Heuristic-based Resource Allocation for Cloud-native Machine Learning Workloads
International Workshop on Ant Colony Optimization and Swarm Intelligence(2022)
摘要
As machine learning workloads become computationally demanding, there is an increased focus on distributed machine learning to train and deploy models across multiple machines in a cloud-native cluster. However, optimizing a machine learning model’s lifecycle to facilitate efficient resource utilization is still an active area of research. The approach typically involves a manual effort to partition the models into distinct layers and decide how to store these distinct layers on a distributed computing framework. However, distributing distinct layers across nodes can induce a network latency bottleneck in the machine learning pipeline. Further, the above process becomes more inefficient as models become increasingly complex. In this paper, we present a heuristic-based approach to distributed model training. Further, we analyze the resource utilization metrics from a sample machine learning pipeline deployed on a KubeFlow MLOps framework testbed.
更多查看译文
关键词
Cloud-native Infrastructure,MLOps,Resource Allocation
AI 理解论文
溯源树
样例
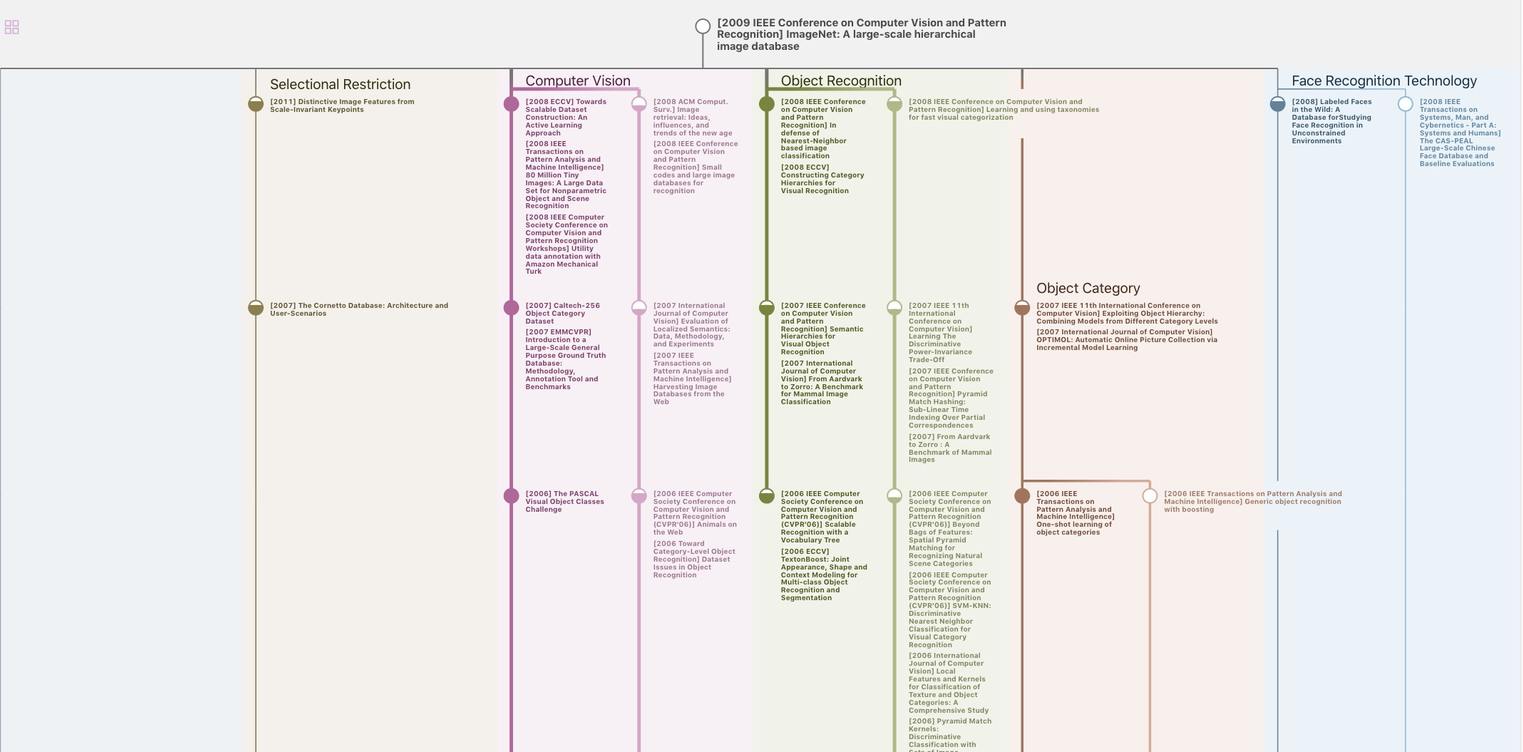
生成溯源树,研究论文发展脉络
Chat Paper
正在生成论文摘要