Powerful Significance Testing for Unbalanced Clusters
arXiv (Cornell University)(2023)
摘要
Clustering methods are popular for revealing structure in data, particularly in the high-dimensional setting common to contemporary data science. A central statistical question is, "are the clusters really there?" One pioneering method in statistical cluster validation is SigClust, but it is severely underpowered in the important setting where the candidate clusters have unbalanced sizes, such as in rare subtypes of disease. We show why this is the case, and propose a remedy that is powerful in both the unbalanced and balanced settings, using a novel generalization of k-means clustering. We illustrate the value of our method using a high-dimensional dataset of gene expression in kidney cancer patients. A Python implementation is available at https://github.com/thomaskeefe/sigclust.
更多查看译文
关键词
unbalanced clusters,powerful significance testing
AI 理解论文
溯源树
样例
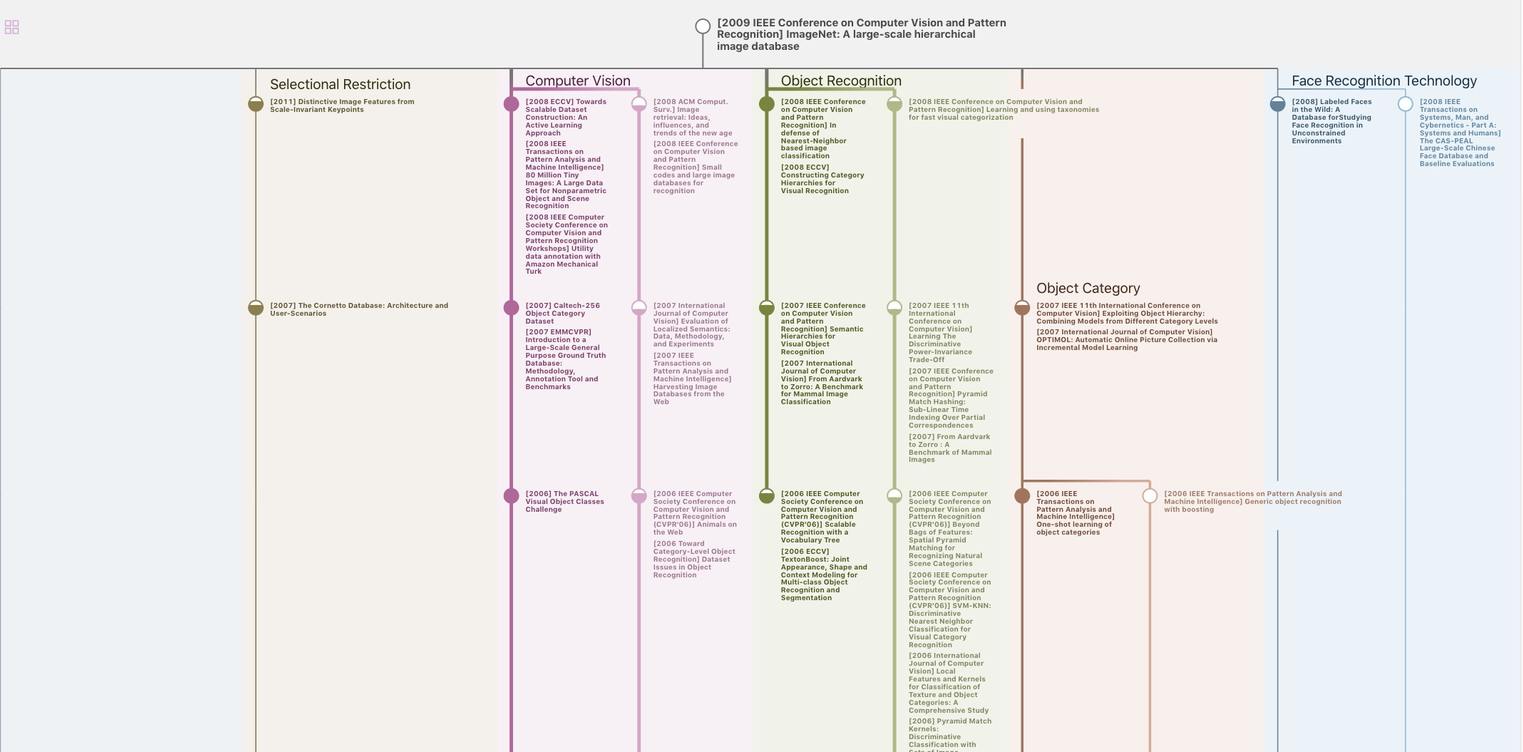
生成溯源树,研究论文发展脉络
Chat Paper
正在生成论文摘要