Time-Series Forecasting to Fill Missing Data in IoT Sensor Data
IEEE Sensors Letters(2023)
摘要
Internet of Things (IoT) devices have been deployed in different industrial applications due to their feasible implementation thanks to the modern toolsets and many sensor brands to select the adequate sensor. However, even when their main task is collecting data, there are challenges, such as working in unpredictable environments, which can affect the communication channel and sensors’ functionalities, which could cause the loss of information. On the other hand, statistical and deep-learning-based forecasting methods can fill in missing data. In this letter, we investigate those forecasting algorithms to select the best one for different data measurements in a real-world setting, such as temperature, humidity, and CO
2
. Based on our results, the Holt–Winters methods were selected to forecast the temperature and CO
2
, and long short-term memory was used to forecast humidity. These models were then trained in the edge-scale servers to define their computational requirements and feasibility to be exported to a microcontroller.
更多查看译文
关键词
Sensor applications,artificial intelligence,forecasting methods,industrial Internet of Things (IoT),missing value filling,wireless sensor network
AI 理解论文
溯源树
样例
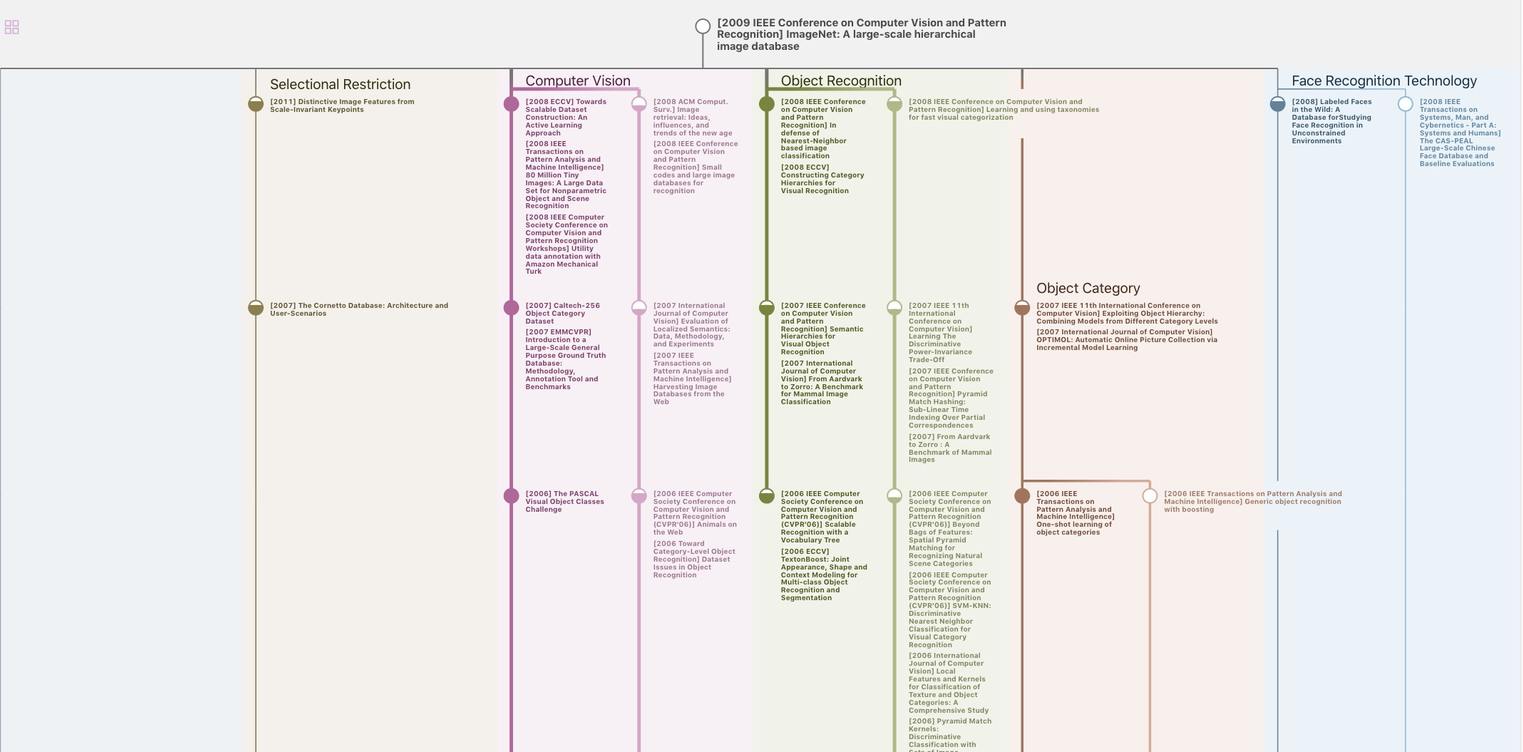
生成溯源树,研究论文发展脉络
Chat Paper
正在生成论文摘要