One-Transistor-Multiple-RRAM Cells for Energy-Efficient In-Memory Computing
2023 21st IEEE Interregional NEWCAS Conference (NEWCAS)(2023)
摘要
The use of resistive random-access memory (RRAM) for in-memory computing (IMC) architectures has significantly improved the energy-efficiency of artificial neural networks (ANN) over the past years. Current RRAM-technologies are physically limited to a defined unambiguously distinguishable number of stable states and a maximum resistive value and are compatible with present complementary metal-oxide semiconductor (CMOS)-technologies. In this work, we improved the accuracy of current ANN models by using increased weight resolutions of memristive devices, combining two or more in-series RRAM cells, integrated in the back end of line (BEOL) of the CMOS process. Based on system level simulations, 1T2R devices were fabricated in IHP's 130nm SiGe:BiCMOS technology node, demonstrating an increased number of states. We achieved an increase in weight resolution from 3 bit in 1T1R cells to 6.5 bit in our 1T2R cell. The experimental data of 1T2R devices gives indications for the performance and energy-efficiency improvement in 1TNR arrays for ANN applications.
更多查看译文
关键词
1T1R,1TNR,ANN,In-Memory Computing,Memristive Devices,Neuromorphic Computing,RRAM
AI 理解论文
溯源树
样例
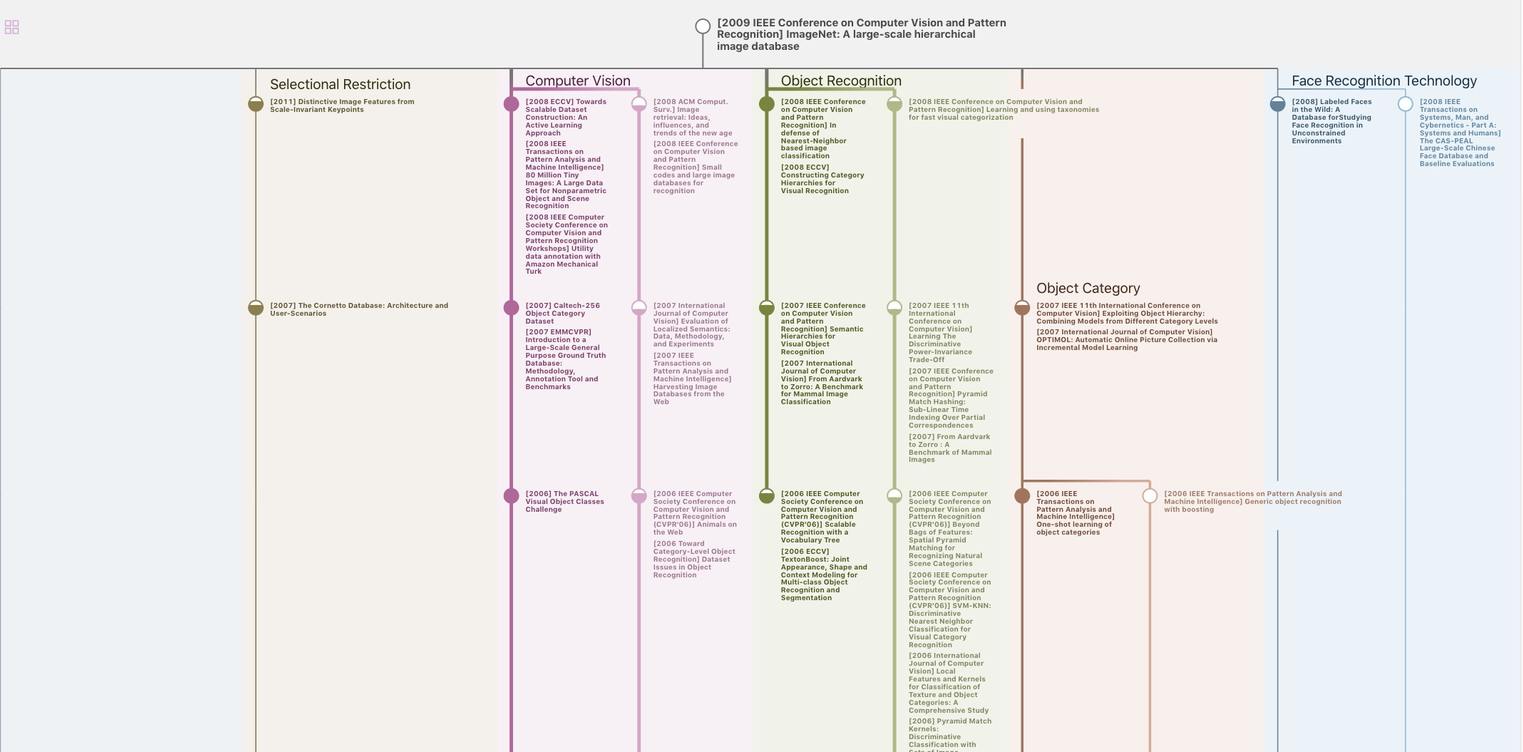
生成溯源树,研究论文发展脉络
Chat Paper
正在生成论文摘要