Revolutionizing Disease Diagnosis: A Microservices-Based Architecture for Privacy-Preserving and Efficient IoT Data Analytics Using Federated Learning
CoRR(2023)
摘要
Deep learning-based disease diagnosis applications are essential for accurate diagnosis at various disease stages. However, using personal data exposes traditional centralized learning systems to privacy concerns. On the other hand, by positioning processing resources closer to the device and enabling more effective data analyses, a distributed computing paradigm has the potential to revolutionize disease diagnosis. Scalable architectures for data analytics are also crucial in healthcare, where data analytics results must have low latency and high dependability and reliability. This study proposes a microservices-based approach for IoT data analytics systems to satisfy privacy and performance requirements by arranging entities into fine-grained, loosely connected, and reusable collections. Our approach relies on federated learning, which can increase disease diagnosis accuracy while protecting data privacy. Additionally, we employ transfer learning to obtain more efficient models. Using more than 5800 chest X-ray images for pneumonia detection from a publically available dataset, we ran experiments to assess the effectiveness of our approach. Our experiments reveal that our approach performs better in identifying pneumonia than other cutting-edge technologies, demonstrating our approach's promising potential detection performance.
更多查看译文
关键词
Microservices architecture, federated learning,transfer learning, data analytics,microservices,disease detection,pneumonia, privacy
AI 理解论文
溯源树
样例
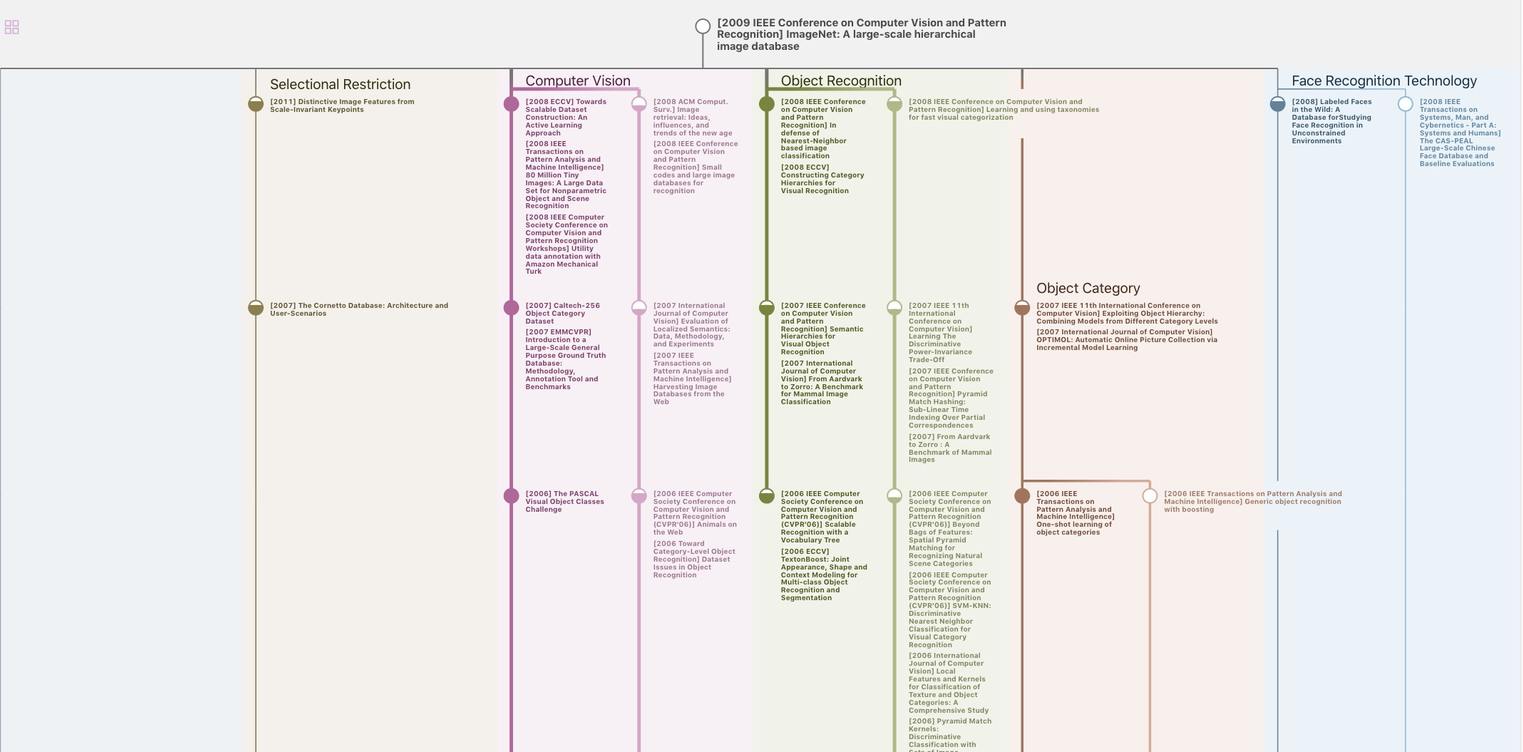
生成溯源树,研究论文发展脉络
Chat Paper
正在生成论文摘要