Automated Image Transcription for Perinatal Blood Pressure Monitoring Using Mobile Health Technology
medRxiv (Cold Spring Harbor Laboratory)(2023)
摘要
This paper introduces a novel approach to address the challenges associated with transferring blood pressure (BP) data from oscillometric devices used in self-measured BP monitoring systems. The primary objective of this study is to improve the accessibility and usability of BP data for monitoring and managing BP during pregnancy and postpartum, particularly in low-resource settings. To this end, we developed an automated image transcription technique to effectively transcribe readings from BP devices. The photos of the BP devices were captured as part of perinatal mobile health (mHealth) monitoring systems, conducted in four studies across two countries. The Guatemala Set 1 and Guatemala Set 2 datasets include the data captured by a cohort of 49 lay midwives from 1697 and 584 pregnant women carrying singletons in the second and third trimesters in rural Guatemala during routine screening. Additionally, we designed an mHealth system in Georgia for postpartum women to monitor and report their BP at home with 23 and 49 African American participants contributing to the Georgia I3 and Georgia IMPROVE projects, respectively. We developed a deep learning-based model which operates in two steps: LCD localization using the You Only Look Once (YOLO) object detection model and digit recognition using a convolutional neural network-based model capable of recognizing multiple digits. We applied color correction and thresholding techniques to minimize the impact of reflection and artifacts. Three experiments were conducted based on the devices used for training the digit recognition model. Overall, our results demonstrate that the device-specific model with transfer learning and the device independent model outperformed the device-specific model without transfer learning. The mean absolute error (MAE) of image transcription on held-out test datasets using the device-independent digit recognition were 1.1 and 1.1 mmHg for systolic and diastolic BP in the Georgia IMPROVE and 1 and 0.6 mmHg in Guatemala Set 2 datasets. The MAE, far below the FDA requirement of 5 mmHg, makes the proposed model suitable for general use when used with appropriate error devices.
### Competing Interest Statement
The authors have declared no competing interest.
### Funding Statement
Research reported in this publication was supported in part by the National Institutes of Health, the Fogarty International Center and the Eunice Kennedy Shriver National Institute of Child Health and Human Development (NICHD), grant number 1R21HD084114-01 (Mobile Health Intervention to Improve Perinatal Continuum of Care in Guatemala), NICHD grant number 1R01HD110480 (AI-driven low-cost ultrasound for automated quantification of hypertension, preeclampsia, and IUGR), the Imagine, Innovate and Impact (I3) Funds from the Emory School of Medicine and through the Georgia IMPROVE, funded by NIH National Center for Advancing Translational Sciences (NCATS) as an Administrative Supplement to the Georgia Clinical and Translational Alliance (UL1-TR002378). The content is solely the responsibility of the authors and does not necessarily represent the official views of the National Institutes of Health. We would also like to acknowledge the support of the Grady Health System, Atlanta, Georgia in conducting this research.
### Author Declarations
I confirm all relevant ethical guidelines have been followed, and any necessary IRB and/or ethics committee approvals have been obtained.
Yes
The details of the IRB/oversight body that provided approval or exemption for the research described are given below:
Ethics committee/IRB of Emory University and Morehouse School of Medicine gave ethical approval for this work.
I confirm that all necessary patient/participant consent has been obtained and the appropriate institutional forms have been archived, and that any patient/participant/sample identifiers included were not known to anyone (e.g., hospital staff, patients or participants themselves) outside the research group so cannot be used to identify individuals.
Yes
I understand that all clinical trials and any other prospective interventional studies must be registered with an ICMJE-approved registry, such as ClinicalTrials.gov. I confirm that any such study reported in the manuscript has been registered and the trial registration ID is provided (note: if posting a prospective study registered retrospectively, please provide a statement in the trial ID field explaining why the study was not registered in advance).
Yes
I have followed all appropriate research reporting guidelines, such as any relevant EQUATOR Network research reporting checklist(s) and other pertinent material, if applicable.
Yes
All data produced in the present study are available upon reasonable request to the authors
更多查看译文
关键词
perinatal blood pressure monitoring,blood pressure,transcription
AI 理解论文
溯源树
样例
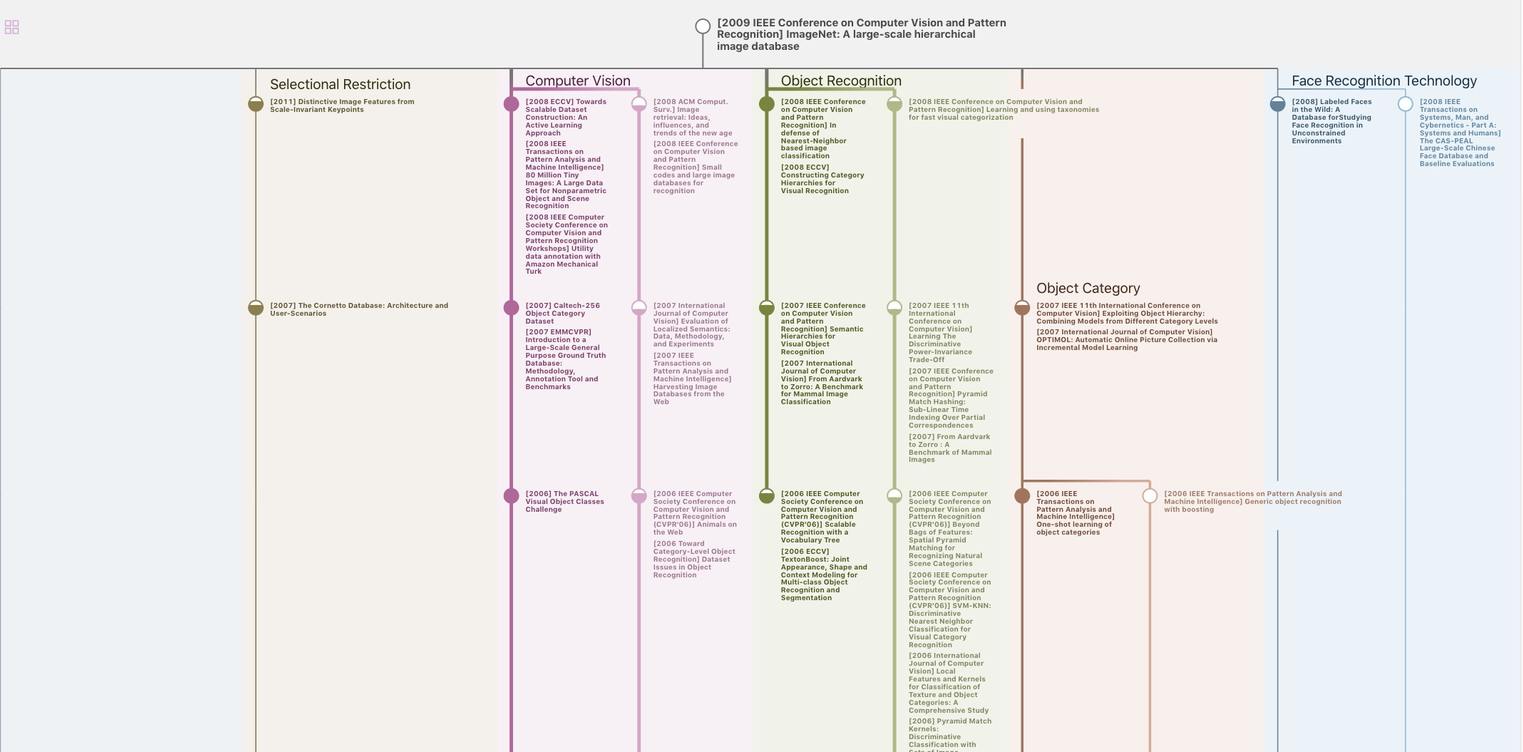
生成溯源树,研究论文发展脉络
Chat Paper
正在生成论文摘要