SamDSK: Combining Segment Anything Model with Domain-Specific Knowledge for Semi-Supervised Learning in Medical Image Segmentation
CoRR(2023)
摘要
The Segment Anything Model (SAM) exhibits a capability to segment a wide array of objects in natural images, serving as a versatile perceptual tool for various downstream image segmentation tasks. In contrast, medical image segmentation tasks often rely on domain-specific knowledge (DSK). In this paper, we propose a novel method that combines the segmentation foundation model (i.e., SAM) with domain-specific knowledge for reliable utilization of unlabeled images in building a medical image segmentation model. Our new method is iterative and consists of two main stages: (1) segmentation model training; (2) expanding the labeled set by using the trained segmentation model, an unlabeled set, SAM, and domain-specific knowledge. These two stages are repeated until no more samples are added to the labeled set. A novel optimal-matching-based method is developed for combining the SAM-generated segmentation proposals and pixel-level and image-level DSK for constructing annotations of unlabeled images in the iterative stage (2). In experiments, we demonstrate the effectiveness of our proposed method for breast cancer segmentation in ultrasound images, polyp segmentation in endoscopic images, and skin lesion segmentation in dermoscopic images. Our work initiates a new direction of semi-supervised learning for medical image segmentation: the segmentation foundation model can be harnessed as a valuable tool for label-efficient segmentation learning in medical image segmentation.
更多查看译文
关键词
combining segment anything model,segmentation,learning,domain-specific,semi-supervised
AI 理解论文
溯源树
样例
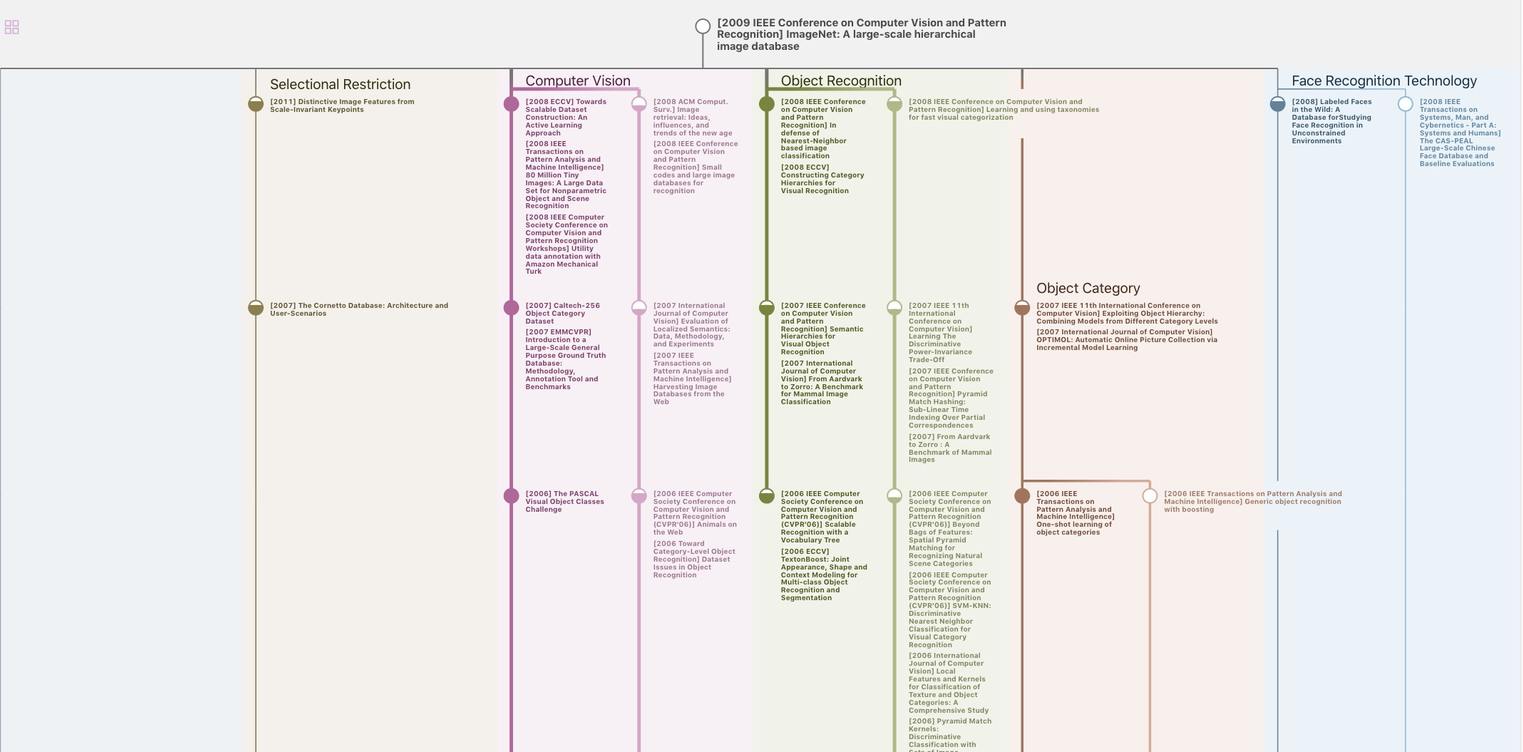
生成溯源树,研究论文发展脉络
Chat Paper
正在生成论文摘要