performance on uncertainty estimation. In particular, we aim at attacking out-domain uncertainty estimation: under our attack, the uncertainty model would be fooled to make high-confident predictions for the out-domain data, which they originally would have rejected. Extensive experimental results on various benchmark image datasets show that the uncertainty estimated by state-of-the-art methods could be easily corrupted by our attack.
更多查看译文
关键词
deep neural networks,uncertainty,neural networks,estimation,out-domain
AI 理解论文
溯源树
样例
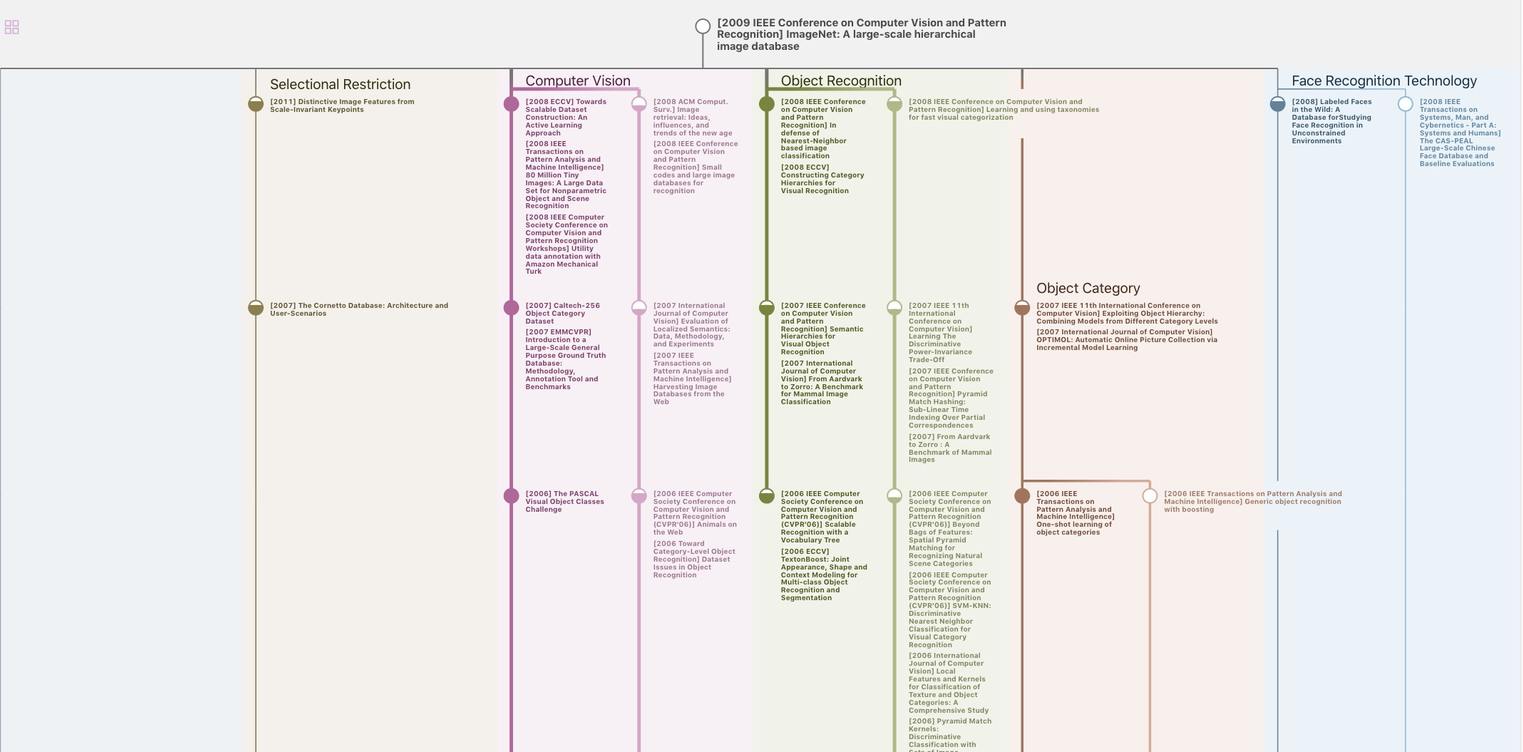
生成溯源树,研究论文发展脉络
Chat Paper
正在生成论文摘要