Incorporating Neuro-Inspired Adaptability for Continual Learning in Artificial Intelligence
Nat. Mac. Intell.(2023)
摘要
Continual learning aims to empower artificial intelligence (AI) with strong adaptability to the real world. For this purpose, a desirable solution should properly balance memory stability with learning plasticity, and acquire sufficient compatibility to capture the observed distributions. Existing advances mainly focus on preserving memory stability to overcome catastrophic forgetting, but remain difficult to flexibly accommodate incremental changes as biological intelligence (BI) does. By modeling a robust Drosophila learning system that actively regulates forgetting with multiple learning modules, here we propose a generic approach that appropriately attenuates old memories in parameter distributions to improve learning plasticity, and accordingly coordinates a multi-learner architecture to ensure solution compatibility. Through extensive theoretical and empirical validation, our approach not only clearly enhances the performance of continual learning, especially over synaptic regularization methods in task-incremental settings, but also potentially advances the understanding of neurological adaptive mechanisms, serving as a novel paradigm to progress AI and BI together.
更多查看译文
关键词
continual learning,artificial intelligence,neuro-inspired
AI 理解论文
溯源树
样例
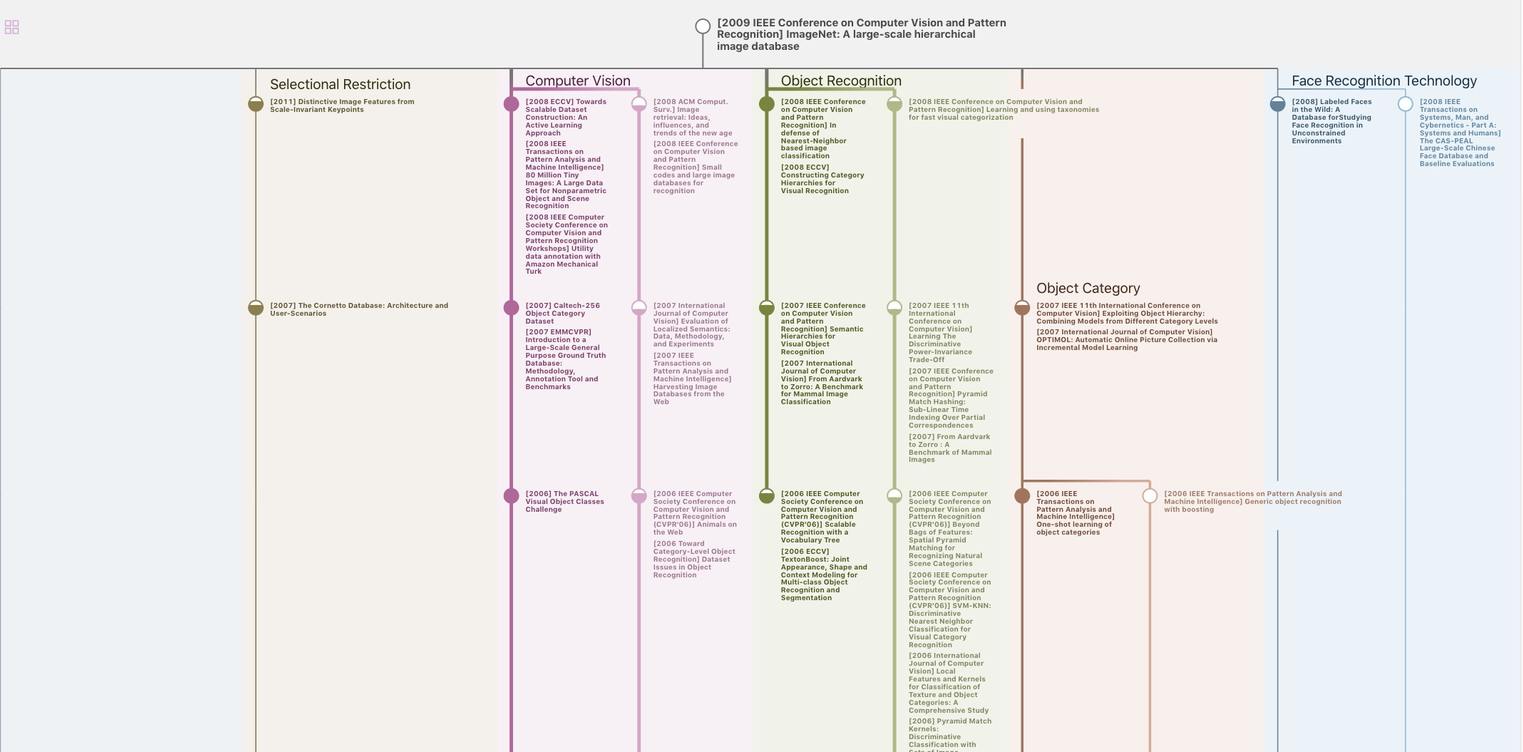
生成溯源树,研究论文发展脉络
Chat Paper
正在生成论文摘要