A Two-Layer Model Predictive Path-Tracking Control with Curvature Adaptive Method for High-Speed Autonomous Driving
IEEE Access(2023)
摘要
A two-layer model predictive control (MPC) algorithm with curvature adaptive is introduced and adopted in path tracking, especially for high-speed autonomous driving. Whether the vehicle can stably reach a safe driving speed in advance is the main consideration of rollover and speed-overshoot avoidance, especially when the trajectory with large curvatures. Thus, the outer layer of the proposed controller, which is built based on the vehicle kinematic model, generates an optimal vehicle velocity. And, the inner layer controller that is established according to the vehicle dynamics provides an optimal front wheel angle obtained combined with the optimal tracking trajectory generated by the path planner. The cross-track error, which is considered an important judging criterion of tracking and obstacle avoidance, is chosen here to validate the control performance. With the MATLAB/Simulink-Carmaker platform for modeling, the two-layer MPC has good performance in a continuous curve, simple obstacle avoidance, and complex obstacle avoidance scenarios. Notably, when the average driving speed reaches $108km {/}h$ , the cross-track error can be controlled within $0.21m$ when employing the proposed MPC method. In contrast, the conventional MPC method yields a cross-track error exceeding $3.4m$ , while an LQR-based strategy results in a maximum error of $0.64m$ . The proposed two-layer MPC algorithm with curvature adaptivity significantly enhances path-tracking performance, particularly for high-speed autonomous driving. By effectively controlling the cross-track error and considering various driving scenarios, it successfully ensures safe and stable driving speeds, thereby mitigating the risks associated with rollover and speed overshoot.
更多查看译文
关键词
Path tracking,two-layer MPC,curvature adaptive,path planning,high-speed,energy-saving,obstacle avoidance
AI 理解论文
溯源树
样例
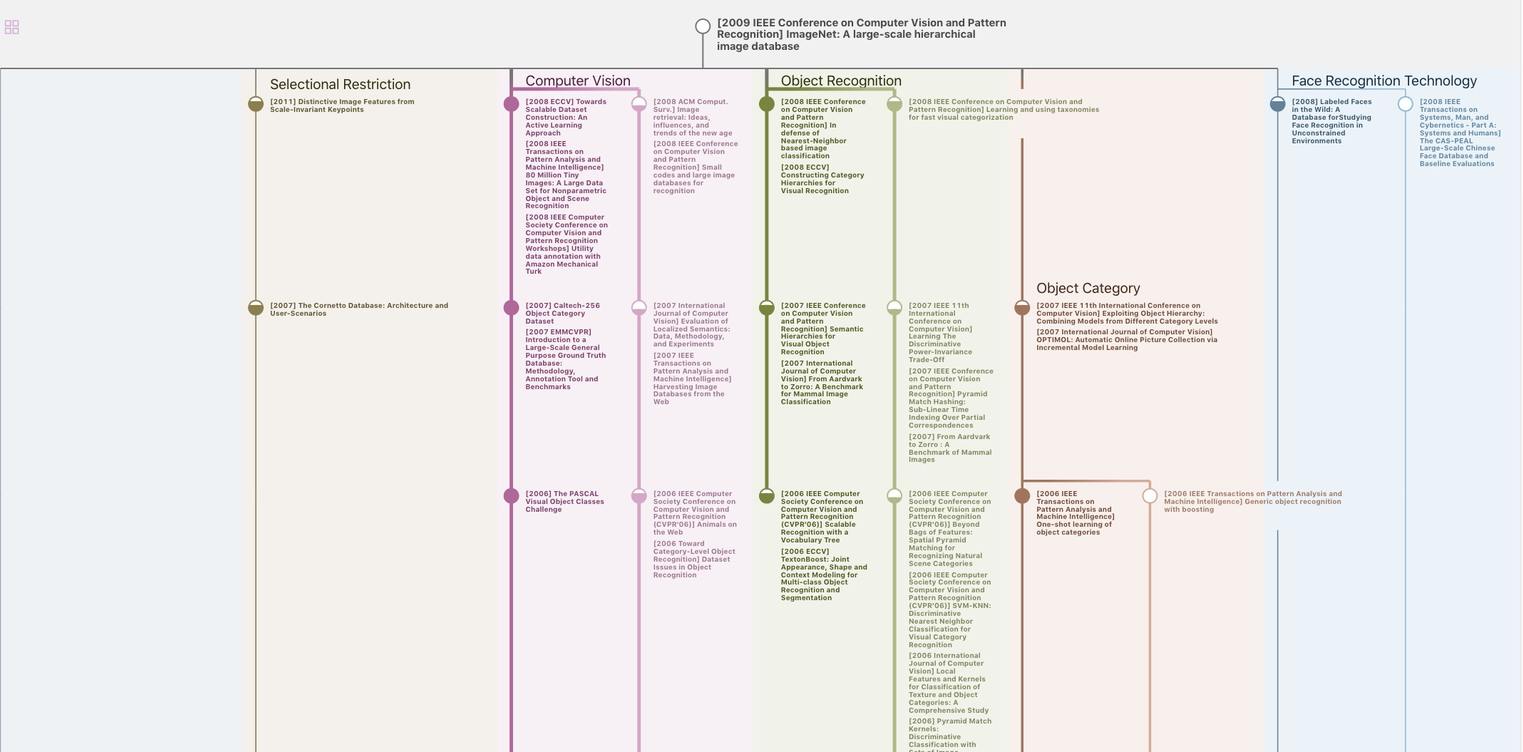
生成溯源树,研究论文发展脉络
Chat Paper
正在生成论文摘要