Atomic Positional Embedding-Based Transformer Model for Predicting the Density of States of Crystalline Materials
The journal of physical chemistry letters(2023)
摘要
The rapid advancement of machine learning has revolutionized quite a few science fields, leading to a surge in the development of highly efficient and accurate materials discovery methods. Recently, predictions of multiple related properties have received attention, with a particular emphasis on spectral properties, where the electronic density of states (DOS) stands out as the fundamental data with enormous potential to advance our understanding of crystalline materials. Leveraging the power of the Transformer framework, we introduce an Atomic Positional Embedding-Based Transformer (APET), which surpasses existing state-of-the-art models for predicting ab initio DOS. APET utilizes atomic periodical positions as its positional embedding, which incorporates all of the structural information in a crystal, providing a more complete and accurate representation. Furthermore, the interpretability of APET enables us to discover the underlying physical properties of materials with greater precision and accuracy.
更多查看译文
关键词
transformer,embedding-based
AI 理解论文
溯源树
样例
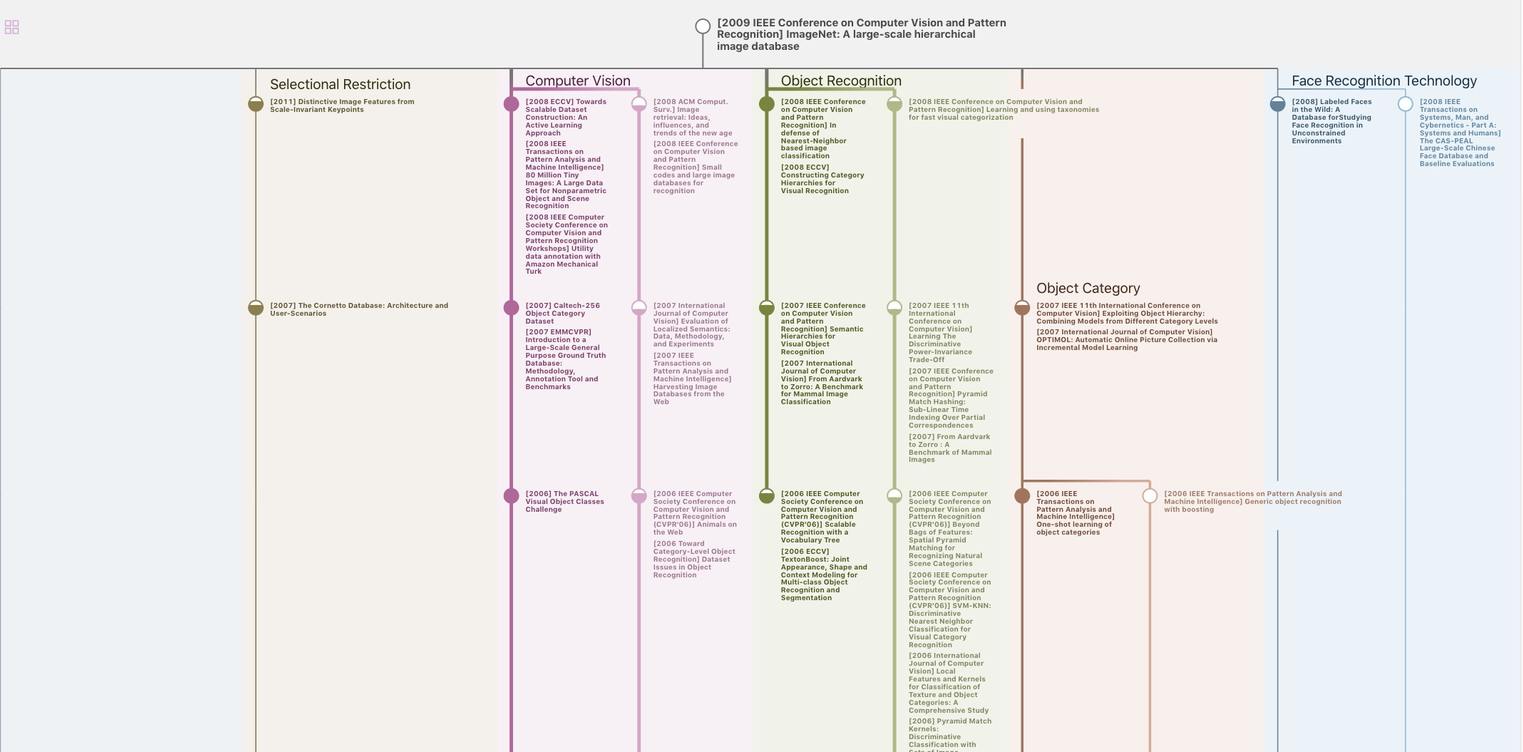
生成溯源树,研究论文发展脉络
Chat Paper
正在生成论文摘要