scGAMNN: Graph Antoencoder-Based Single-Cell RNA Sequencing Data Integration Algorithm Using Mutual Nearest Neighbors
IEEE JOURNAL OF BIOMEDICAL AND HEALTH INFORMATICS(2023)
摘要
It is critical to correctly assemble high-dimensional single-cell RNA sequencing (scRNA-seq) datasets and downscale them for downstream analysis. However, given the complex relationships between cells, it remains a challenge to simultaneously eliminate batch effects between datasets and maintain the topology between cells within each dataset. Here, we propose scGAMNN, a deep learning model based on graph autoencoder, to simultaneously achieve batch correction and topology-preserving dimensionality reduction. The low-dimensional integrated data obtained by scGAMNN can be used for visualization, clustering and trajectory inference.By comparing it with the other five methods, multiple tasks show that scGAMNN consistently has comparable data integration performance in clustering and trajectory conservation.
更多查看译文
关键词
Batch correction,deep learning,graph autoencoder,mutual nearest neighbor,scRNA-seq data integration
AI 理解论文
溯源树
样例
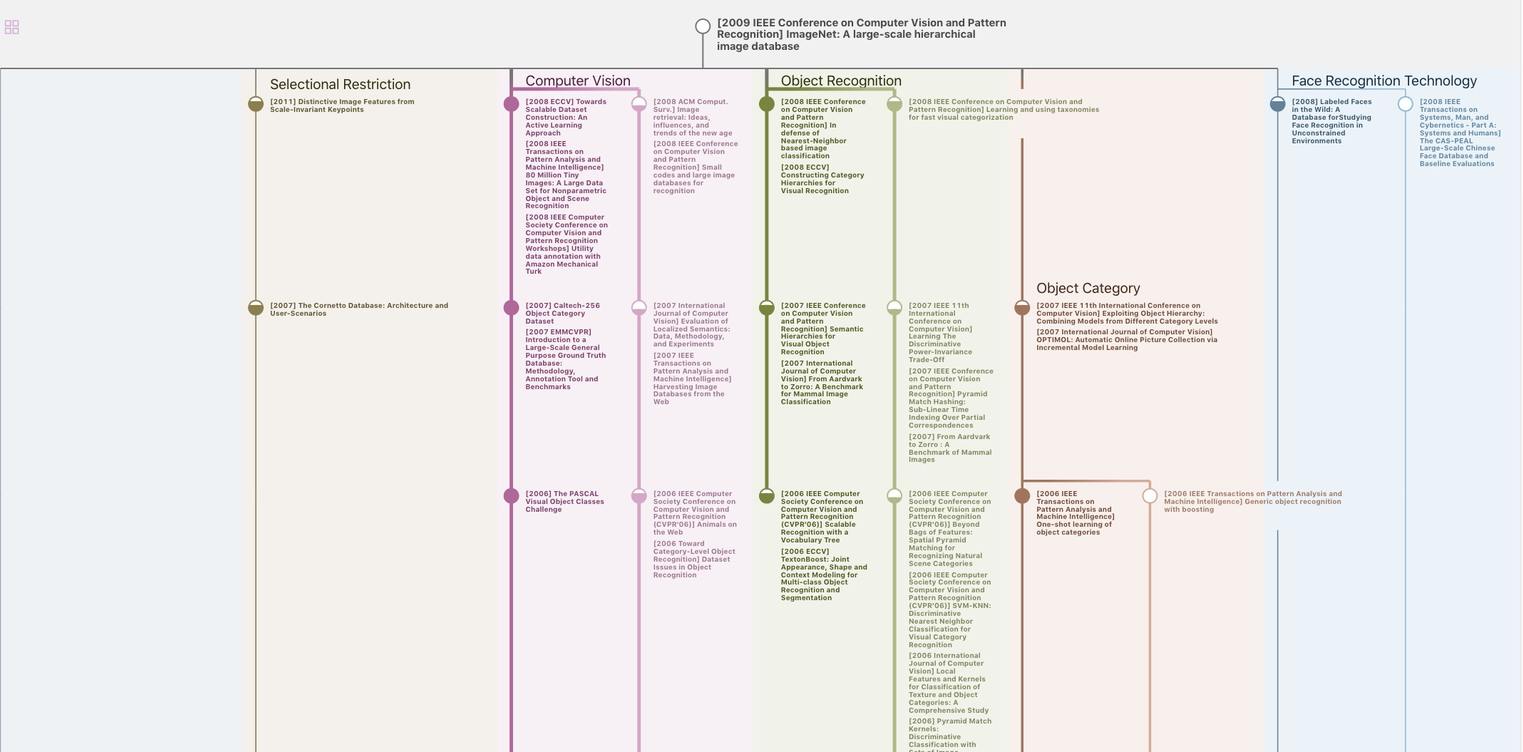
生成溯源树,研究论文发展脉络
Chat Paper
正在生成论文摘要