Students' Learning Behaviour in Programming Education Analysis: Insights from Entropy and Community Detection
Entropy (Basel, Switzerland)(2023)
摘要
The high dropout rates in programming courses emphasise the need for monitoring and understanding student engagement, enabling early interventions. This activity can be supported by insights into students' learning behaviours and their relationship with academic performance, derived from student learning log data in learning management systems. However, the high dimensionality of such data, along with their numerous features, pose challenges to their analysis and interpretability. In this study, we introduce entropy-based metrics as a novel manner to represent students' learning behaviours. Employing these metrics, in conjunction with a proven community detection method, we undertake an analysis of learning behaviours across higher- and lower-performing student communities. Furthermore, we examine the impact of the COVID-19 pandemic on these behaviours. The study is grounded in the analysis of empirical data from 391 Software Engineering students over three academic years. Our findings reveal that students in higher-performing communities typically tend to have lower volatility in entropy values and reach stable learning states earlier than their lower-performing counterparts. Importantly, this study provides evidence of the use of entropy as a simple yet insightful metric for educators to monitor study progress, enhance understanding of student engagement, and enable timely interventions.
更多查看译文
关键词
entropy,learning behaviours,learning analytics,educational data mining,community detection,random matrix theory
AI 理解论文
溯源树
样例
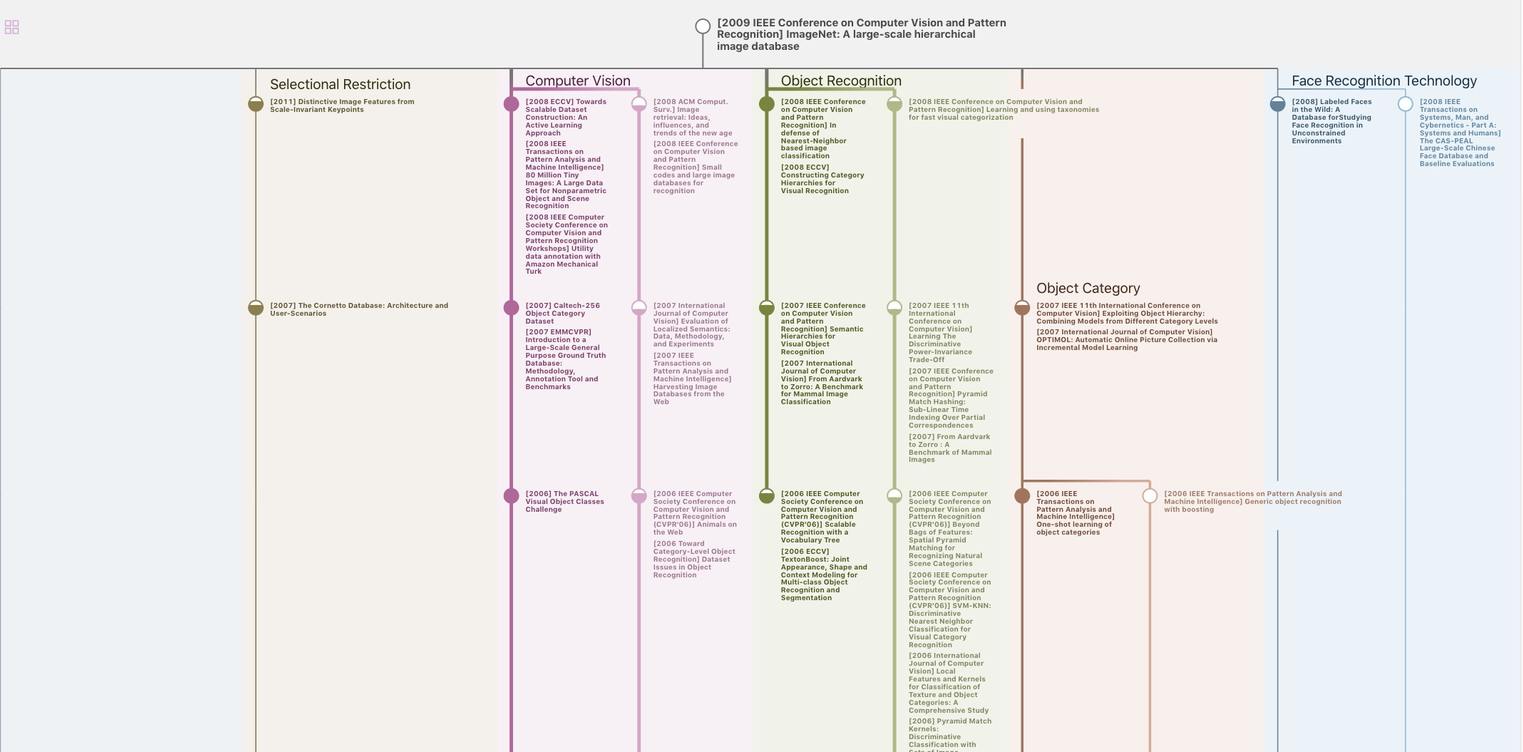
生成溯源树,研究论文发展脉络
Chat Paper
正在生成论文摘要