AI-triaged 3D pathology to improve detection of esophageal neoplasia while reducing pathologist workloads.
Modern pathology : an official journal of the United States and Canadian Academy of Pathology, Inc(2023)
摘要
Early detection of esophageal neoplasia via evaluation of endoscopic surveillance biopsies is key to maximizing survival for patients with Barrett's esophagus, but is hampered by the sampling limitations of conventional slide-based histopathology. Comprehensive evaluation of whole biopsies with 3D pathology may improve early detection of malignancies, but large 3D pathology datasets are tedious for pathologists to analyze. Here we present a deep learning-based method to automatically identify the most-critical 2D image sections within 3D pathology datasets for pathologists to review. Our method first generates a 3D heatmap of neoplastic risk for each biopsy, then classifies all 2D image sections within the 3D dataset in order of neoplastic risk. In a clinical validation study, we diagnose esophageal biopsies with AI-triaged 3D pathology (3 images per biopsy) vs. standard slide-based histopathology (16 images per biopsy) and show that our method improves detection sensitivity while reducing pathologist workloads.
更多查看译文
关键词
3D pathology, light-sheet microscopy, deep learning, Barrett's esophagus, dysplasia, esophageal adenocarcinoma
AI 理解论文
溯源树
样例
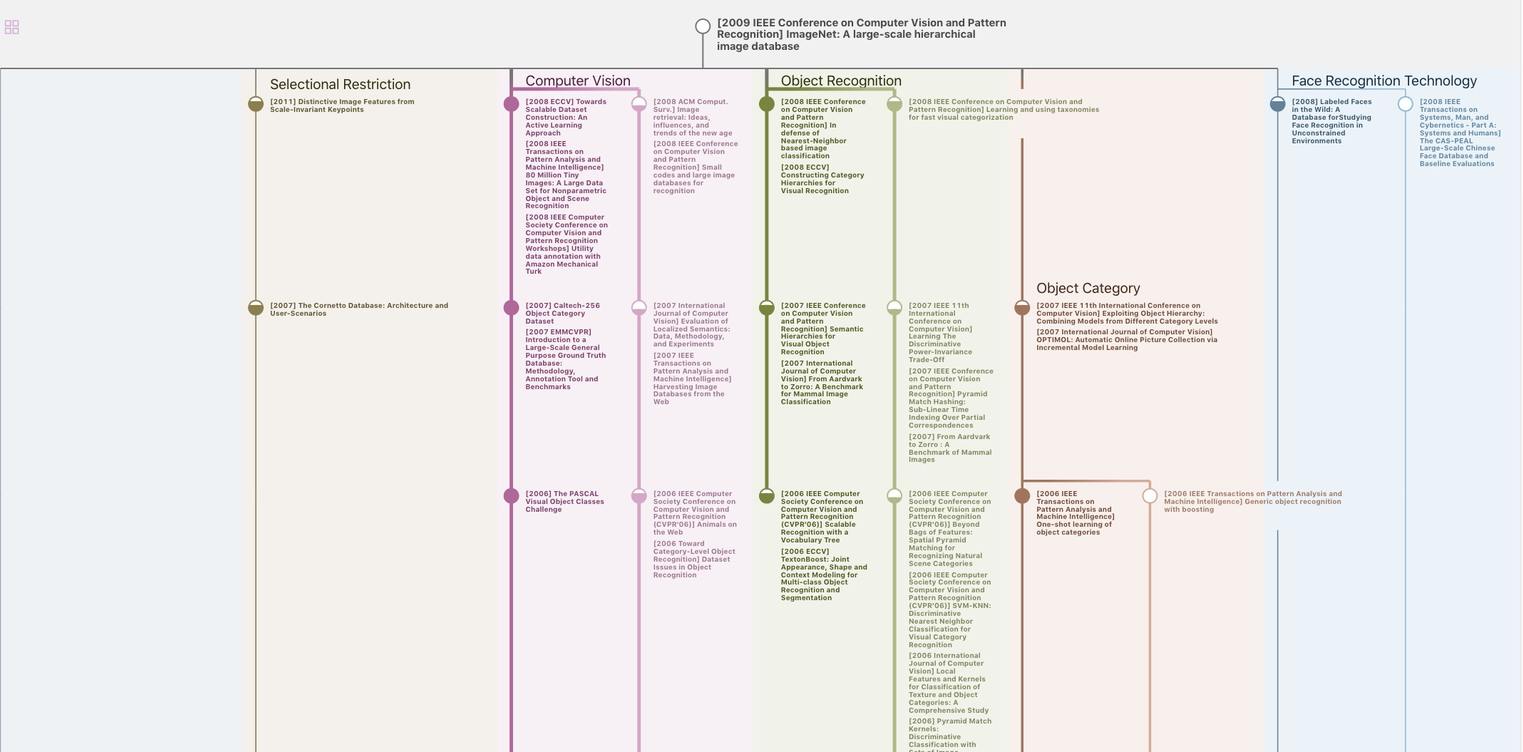
生成溯源树,研究论文发展脉络
Chat Paper
正在生成论文摘要