Stealthy Adversarial Attacks Against Automated Modulation Classification in Cognitive Radio
2023 IEEE Cognitive Communications for Aerospace Applications Workshop (CCAAW)(2023)
摘要
In cognitive radio systems, wireless spectrum sensing plays a crucial role in identifying the state of the wireless environment, which leads to the effective utilization of scarce spectral resources for various application fields. As a critical part of wireless spectrum sensing, automatic modulation classification (AMC) is used to identify the modulation types of the received signals automatically. With the advances in sensing and computing technologies, artificial intelligence (AI), especially deep learning (DL), has been widely applied to enhance the effectiveness and timely response of AMC. However, in recent years, increasing evidence shows that carefully-crafted adversarial noise can mislead learning models, which raises concerns about the trustworthiness of DL solutions in AMC. Therefore, it is crucial to sufficiently mitigate the adversarial perturbations in DL-powered AMC. To realize a successful mitigation strategy, it can be beneficial to first adopt an adversarial mindset and formulate threat models of practical perturbations in DL-powered AMC. However, there is still limited work focusing on generating practical adversarial attacks in DL-powered AMC that has complex domain knowledge. In this paper, we propose an innovative and stealthy adversarial attack method that can practically compromise the DL-based decision-making of AMCs. In the performance evaluation section, different scenarios will be considered to illustrate the effectiveness of our proposed adversarial attack method in achieving a high success rate and sufficient stealthiness in AMC.
更多查看译文
关键词
Automatic Modulation Classification, Adversarial Attack, Deep Learning, Cognitive Radio
AI 理解论文
溯源树
样例
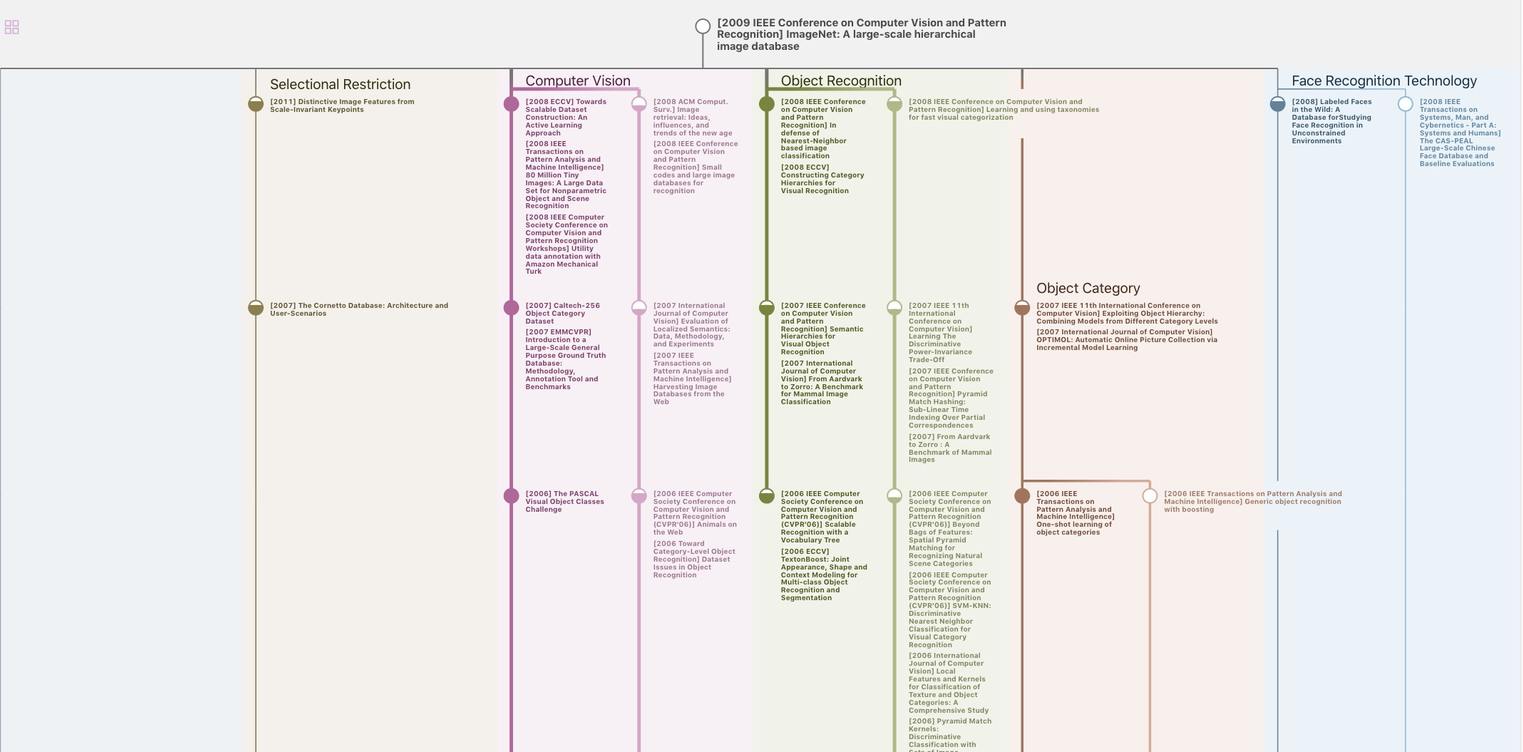
生成溯源树,研究论文发展脉络
Chat Paper
正在生成论文摘要