Critique of: “A Parallel Framework for Constraint-Based Bayesian Network Learning via Markov Blanket Discovery” by SCC Team From UC San Diego
IEEE Transactions on Parallel and Distributed Systems(2023)
Abstract
Bayesian networks (BNs) have become popular in recent years to describe natural phenomena in situations where causal linkages are important to understand. In order to get around the inherent non-tractability of learning BNs, Srivastava et al. propose a markov blanket discovery-based approach to learning in their paper titled “A Parallel Framework for Constraint-based Bayesian Network Learning via Markov Blanket Discovery.” We are able to reproduce both the strong and weak scaling experiments from the paper up to 128 cores, and verify communication cost scaling for all three algorithms in the paper. We also introduce methodological improvements to weak scaling that show the paper's findings are unique to the methodology and not the datasets used. Slight variations in performance were observed due to differences in datasets, core count, and job scheduling.
MoreTranslated text
Key words
bayesian network learning,markov blanket discovery”,constraint-based
AI Read Science
Must-Reading Tree
Example
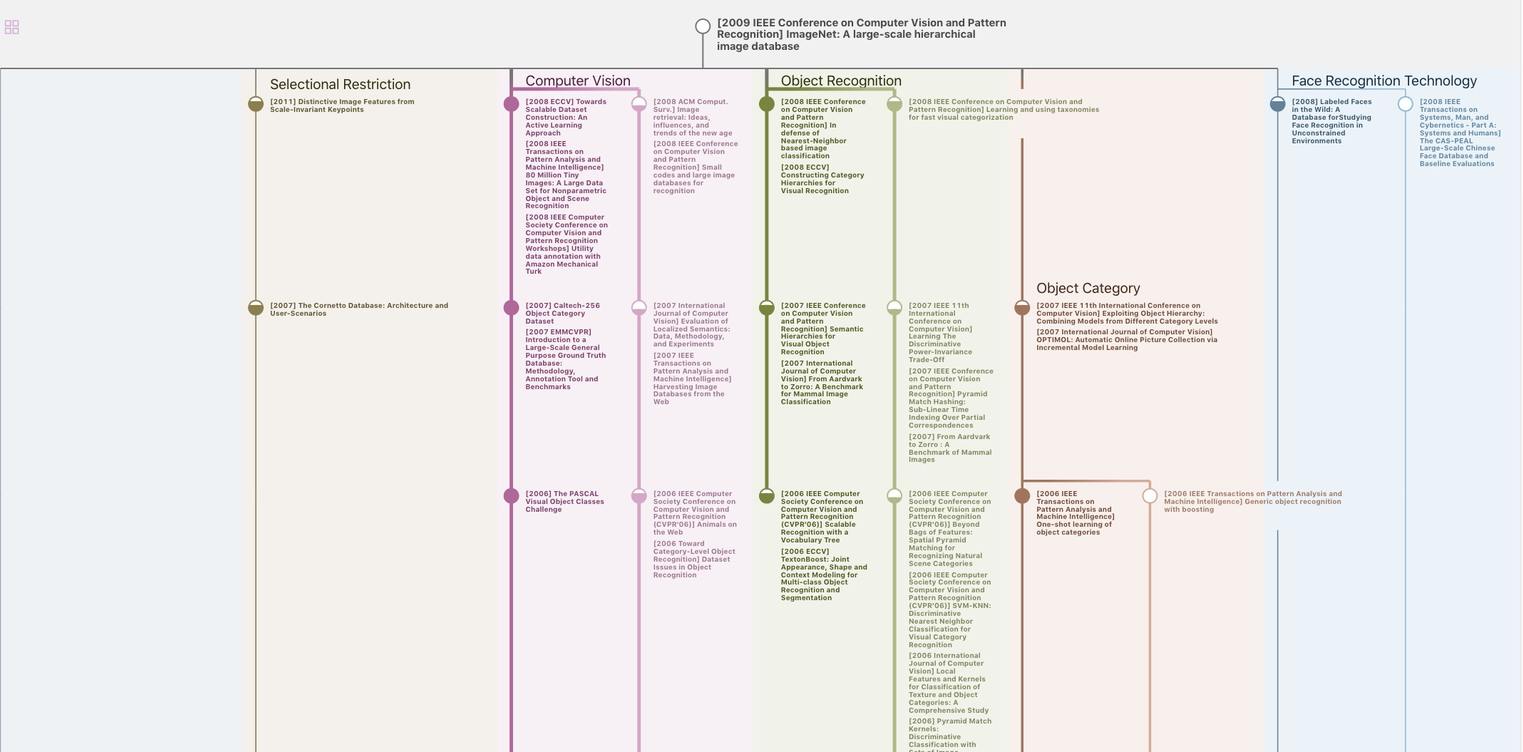
Generate MRT to find the research sequence of this paper
Chat Paper
Summary is being generated by the instructions you defined