Marker-Free Isoelectric Focusing Patterns for Identification of Meat Samples via Deep Learning.
Analytical chemistry(2023)
摘要
Isoelectric focusing (IEF) is a powerful tool for resolving complex protein samples, which generates IEF patterns consisting of multiplex analyte bands. However, the interpretation of IEF patterns requires the careful selection of isoelectric point (pI) markers for profiling the pH gradient and a trivial process of pI labeling, resulting in low IEF efficiency. Here, we for the first time proposed a marker-free IEF method for the efficient and accurate classification of IEF patterns by using a convolutional neural network (CNN) model. To verify our method, we identified 21 meat samples whose IEF patterns comprised different bands of meat hemoglobin, myoglobin, and their oxygen-binding variants but no pI marker. Thanks to the high throughput and short assay time of the microstrip IEF, we efficiently collected 1449 IEF patterns to construct the data set for model training. Despite the absence of pI markers, we experimentally introduced the severe pH gradient drift into 189 IEF patterns in the data set, thereby omitting the need for profiling the pH gradient. To enhance the model robustness, we further employed data augmentation during the model training to mimic pH gradient drift. With the advantages of simple preprocessing, a rapid inference of 50 ms, and a high accuracy of 97.1%, the CNN model outperformed the traditional algorithm for simultaneously identifying meat species and cuts of meat of 105 IEF patterns, suggesting its great potential of being combined with microstrip IEF for large-scale IEF analyses of complicated protein samples.
更多查看译文
关键词
meat samples,deep learning,marker-free
AI 理解论文
溯源树
样例
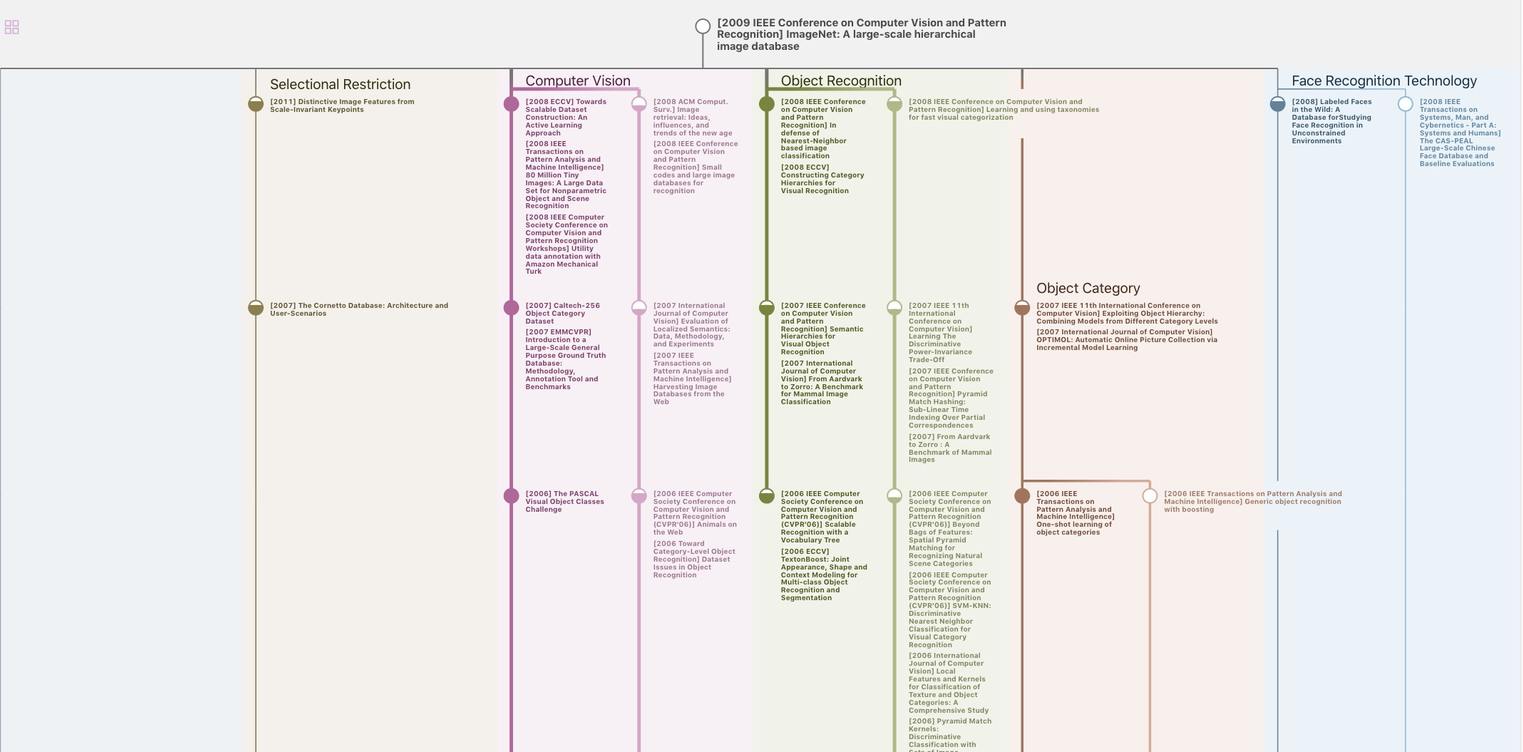
生成溯源树,研究论文发展脉络
Chat Paper
正在生成论文摘要