Elucidating the Exposure Bias in Diffusion Models
ICLR 2024(2023)
摘要
Diffusion models have demonstrated impressive generative capabilities, but their 'exposure bias' problem, described as the input mismatch between training and sampling, lacks in-depth exploration. In this paper, we systematically investigate the exposure bias problem in diffusion models by first analytically modelling the sampling distribution, based on which we then attribute the prediction error at each sampling step as the root cause of the exposure bias issue. Furthermore, we discuss potential solutions to this issue and propose an intuitive metric for it. Along with the elucidation of exposure bias, we propose a simple, yet effective, training-free method called Epsilon Scaling to alleviate the exposure bias. We show that Epsilon Scaling explicitly moves the sampling trajectory closer to the vector field learned in the training phase by scaling down the network output (Epsilon), mitigating the input mismatch between training and sampling. Experiments on various diffusion frameworks (ADM, DDPM/DDIM, LDM), unconditional and conditional settings, and deterministic vs. stochastic sampling verify the effectiveness of our method.
更多查看译文
关键词
Generative models,Diffusion models
AI 理解论文
溯源树
样例
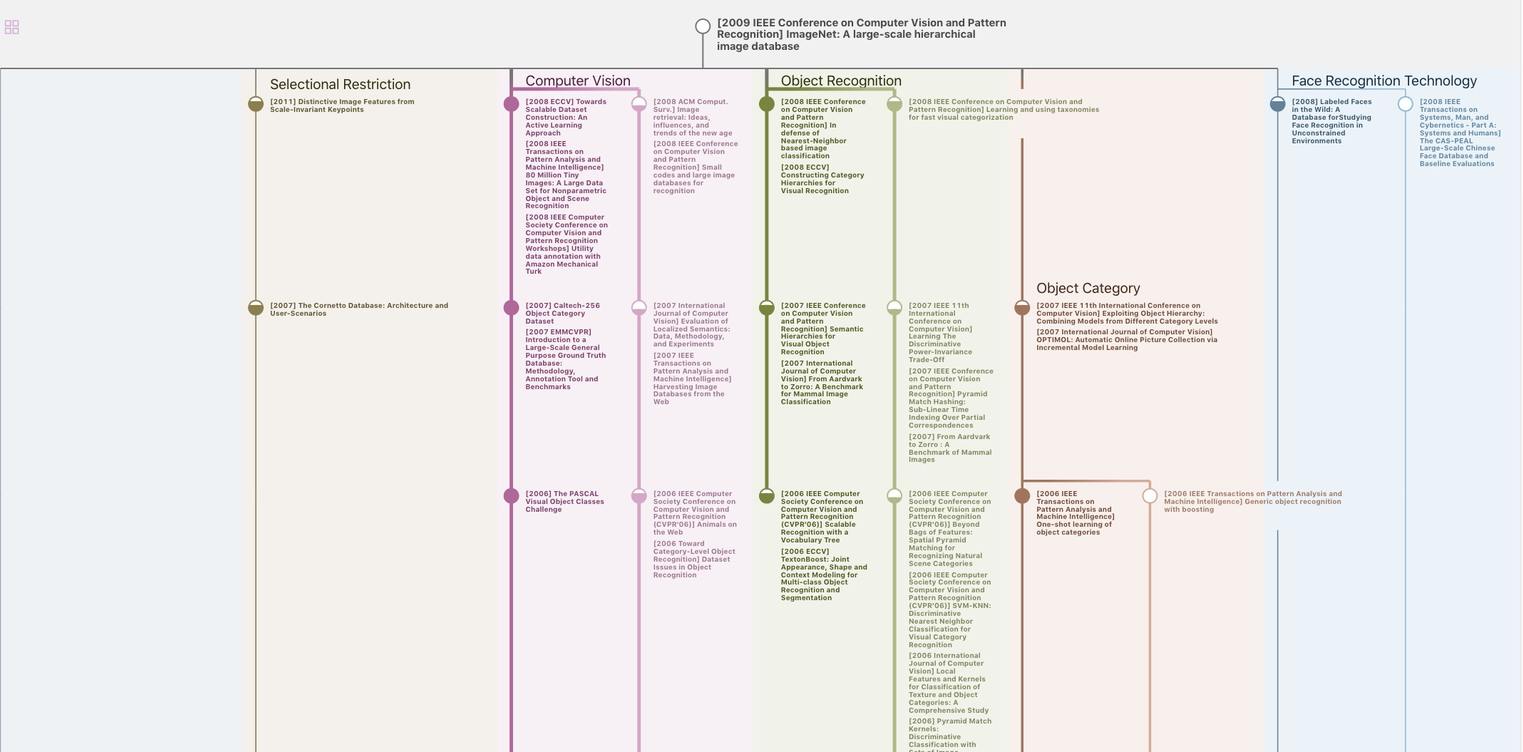
生成溯源树,研究论文发展脉络
Chat Paper
正在生成论文摘要