Target-X: An Efficient Algorithm for Generating Targeted Adversarial Images to Fool Neural Networks.
2023 IEEE 47th Annual Computers, Software, and Applications Conference (COMPSAC)(2023)
摘要
Deep neural networks (DNNs) have achieved a series of significant successes in a wide spectrum of critical domains. For instance, in the field of computer vision, DNNs become the first choice in developing image recognition and classification solutions. However, DNNs have been recently found vulnerable to manipulations of input samples, called adversarial images. The adversarial images can be classified into two categories: untargeted adversarial images which aim to manipulate the output of the DNNs to any incorrect label and targeted adversarial images which force the prediction of the DNNs to a specified target label predefined by the adversary. That being said, the construction of targeted adversarial images requires careful crafting of the targeted perturbations. Different research works have been done to generate targeted adversarial images. However, the majority of them have two limitations: (1) adding large size of perturbations to generate successfully targeted images, and (2) they require extensive computational resources to be utilized in large-scale datasets. This paper introduces Target-X, a novel and fast method for the construction of adversarial targeted images on large-scale datasets that can fool the state-of-the-art image classification neural networks. We evaluate the performance of Target-X using the well-trained image classification neural networks of different architectures and compare it with the well-known T-FGSM and T-UAP targeted attacks. The reported results demonstrate that Target-X can generate targeted adversarial images with the least perturbations on large-scale datasets that can fool the image classification neural networks and significantly outperform the T-FGSM and T-UAP attacks.
更多查看译文
关键词
adversarial images, deep learning, image classification neural networks, adversarial deep neural networks
AI 理解论文
溯源树
样例
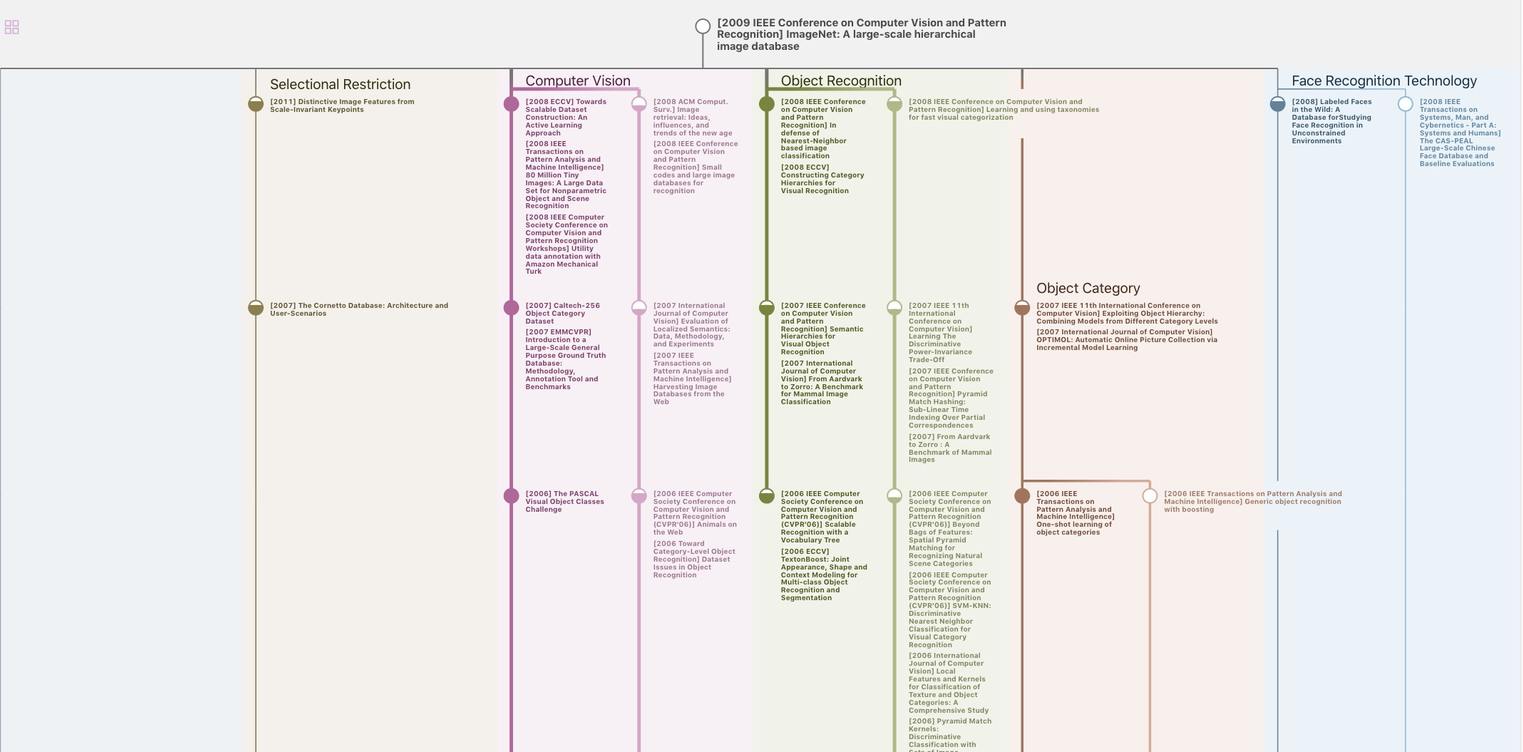
生成溯源树,研究论文发展脉络
Chat Paper
正在生成论文摘要