Self-Attention Metric Learning Based on Multiscale Feature Fusion for Few-Shot Fault Diagnosis
IEEE SENSORS JOURNAL(2023)
摘要
The intelligent diagnosis model based on deep learning method can effectively and accurately diagnose the health state of bearings widely used in various mechanical equipment. However, in engineering practice, it is difficult to obtain sufficient labeled fault data, which would reduce the diagnosis performance. In order to solve the above problem, this article proposes a self-attention metric learning based on multiscale feature fusion to classify the bearing fault with few shots. The proposed few-shot intelligent diagnosis model mainly contains the feature extraction module and metric learning module. First, the vibration data need to be combined in pairs to form sample pairs, which are input into the feature extraction module based on multiscale feature fusion (MSFF). This input approach can increase the learning tasks so as to alleviate the problem of insufficient training samples. Then, the extracted features are concatenated and input into the metric learning module whose learning ability is improved by adding the self-attention (SA) network, and the input features are eventually converted into the similarity. Finally, the fault type can be identified according to the maximum similarity value between the test sample and samples with different labels. Three experiment cases are conducted to validate the performance of proposed intelligent diagnosis model. The experimental results show that the proposed model can accurately classify the bearing fault with few-shot case. Additionally, the comparison experiments with the traditional models have also validated the advantage of the proposed intelligent diagnosis model for few-shot case.
更多查看译文
关键词
Deep learning,few-shot fault diagnosis,metric learning,multiscale feature fusion (MSFF),self-attention (SA) mechanism
AI 理解论文
溯源树
样例
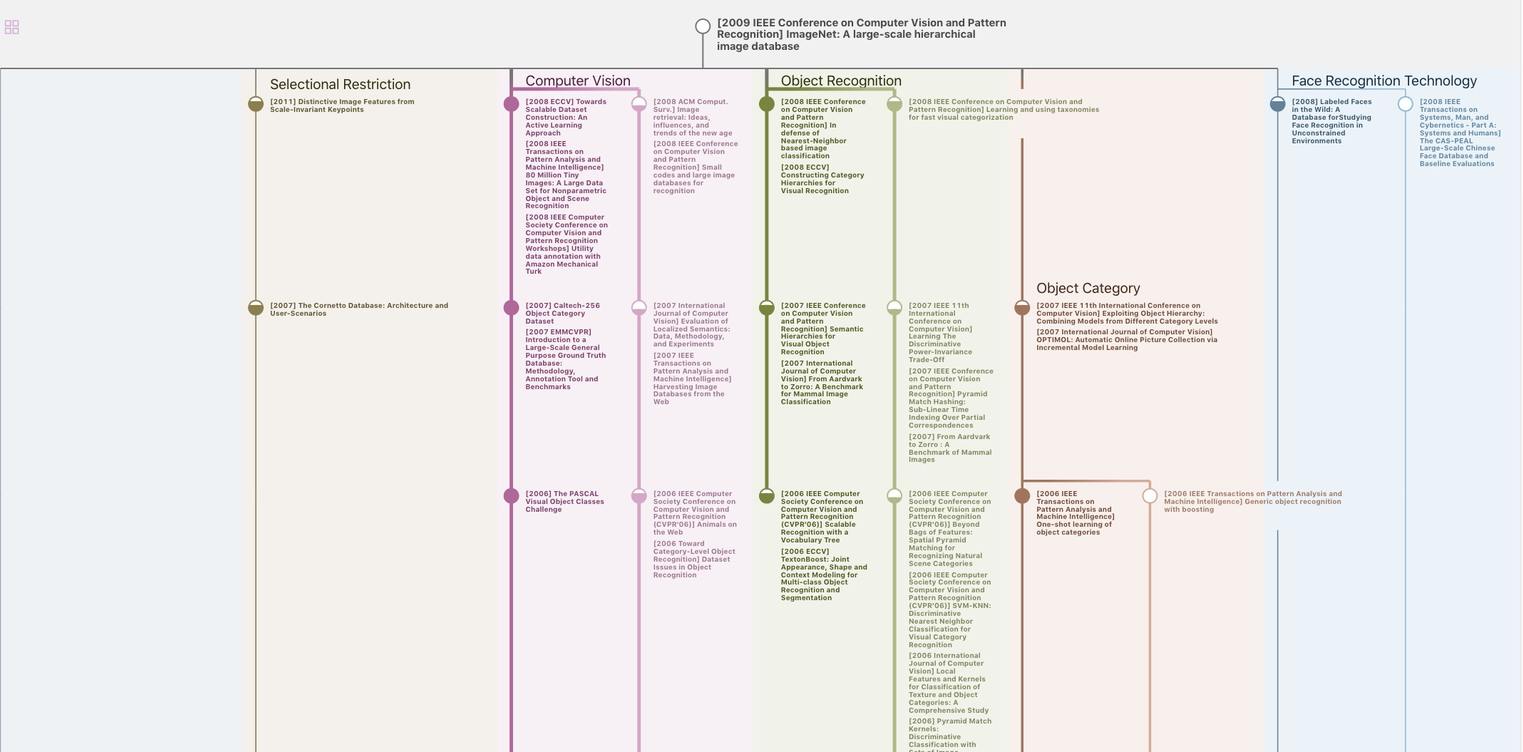
生成溯源树,研究论文发展脉络
Chat Paper
正在生成论文摘要