Efficient Generation of Pretraining Samples for Developing a Deep Learning Brain Injury Model via Transfer Learning
Annals of biomedical engineering(2023)
摘要
The large amount of training samples required to develop a deep learning brain injury model demands enormous computational resources. Here, we study how a transformer neural network (TNN) of high accuracy can be used to efficiently generate pretraining samples for a convolutional neural network (CNN) brain injury model to reduce computational cost. The samples use synthetic impacts emulating real-world events or augmented impacts generated from limited measured impacts. First, we verify that the TNN remains highly accurate for the two impact types ( N = 100 each; R^2 of 0.948–0.967 with root mean squared error, RMSE, 0.01, for voxelized peak strains). The TNN-estimated samples (1000–5000 for each data type) are then used to pretrain a CNN, which is further finetuned using directly simulated training samples (250–5000). An independent measured impact dataset considered of complete capture of impact event is used to assess estimation accuracy ( N = 191). We find that pretraining can significantly improve CNN accuracy via transfer learning compared to a baseline CNN without pretraining. It is most effective when the finetuning dataset is relatively small (e.g., 2000–4000 pretraining synthetic or augmented samples improves success rate from 0.72 to 0.81 with 500 finetuning samples). When finetuning samples reach 3000 or more, no obvious improvement occurs from pretraining. These results support using the TNN to rapidly generate pretraining samples to facilitate a more efficient training strategy for future deep learning brain models, by limiting the number of costly direct simulations from an alternative baseline model. This study could contribute to a wider adoption of deep learning brain injury models for large-scale predictive modeling and ultimately, enhancing safety protocols and protective equipment.
更多查看译文
关键词
Synthetic data,Transfer learning,Convolutional neural network,Transformer neural network,Traumatic brain injury
AI 理解论文
溯源树
样例
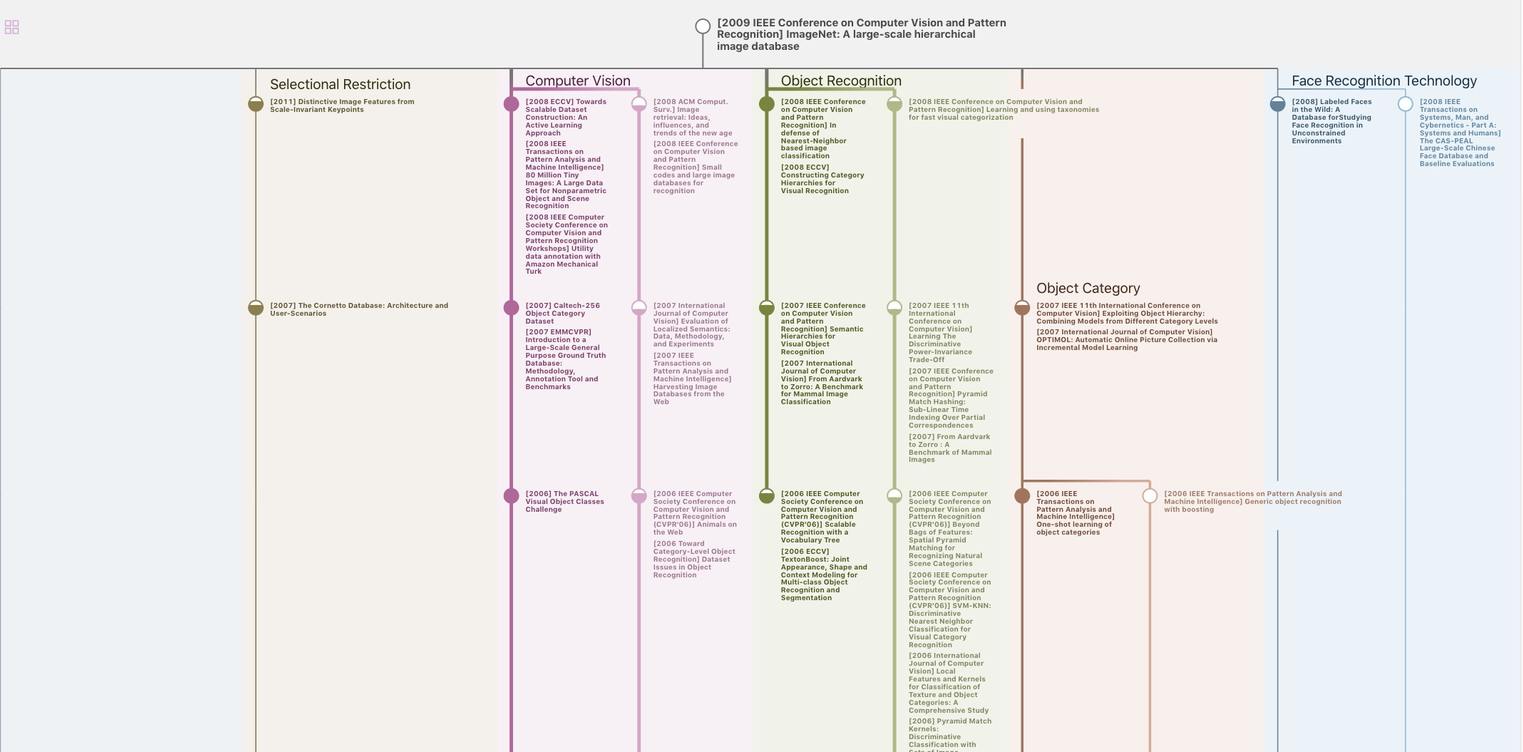
生成溯源树,研究论文发展脉络
Chat Paper
正在生成论文摘要