Partners in Crime: Inferring Cell-to-cell Interactions in Kidney Allograft Rejection From Single-cell RNA Sequencing.
Transplantation(2023)
摘要
Single-cell RNA sequencing (scRNA-seq) of kidney transplants has uncovered a functional role for noncanonical sources of cell types in the allograft such as donor-derived macrophages demonstrating antigen presentation and complement signaling, and recipient-derived graft infiltrating fibroblasts demonstrating increased expression of extracellular matrix proteins.1,2 Although this understanding of cellular-level events provides new insights, an essential piece of the puzzle remains to be solved—the signaling events during cell-to-cell interaction (CCI). Individual cells interact with other cells through physical contact, surface receptor-ligand interaction, cellular junctions, and secreted factors. Such CCIs are a key requisite for the coordination of cellular activities in multicellular organisms.3 In cell-mediated rejection of the kidney allograft, for example, the invitation to lymphocytes, their transendothelial migration, and interaction with tubular epithelium are highly orchestrated molecular events. Different proteins, which include ligand-receptor, receptor-receptor, and extracellular matrix-receptor interactions, mediate the key signaling events during CCI. Although proteomic technologies are desirable to decipher their biological relevance, advances in sequencing technologies have enabled the routine analyses of CCI using transcriptomics. Current computational tools to study CCI from gene expression fall under 4 broad categories based on the mathematical models used as follows: (i) differential combination-based (analyzes differentially expressed genes for interacting ligand-receptor pairs), (ii) expression permutation-based (computes a communication score for each ligand-receptor pair and assesses its significance through cluster-based nonparametric permutation tests), (iii) network-based (exploits the properties of connections between genes, builds a network of ligand-receptor relationships, and computes a score), and (iv) tensor-based (models the ligand-receptor interactions using a tensor—a mathematical function that maps a selection of vectors to a numerical value).3 Visualization methods including Sankey diagrams, Circos plots, and bipartite networks facilitate the interpretation of results. Using such computational tools, scRNA-seq in kidney transplants has highlighted unexpected findings such as collecting duct epithelia actively coordinating mast cells in an allograft kidney with mixed rejection4 and a subpopulation of peritubular capillary endothelium characterized by the unique expression of the structurally and functionally related cytokines CXCL9, 10, and 11, that are chemoattractant to CXCR3-expressing T cells, in an allograft kidney after successful treatment of active antibody-mediated rejection.2 In the 10× Genomics Chromium, for example, a popular scRNA-seq platform, single-cell suspension of the biospecimen, reverse transcription reagents, and gel beads containing barcoded oligonucleotides are combined to form reaction vesicles in an oil drop.5 Thousands of such vesicles are formed, and each oil drop is expected to contain a single bead and a single cell. Inside each vesicle, the cell is lysed, and its mRNAs are reverse transcribed. As the number of cells loaded onto the 10× chip increases, the chance of two or more cells partitioning together into the same oil drop increases. In the version 3.1 assay, such cell doublet/multiplet rates are ~0.8% when capturing 1000 cells to ~8% when capturing 10 000 cells. These are typically discarded as technical artifacts during the analysis. One person’s trash is another’s treasure. Accordingly, a doublet need not always be an artifact but could be a pair of physically interacting cells (PIC). Recently, Giladi et al described PIC-seq, a technique for profiling PICs.6 PIC-seq combines scRNA-seq data of the doublet PIC and both the single cells that constitute the doublet to computationally deconvolve each sequenced doublet into the 2 contributing single cells. However, several assumptions underpin the computational framework: (i) PICs are composed of cells derived from 2 distinct, transcriptionally dissimilar, single-cell populations, A and B; (ii) the contributing cells of each PIC can be approximated as a sampling of 2 representative cells from the background singlet datasets of populations, A and B; and (iii) PIC transcripts can be modeled as a linear combination of the contributing partners’ transcripts, mixed at a specific ratio α. By identifying the 2 cellular subtypes that comprise the PIC, the expected expression is computed, and compared with the actual expression of the PIC. If PIC is essentially a doublet, then does the success of PIC-seq depend on the artifactual generation of doublets during sample preparation? No; instead, a milder version of tissue dissociation protocol is employed that selectively enriches PICs in the single-cell suspension.7 Using PIC-seq, Giladi et al mapped T cell–dendritic cell (DC) interactions in cell cultures and showed that this interaction was required for T-cell activation, proliferation, and differentiation.6 For in vivo applications, they were able to assess the crosstalk in epithelial–immune PICs as well as downstream gene regulatory programs in a mouse model of lung development.6 In this issue of the Transplantaion, Leckie-Harre et al report on the first application of PIC-seq in human kidney transplants (Figure 1).8 In a proof of principle study of 11 biopsies across different rejection categories, they show that doublets in scRNA-seq can indeed be biologically relevant PICs. Rather than directly enriching PICs as described in the original protocol, cells from each biopsy were enriched for CD45 surface protein to identify immune PICs. This strategy limited the loss of viable cells for scRNA-seq obtained from precious clinical specimens and ensured at least 1 immune cell would be represented in PICs. They developed a probabilistic model to distinguish PICs from doublets and identified 3 groups of PICs: (i) between plasmacytoid DC and B cells, wherein genes increased in these PICs revealed biological processes that included immune cell activation, regulation of immune responses, and cytokine production, suggesting that plasmacytoid DCs are activated within the kidney allograft on physical contact with B cells; (ii) between macrophages and natural killer (NK) cells, wherein the genes revealed biological processes that included NK cell chemotaxis, cytokine-mediated signaling, and cell killing; and (iii) between endothelial cells and T cells/NK cells that showed increased expression of interferon-gamma inducible proteins CXCL9 and CXCL10, and cell killing as the top biologic pathway in the genes increased in these PICs.FIGURE 1.: RNA sequencing of physically interacting cells. In scRNA-seq, each reaction vesicle—an oil drop, is expected to contain only a single bead and a single cell. However, 2 or more cells can partition together into the same reaction vesicle. PICs are essentially such cell doublets. The messenger RNA transcriptome from each reaction vesicle—whether a PIC or a doublet or >2 cells is sequenced, as if they are a single cell. Tissue dissociation protocol can be altered to enhance the cell suspension for PICs. Various methods are used to distinguish a doublet because of a technical artifact from a doublet due to a PIC. In kidney allograft biopsy single-cell suspensions, Leckie-Harre et al8 used a probabilistic model to distinguish the 2. They then applied PIC-seq, an approach developed by Giladi et al6 that combines the enrichment of PICs in biospecimens with scRNA-seq. PIC-seq systematically maps in situ cellular interactions and characterizes their molecular crosstalk. In simple terms, if m represents the transcriptome of a reaction vesicle containing cell A, n represents the transcriptome of a reaction vesicle containing cell B, then the transcriptome of an artifact doublet containing cells A and B is m + n, whereas, for a PIC doublet containing cells A and B, it is m + n + a, where a is the change in transcriptome because of the interaction between cell A and cell B. The shortcoming of this technique includes but are not limited to the following: (i) currently applicable to study doublets only and not >2 cells in a reaction vesicle, (ii) the algorithm cannot be applied for PICs composed of similar or identical cells, (iii) because different dissociation protocols show preferences toward specific interacting pairs, PIC-seq does not capture the entirety of interactions occurring in vivo, and (iv) it can prove difficult to determine which of the conjugated cells is the source of the transcripts in PIC-specific gene expression. PIC, physically interacting cell; scRNA-seq, single-cell RNA sequencing. Figure created with BioRender.comPIC-seq is a powerful technology but its shortcomings are worth highlighting (Figure 1). In the study by Leckie-Harre et al,8 the small number and size of PIC clusters severely limited the statistical power to detect more PIC-associated genes. Nonetheless, while it’s easy to identify the criminal in the allograft, PIC-seq promises to seek and pick up the partners in the crime!
更多查看译文
关键词
kidney allograft rejection,allograft rejection,rna,cell-to-cell,single-cell
AI 理解论文
溯源树
样例
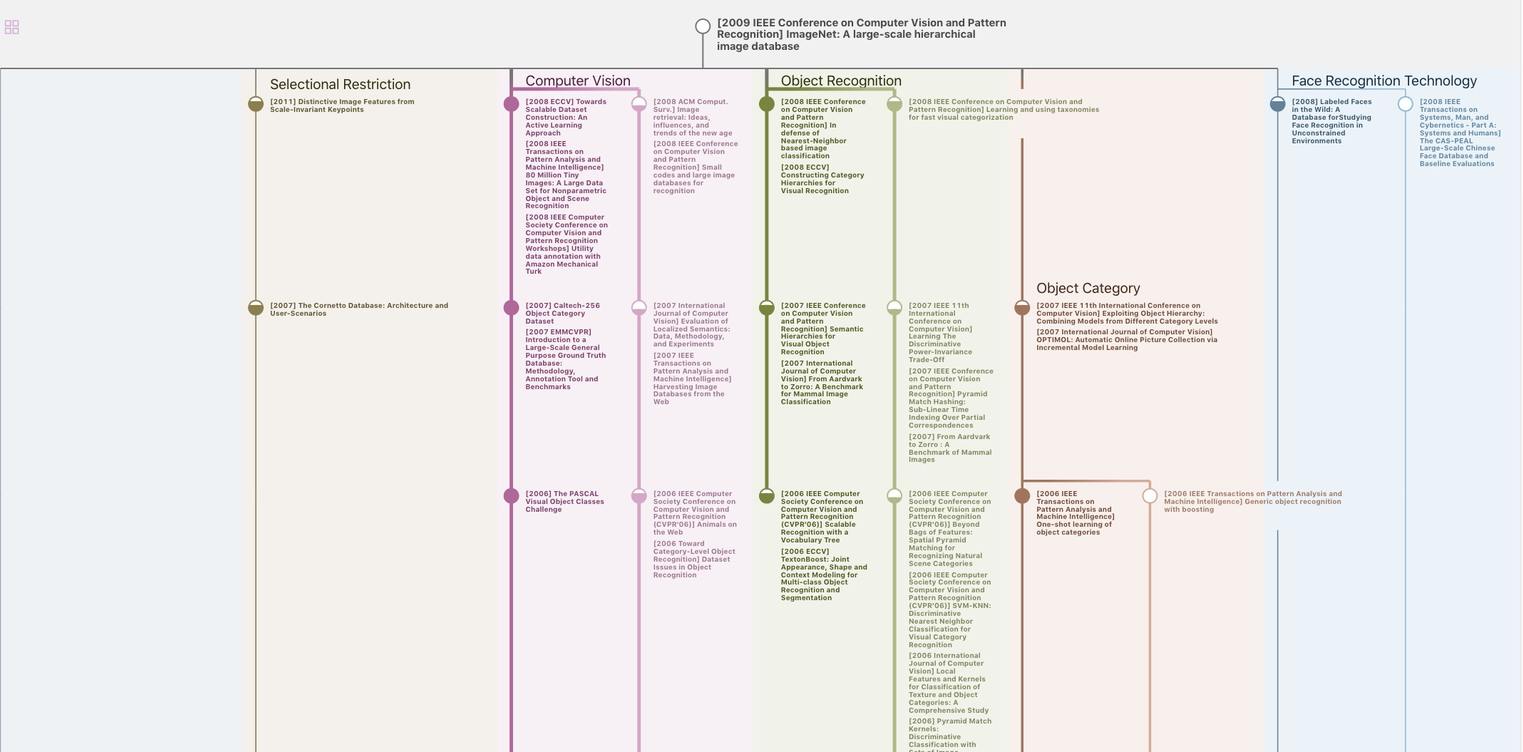
生成溯源树,研究论文发展脉络
Chat Paper
正在生成论文摘要