Low-complexity Samples versus Symbols-based Neural Network Receiver for Channel Equalization
Journal of Lightwave Technology(2023)
摘要
Low-complexity neural networks (NNs) have successfully been applied for digital signal processing (DSP) in short-reach intensity-modulated directly detected optical links, where chromatic dispersion-induced impairments significantly limit the transmission distance. The NN-based equalizers are usually optimized independently from other DSP components, such as matched filtering. This approach may result in lower equalization performance. Alternatively, optimizing a NN equalizer to perform functionalities of multiple DSP blocks may increase transmission reach while keeping the complexity low. In this work, we propose a low-complexity NN that performs samples-to-symbol equalization, meaning that the NN-based equalizer includes match filtering and downsampling. We compare it to a samples-to-sample equalization approach followed by match filtering and downsampling in terms of performance and computational complexity. Both approaches are evaluated using three different types of NNs combined with optical preprocessing. We numerically and experimentally show that the proposed samples-to-symbol equalization approach applied for 32 GBd on-off keying (OOK) signals outperforms the samples-domain alternative keeping the computational complexity low. Additionally, the different types of NN-based equalizers are compared in terms of performance with respect to computational complexity.
更多查看译文
关键词
Neural Network equalizer,Optical communications,Intensity-modulation,Direct-detection
AI 理解论文
溯源树
样例
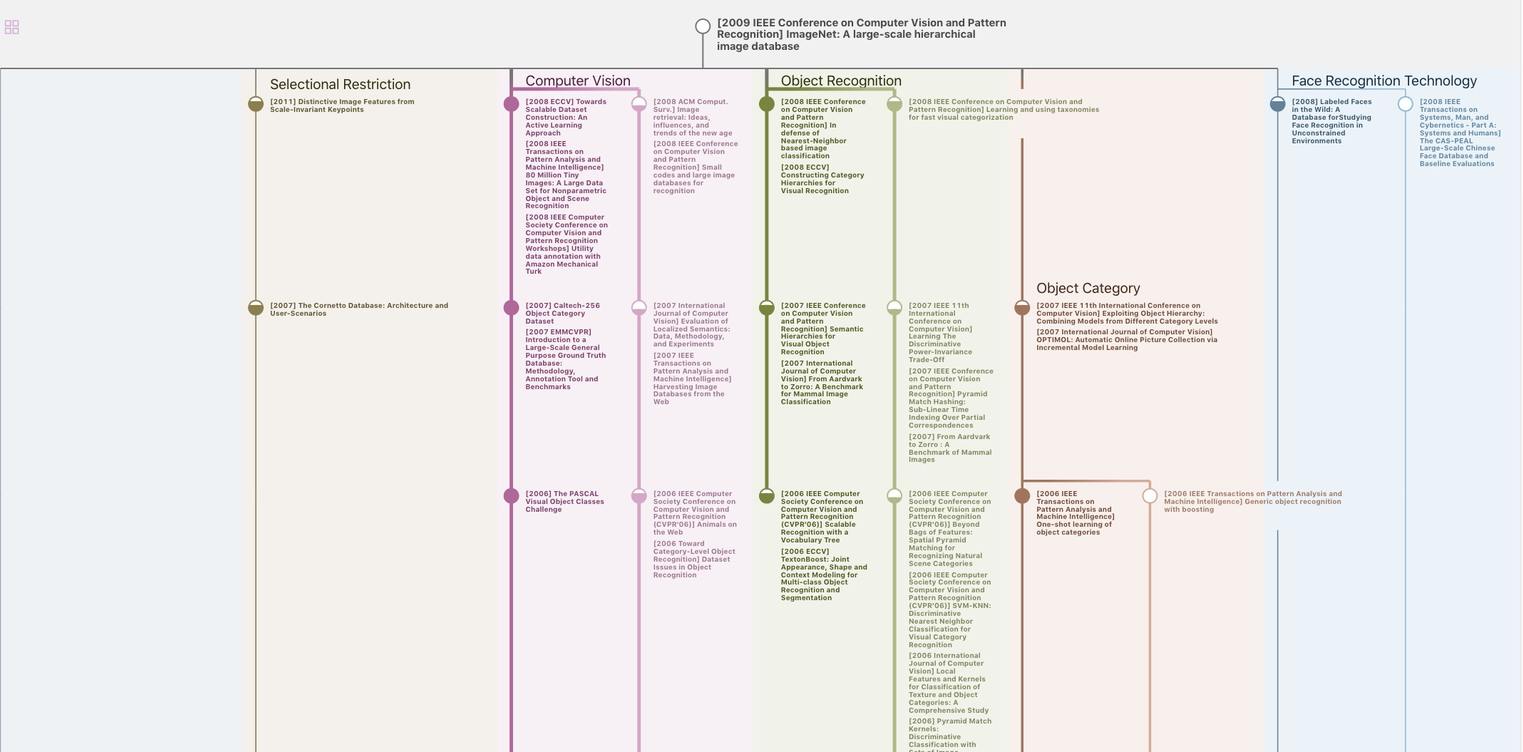
生成溯源树,研究论文发展脉络
Chat Paper
正在生成论文摘要