Distorted image classification using neural activation pattern matching loss.
Neural networks : the official journal of the International Neural Network Society(2023)
摘要
In image classification, a deep neural network (DNN) that is trained on undistorted images constitutes an effective decision boundary. Unfortunately, this boundary does not support distorted images, such as noisy or blurry ones, leading to accuracy drop-off. As a simple approach for classifying distorted images as well as undistorted ones, previous methods have optimized the trained DNN again on both kinds of images. However, in these methods, the decision boundary may become overly complicated during optimization because there is no regularization of the decision boundary. Consequently, this decision boundary limits efficient optimization. In this paper, we study a simple yet effective decision boundary for distorted image classification through the use of a novel loss, called a "neural activation pattern matching (NAPM) loss". The NAPM loss is based on recent findings that the decision boundary is a piecewise linear function, where each linear segment is constructed from a neural activation pattern in the DNN when an image is fed to it. The NAPM loss extracts the neural activation patterns when the distorted image and its undistorted version are fed to the DNN and then matches them with each other via the sigmoid cross-entropy. Therefore, it constrains the DNN to classify the distorted image and its undistorted version by the same linear segment. As a result, our loss accelerates efficient optimization by preventing the decision boundary from becoming overly complicated. Our experiments demonstrate that our loss increases the accuracy of the previous methods in all conditions evaluated.
更多查看译文
关键词
Deep neural network,Distorted image classification,Neural activation pattern matching loss
AI 理解论文
溯源树
样例
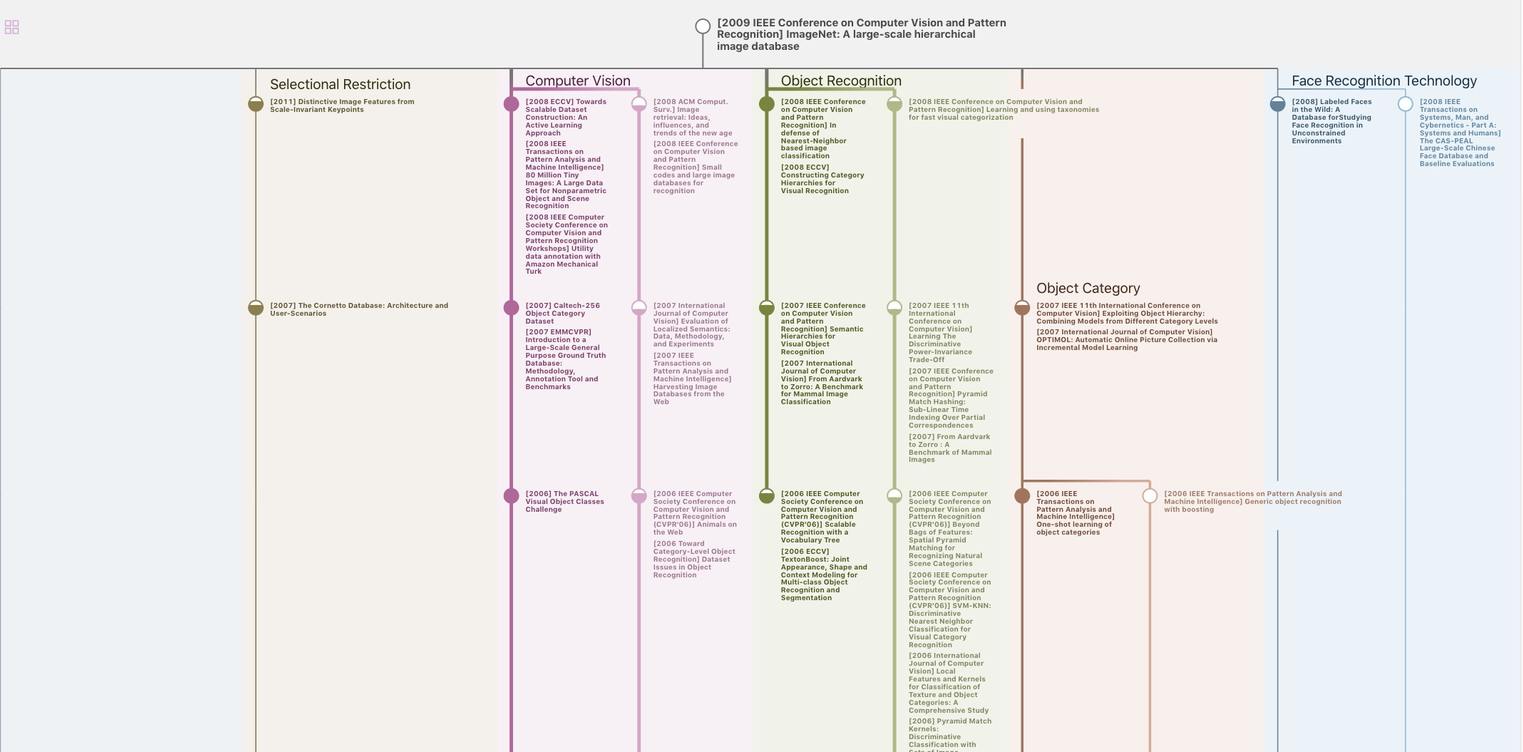
生成溯源树,研究论文发展脉络
Chat Paper
正在生成论文摘要