Unleashing the Power of Language Models in Clinical Settings: A Trailblazing Evaluation Unveiling Novel Test Design
medRxiv (Cold Spring Harbor Laboratory)(2023)
摘要
The realm of clinical medicine stands on the brink of a revolutionary breakthrough as large language models (LLMs) emerge as formidable allies, propelled by the prowess of deep learning and a wealth of clinical data. Yet, amidst the disquieting specter of misdiagnoses haunting the halls of medical treatment, LLMs offer a glimmer of hope, poised to reshape the landscape. However, their mettle and medical acumen, particularly in the crucible of real-world professional scenarios replete with intricate logical interconnections, re-main shrouded in uncertainty. To illuminate this uncharted territory, we present an audacious quantitative evaluation method, harnessing the ingenuity of tho-racic surgery questions as the litmus test for LLMs’ medical prowess. These clinical questions covering various diseases were collected, and a test format consisting of multi-choice questions and case analysis was designed based on the Chinese National Senior Health Professional Technical Qualification Examination. Five LLMs of different scales and sources were utilized to answer these questions, and evaluation and feedback were provided by professional thoracic surgeons. Among these models, GPT-4 demonstrated the highest performance with a score of 48.67 out of 100, achieving accuracies of 0.62, 0.27, and 0.63 in single-choice, multi-choice, and case-analysis questions, respectively. However, further improvement is still necessary to meet the passing threshold of the examination. Additionally, this paper analyzes the performance, advantages, disadvantages, and risks of LLMs, and proposes suggestions for improvement, providing valuable insights into the capabilities and limitations of LLMs in the specialized medical domain.
### Competing Interest Statement
The authors have declared no competing interest.
### Funding Statement
This study did not receive any funding
### Author Declarations
I confirm all relevant ethical guidelines have been followed, and any necessary IRB and/or ethics committee approvals have been obtained.
Yes
I confirm that all necessary patient/participant consent has been obtained and the appropriate institutional forms have been archived, and that any patient/participant/sample identifiers included were not known to anyone (e.g., hospital staff, patients or participants themselves) outside the research group so cannot be used to identify individuals.
Yes
I understand that all clinical trials and any other prospective interventional studies must be registered with an ICMJE-approved registry, such as ClinicalTrials.gov. I confirm that any such study reported in the manuscript has been registered and the trial registration ID is provided (note: if posting a prospective study registered retrospectively, please provide a statement in the trial ID field explaining why the study was not registered in advance).
Yes
I have followed all appropriate research reporting guidelines, such as any relevant EQUATOR Network research reporting checklist(s) and other pertinent material, if applicable.
Yes
All data produced in the present study are available upon reasonable request to the authors.
更多查看译文
关键词
language models,clinical settings,test
AI 理解论文
溯源树
样例
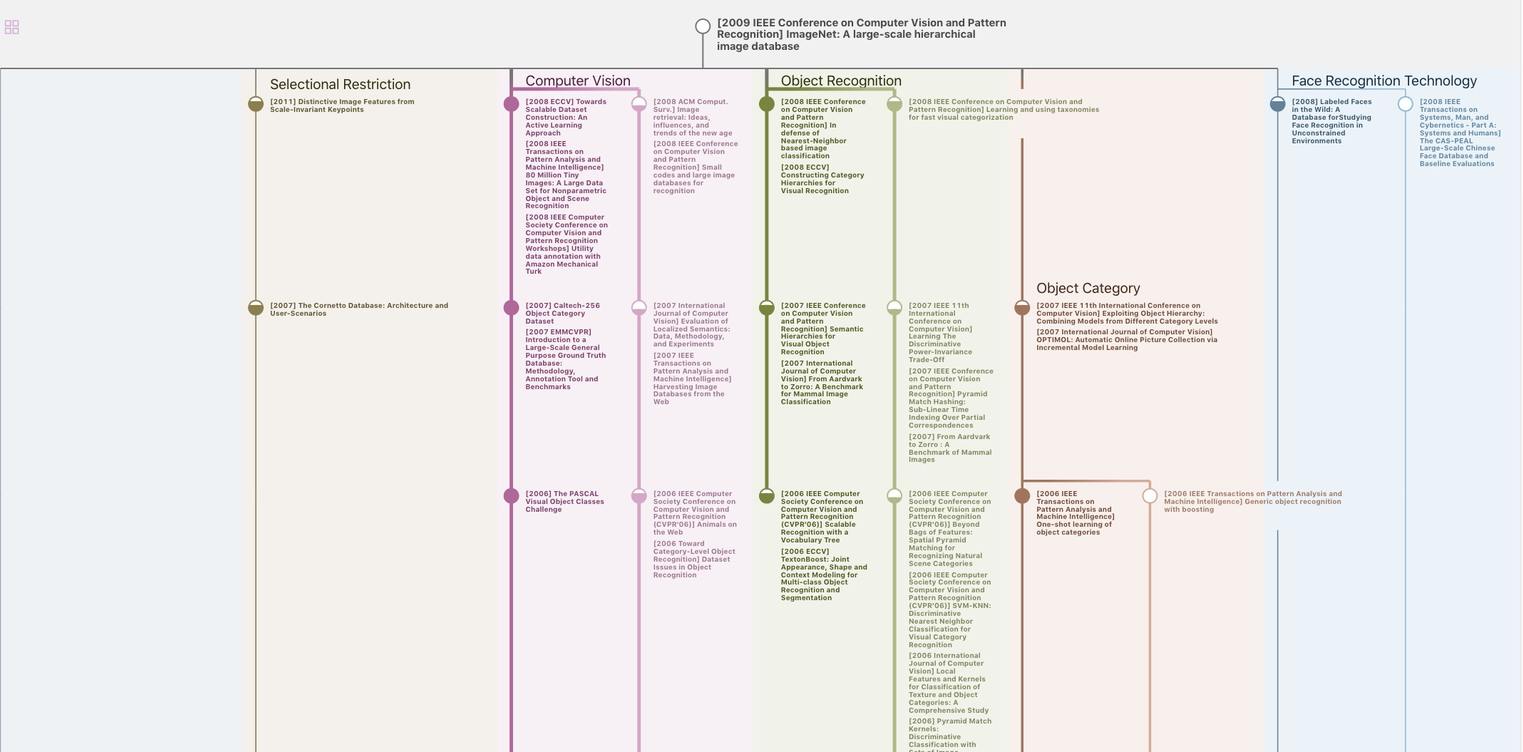
生成溯源树,研究论文发展脉络
Chat Paper
正在生成论文摘要