Task-Decoupled Knowledge Transfer for Cross-Modality Object Detection.
Entropy(2023)
摘要
In harsh weather conditions, the infrared modality can supplement or even replace the visible modality. However, the lack of a large-scale dataset for infrared features hinders the generation of a robust pre-training model. Most existing infrared object-detection algorithms rely on pre-training models from the visible modality, which can accelerate network convergence but also limit performance due to modality differences. In order to provide more reliable feature representation for cross-modality object detection and enhance its performance, this paper investigates the impact of various task-relevant features on cross-modality object detection and proposes a knowledge transfer algorithm based on classification and localization decoupling analysis. A task-decoupled pre-training method is introduced to adjust the attributes of various tasks learned by the pre-training model. For the training phase, a task-relevant hyperparameter evolution method is proposed to increase the network's adaptability to attribute changes in pre-training weights. Our proposed method improves the accuracy of multiple modalities in multiple datasets, with experimental results on the FLIR ADAS dataset reaching a state-of-the-art level and surpassing most multi-spectral object-detection methods.
更多查看译文
关键词
cross-modality,knowledge transfer,task-decoupled pre-training,task-relevant hyperparameter evolution
AI 理解论文
溯源树
样例
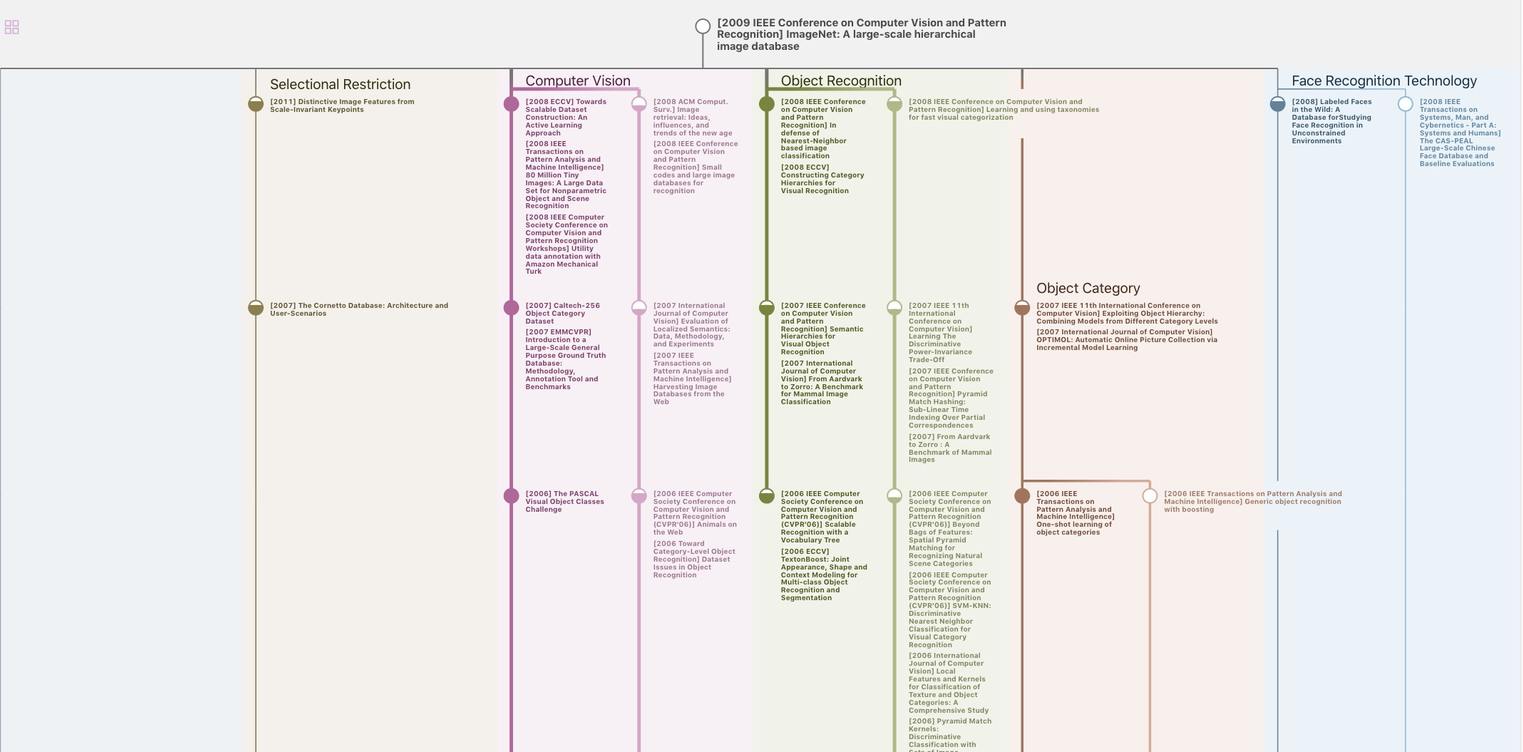
生成溯源树,研究论文发展脉络
Chat Paper
正在生成论文摘要