Adfa: attention-augmented differentiable top-k feature adaptation for unsupervised medical anomaly detection
2023 IEEE INTERNATIONAL CONFERENCE ON IMAGE PROCESSING, ICIP(2023)
摘要
The scarcity of annotated data, particularly for rare diseases, limits the variability of training data and the range of detectable lesions, presenting a significant challenge for supervised anomaly detection in medical imaging. To solve this problem, we propose a novel unsupervised method for medical image anomaly detection: Attention-Augmented Differentiable top-k Feature Adaptation (ADFA). The method utilizes Wide-ResNet50-2 (WR50) network pre-trained on ImageNet to extract initial feature representations. To reduce the channel dimensionality while preserving relevant channel information, we employ an attention-augmented patch descriptor on the extracted features. We then apply differentiable top-k feature adaptation to train the patch descriptor, mapping the extracted feature representations to a new vector space, enabling effective detection of anomalies. Experiments show that ADFA outperforms state-of-the-art (SOTA) methods on multiple challenging medical image datasets, confirming its effectiveness in medical anomaly detection.
更多查看译文
关键词
anomaly detection,medical image,unsupervised learning,attention mechanism,feature adaptation
AI 理解论文
溯源树
样例
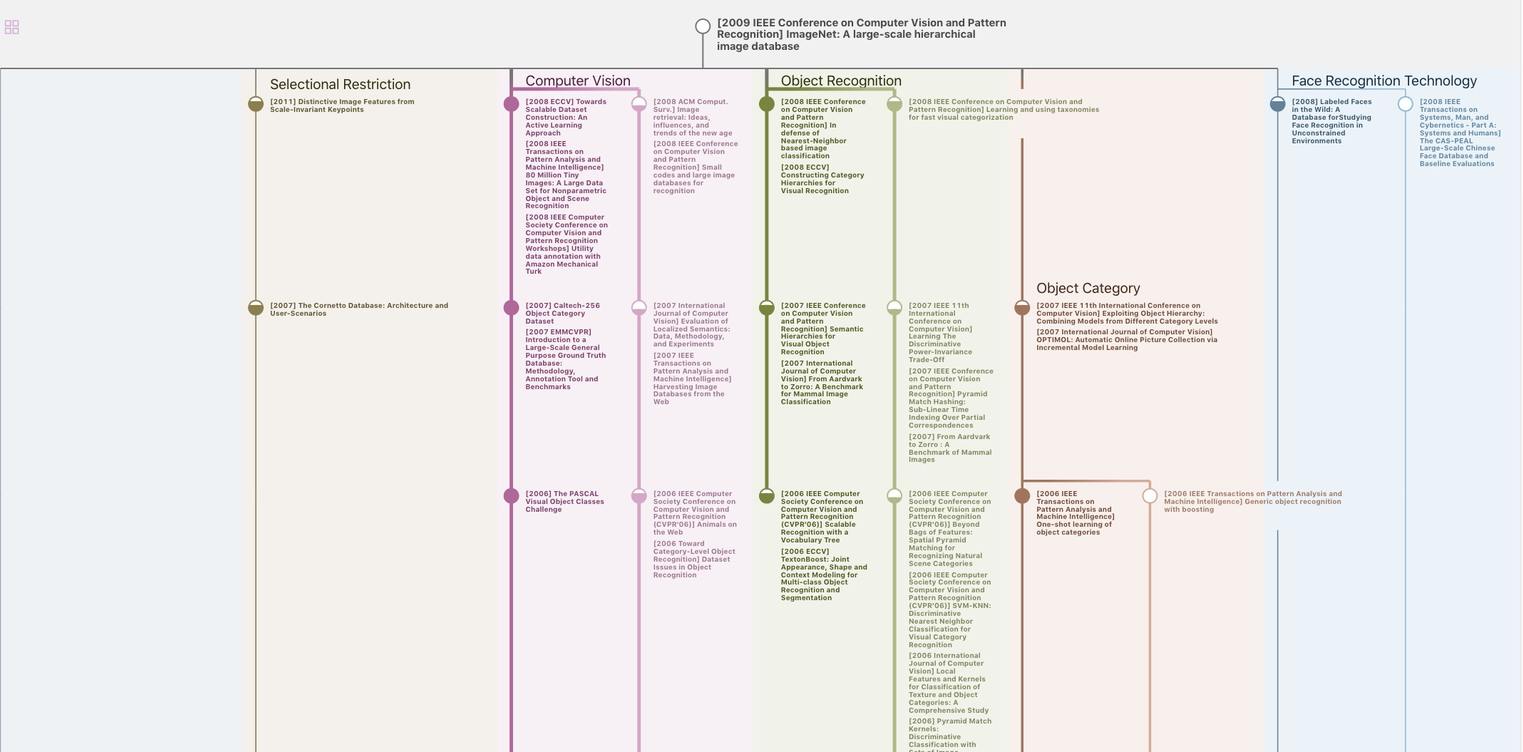
生成溯源树,研究论文发展脉络
Chat Paper
正在生成论文摘要