Group Regression for Query Based Object Detection and Tracking
CoRR(2023)
摘要
Group regression is commonly used in 3D object detection to predict box parameters of similar classes in a joint head, aiming to benefit from similarities while separating highly dissimilar classes. For query-based perception methods, this has, so far, not been feasible. We close this gap and present a method to incorporate multi-class group regression, especially designed for the 3D domain in the context of autonomous driving, into existing attention and query-based perception approaches. We enhance a transformer based joint object detection and tracking model with this approach, and thoroughly evaluate its behavior and performance. For group regression, the classes of the nuScenes dataset are divided into six groups of similar shape and prevalence, each being regressed by a dedicated head. We show that the proposed method is applicable to many existing transformer based perception approaches and can bring potential benefits. The behavior of query group regression is thoroughly analyzed in comparison to a unified regression head, e.g. in terms of class-switching behavior and distribution of the output parameters. The proposed method offers many possibilities for further research, such as in the direction of deep multi-hypotheses tracking.
更多查看译文
关键词
query based object detection,tracking,group,regression
AI 理解论文
溯源树
样例
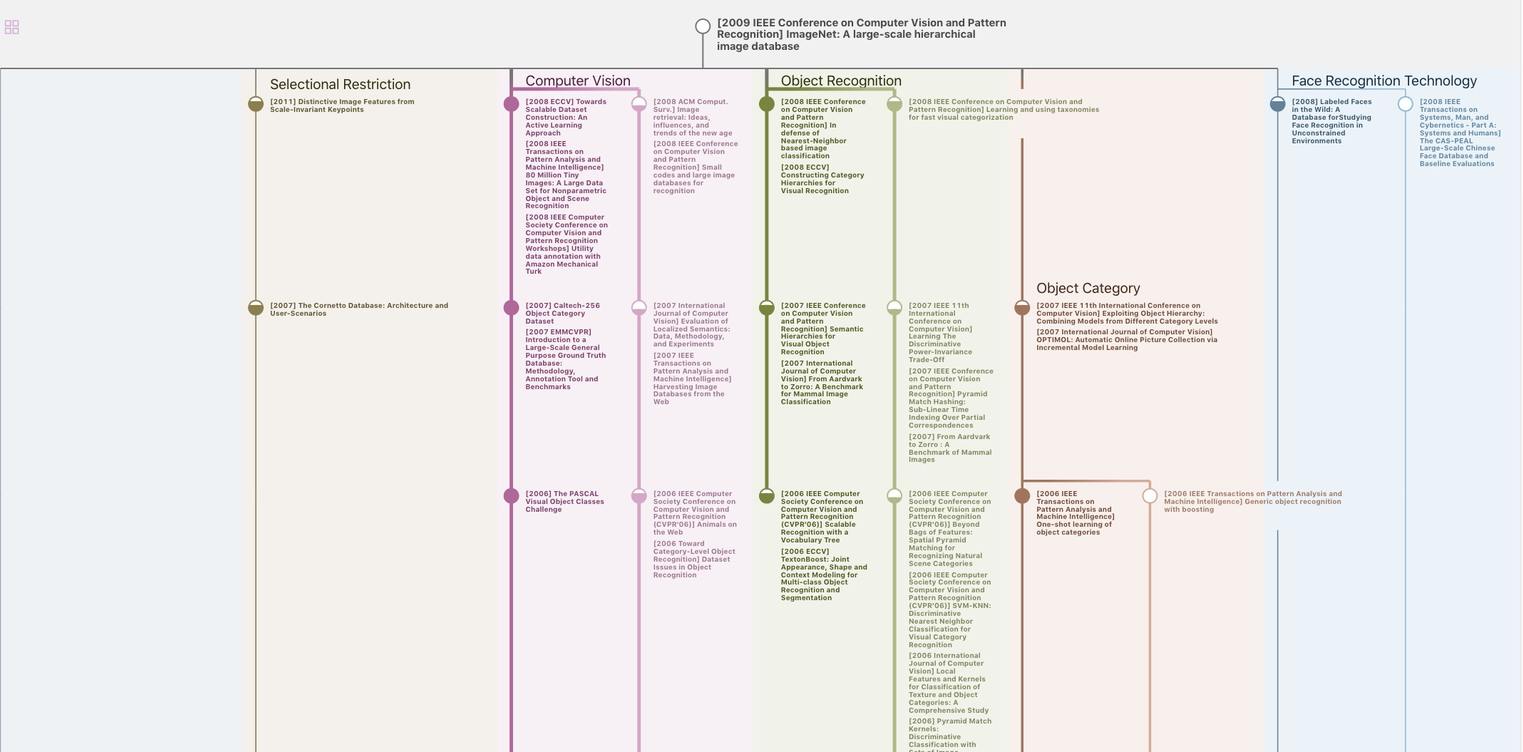
生成溯源树,研究论文发展脉络
Chat Paper
正在生成论文摘要