Consensus-Based Distributed Kernel One-class Support Vector Machine for Anomaly Detection.
IJCNN(2023)
摘要
One-class support vector machine (OCSVM) is one of the most widely used methods for learning from imbalanced data and has been successfully applied to numerous tasks such as anomaly detection. However, the study on decentralized OCSVM is currently limited to linear cases. The main challenge is how to communicate the non-parametric and local-data-dependent decision functions between neighboring nodes. To tackle it, this paper proposes a projection consensus constraint to formulate a decentralized OCSVM, where local solutions are assumed to be the projection of the global optimum on local reproducing kernel Hilbert spaces. A fast non-parametric solving algorithm is then designed based on alternating direction method of multipliers. Experiments on real-world anomaly datasets indicate that our method outperforms the existing distributed OCSVM methods while reducing the communication cost.
更多查看译文
关键词
alternating direction method of multipliers,anomaly detection,consensus-based distributed kernel one-class support vector machine,decentralized OCSVM,kernel Hilbert spaces,linear cases,local-data-dependent decision,nonparametric solving algorithm,projection consensus constraint
AI 理解论文
溯源树
样例
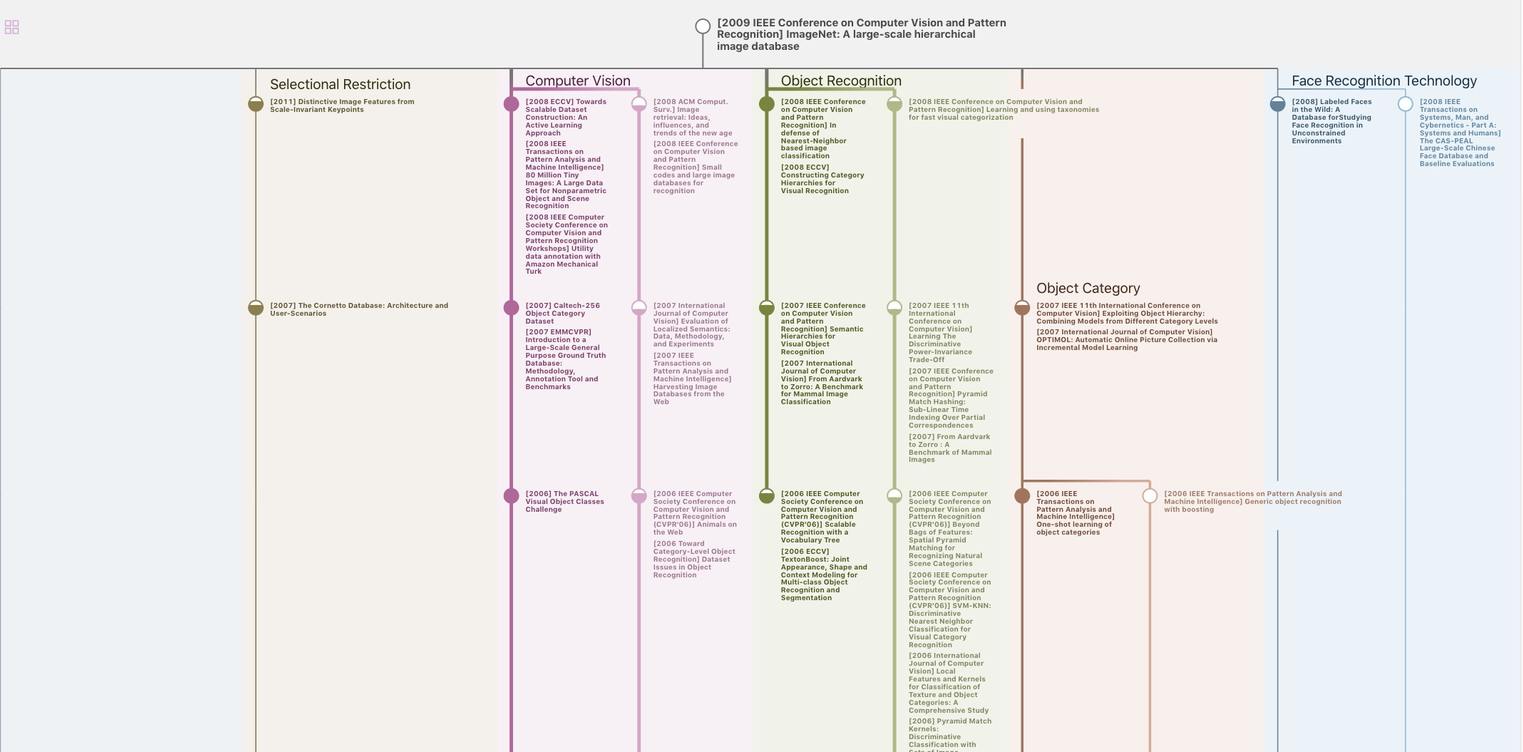
生成溯源树,研究论文发展脉络
Chat Paper
正在生成论文摘要