An Improved Trust-Region Method for Off-Policy Deep Reinforcement Learning.
IJCNN(2023)
摘要
Reinforcement learning (RL) is a powerful tool for training agents to interact with complex environments. In particular, trust-region methods are widely used for policy optimization in model-free RL. However, these methods suffer from high sample complexity due to their on-policy nature, which requires interactions with the environment for each update. To address this issue, off-policy trust-region methods have been proposed, but they have shown limited success in high-dimensional continuous control problems compared to other offpolicy DRL methods. To improve the performance and sample efficiency of trust-region policy optimization, we propose an offpolicy trust-region RL algorithm. Our algorithm is based on a theoretical result on a closed-form solution to trust-region policy optimization and is effective in optimizing complex nonlinear policies. We demonstrate the superiority of our algorithm over prior trust-region DRL methods and show that it achieves excellent performance on a range of continuous control tasks in the Multi-Joint dynamics with Contact (MuJoCo) environment, comparable to state-of-the-art off-policy algorithms.
更多查看译文
关键词
closed-form solution,complex nonlinear policies,high-dimensional continuous control problems,model-free RL,MuJoCo environment,multijoint dynamics with contact environment,off-policy deep reinforcement learning,off-policy DRL methods,off-policy trust-region methods,off-policy trust-region RL algorithm,prior trust-region DRL methods,sample complexity,trust-region policy optimization
AI 理解论文
溯源树
样例
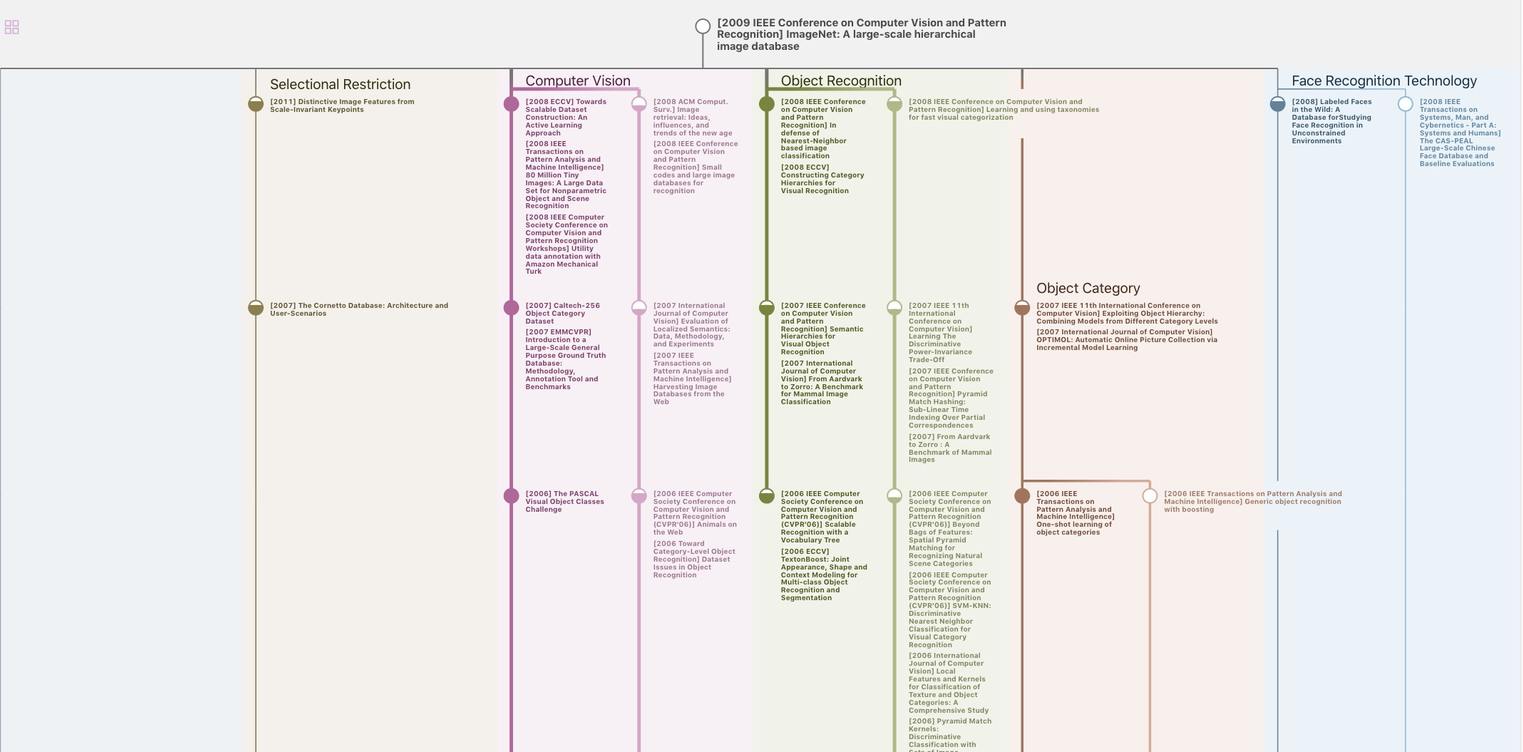
生成溯源树,研究论文发展脉络
Chat Paper
正在生成论文摘要