DAG-ACFL: Asynchronous Clustered Federated Learning based on DAG-DLT
CoRR(2023)
摘要
Federated learning (FL) aims to collaboratively train a global model while ensuring client data privacy. However, FL faces challenges from the non-IID data distribution among clients. Clustered FL (CFL) has emerged as a promising solution, but most existing CFL frameworks adopt synchronous frameworks lacking asynchrony. An asynchronous CFL framework called SDAGFL based on directed acyclic graph distributed ledger techniques (DAG-DLT) was proposed, but its complete decentralization leads to high communication and storage costs. We propose DAG-ACFL, an asynchronous clustered FL framework based on directed acyclic graph distributed ledger techniques (DAG-DLT). We first detail the components of DAG-ACFL. A tip selection algorithm based on the cosine similarity of model parameters is then designed to aggregate models from clients with similar distributions. An adaptive tip selection algorithm leveraging change-point detection dynamically determines the number of selected tips. We evaluate the clustering and training performance of DAG-ACFL on multiple datasets and analyze its communication and storage costs. Experiments show the superiority of DAG-ACFL in asynchronous clustered FL. By combining DAG-DLT with clustered FL, DAG-ACFL realizes robust, decentralized and private model training with efficient performance.
更多查看译文
关键词
asynchronous clustered federated learning,dag-acfl,dag-dlt
AI 理解论文
溯源树
样例
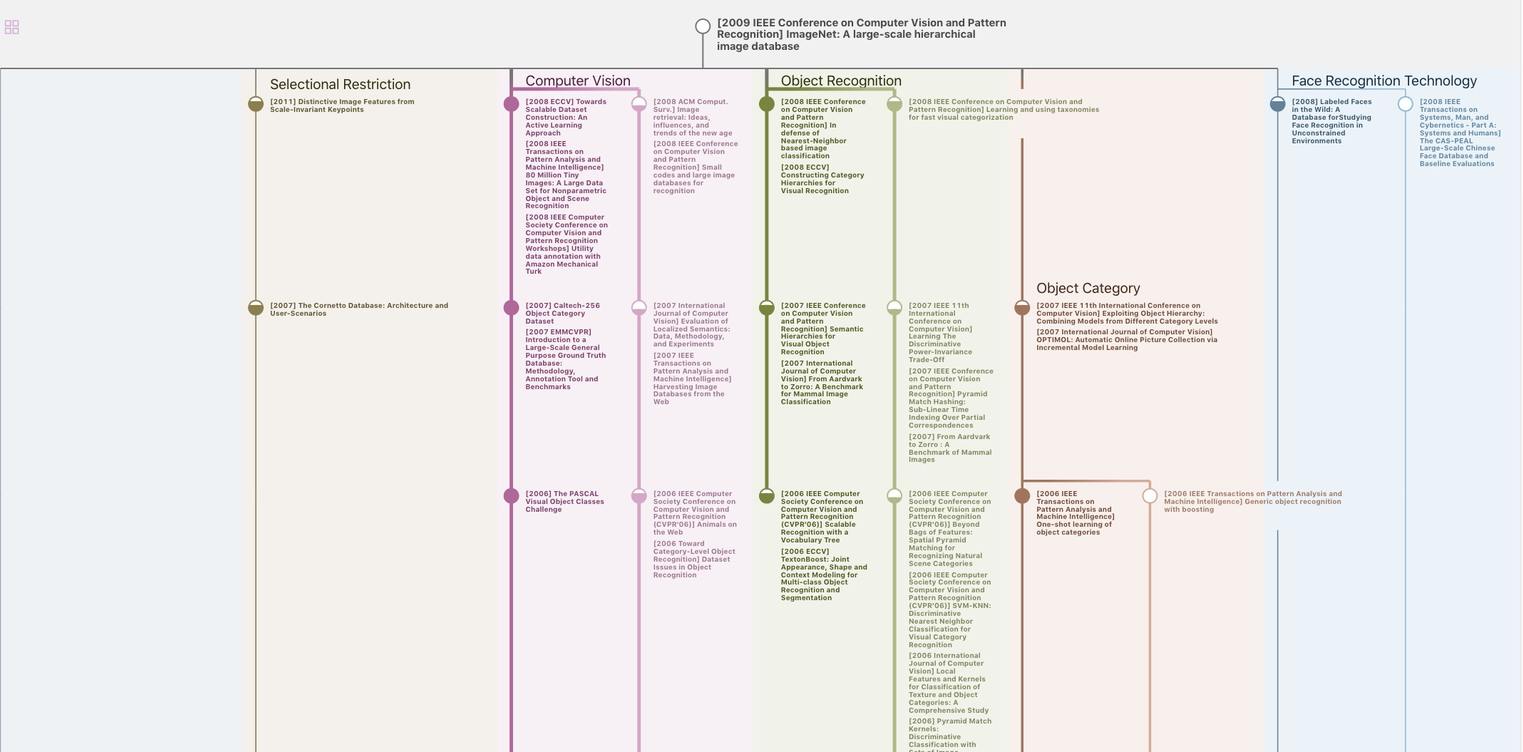
生成溯源树,研究论文发展脉络
Chat Paper
正在生成论文摘要