Control of soft robots with inertial dynamics
SCIENCE ROBOTICS(2023)
摘要
Soft robots promise improved safety and capability over rigid robots when deployed near humans or in complex, delicate, and dynamic environments. However, infinite degrees of freedom and the potential for highly nonlinear dynamics severely complicate their modeling and control. Analytical and machine learning methodologies have been applied to model soft robots but with constraints: quasi-static motions, quasi-linear deflections, or both. Here, we advance the modeling and control of soft robots into the inertial, nonlinear regime. We controlled motions of a soft, continuum arm with velocities 10 times larger and accelerations 40 times larger than those of previous work and did so for high-deflection shapes with more than 110 degrees of curvature. We leveraged a data-driven learning approach for modeling, based on Koopman operator theory, and we introduce the concept of the static Koopman operator as a pregain term in optimal control. Our approach is rapid, requiring less than 5 min of training; is computationally low cost, requiring as little as 0.5 s to build the model; and is design agnostic, learning and accurately controlling two morphologically different soft robots. This work advances rapid modeling and control for soft robots from the realm of quasi-static to inertial, laying the groundwork for the next generation of compliant and highly dynamic robots.
更多查看译文
关键词
soft robots,inertial dynamics
AI 理解论文
溯源树
样例
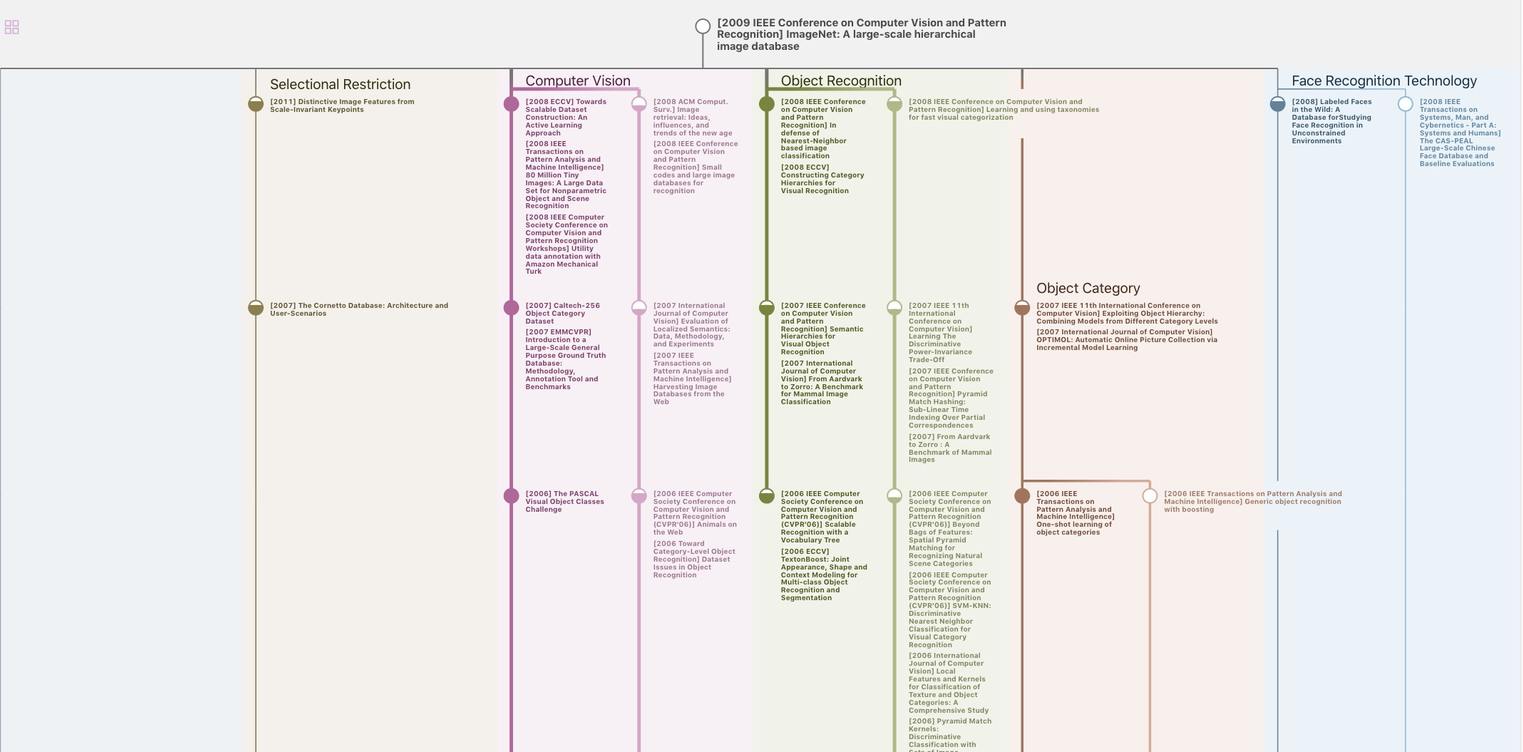
生成溯源树,研究论文发展脉络
Chat Paper
正在生成论文摘要