SuperUDF: Self-supervised UDF Estimation for Surface Reconstruction.
IEEE transactions on visualization and computer graphics(2023)
摘要
Learning-based surface reconstruction based on unsigned distance functions (UDF) has many advantages such as handling open surfaces. We propose SuperUDF, a self-supervised UDF learning which exploits a learned geometry prior for efficient training and a novel regularization for robustness to sparse sampling. The core idea of SuperUDF draws inspiration from the classical surface approximation operator of locally optimal projection (LOP). The key insight is that if the UDF is estimated correctly, the 3D points should be locally projected onto the underlying surface following the gradient of the UDF. Based on that, a number of inductive biases on UDF geometry and a pre-learned geometry prior are devised to learn UDF estimation efficiently. A novel regularization loss is proposed to make SuperUDF robust to sparse sampling. Furthermore, we also contribute a learning-based mesh extraction from the estimated UDFs. Extensive evaluations demonstrate that SuperUDF outperforms the state of the arts on several public datasets in terms of both quality and efficiency. Code will be released after accteptance.
更多查看译文
关键词
UDF,Point Cloud Reconstruction,Implicit Surface
AI 理解论文
溯源树
样例
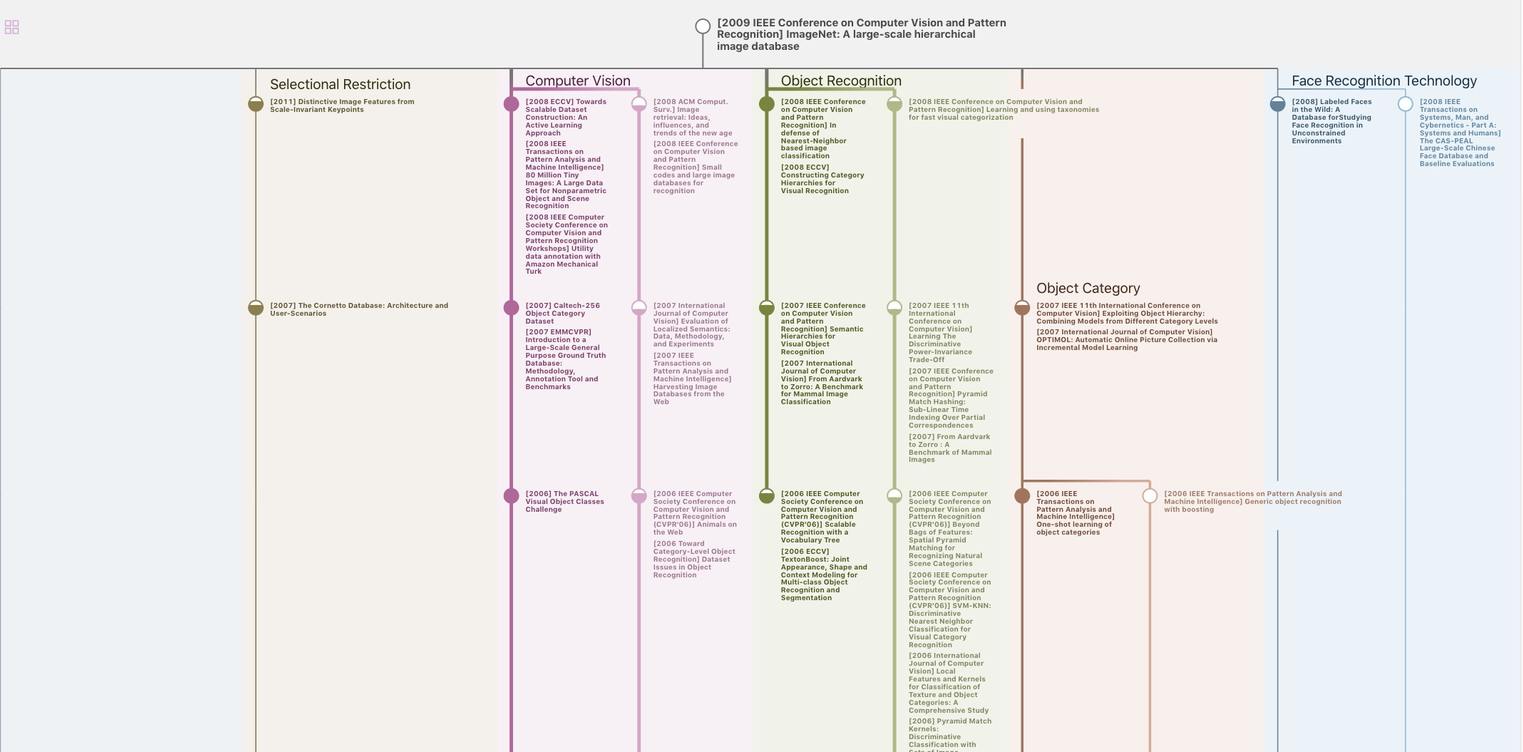
生成溯源树,研究论文发展脉络
Chat Paper
正在生成论文摘要