Few-Shot Bayesian Performance Modeling for Silicon Photonic Devices Under Process Variation
Journal of Lightwave Technology(2023)
摘要
We develop and apply a Bayesian linear regression technique for few-shot performance modeling of silicon photonic devices under process variation. The key contribution is to leverage information from low-fidelity simulations which are cheap to obtain, and to encode that information into the prior distribution under a Bayesian framework. We then evaluate the maximum-a-posteriori (MAP) estimator according to Bayes' theorem. Our numerical experiments on a rectangular waveguide and a Y-branch show that compared to linear regression, ridge regression, and a multi-layer feedforward neural network, this approach can achieve 7x smaller error given the same number of samples, or reduce running time by 2.4x to reach the same accuracy. Most importantly, we demonstrate that with only 10 expensive high-fidelity simulations and a small number of cheap low-fidelity simulations, our approach can obtain a model with good performance.
更多查看译文
关键词
Bayesian inference, process variation, performance modeling
AI 理解论文
溯源树
样例
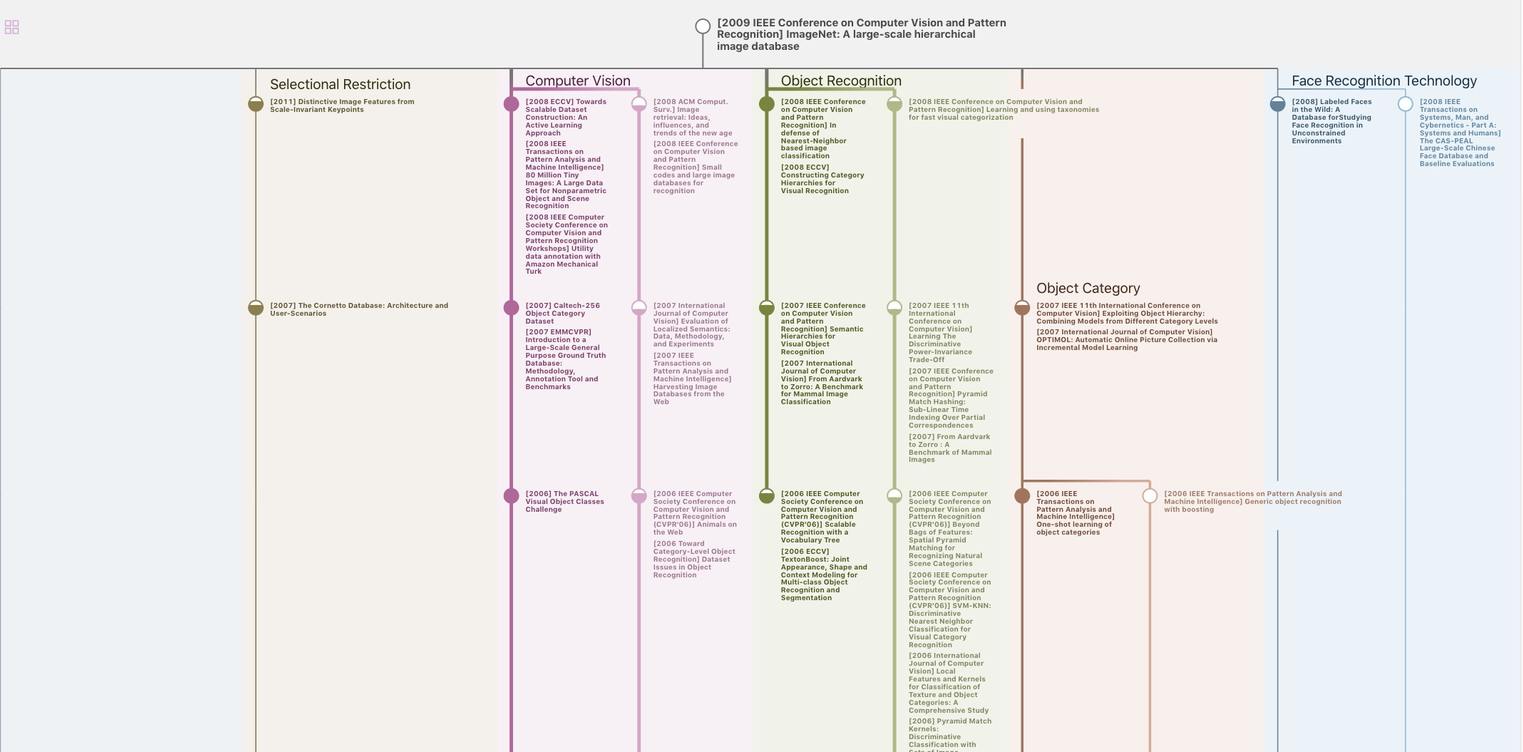
生成溯源树,研究论文发展脉络
Chat Paper
正在生成论文摘要