Transformed-*: A domain-incremental lifelong learning scenario generation framework.
IJCNN(2023)
摘要
Lifelong learning is becoming a popular trend in modern machine learning research. Domain-incremental scenarios are particularly relevant since they closely reflects real-world characteristics. However, one open challenge is the ability to devise scenarios that entail the inherent unpredictability and complexities of domains still unexplored in lifelong learning. To tackle this issue, we propose a framework for domainincremental scenario generation. The framework enables users to create lifelong learning scenarios using any image dataset, and leveraging a fully customizable pool of transformation functions. We devise an algorithm and criteria that iteratively guide users in evaluating the inclusion of candidate transformation functions to the scenario and in making this decision based on desired outcomes. Experimental results with common lifelong learning strategies and benchmark datasets show that our framework is highly flexible since it allows tweaking complexities and challenges incorporated in generated scenarios. Furthermore, experimental results show that there is a gap between state-ofthe-art learning strategies and a proposed upper bound to be exploited in the design of future learning strategies.
更多查看译文
关键词
lifelong learning, lifelong image classification, domain-incremental
AI 理解论文
溯源树
样例
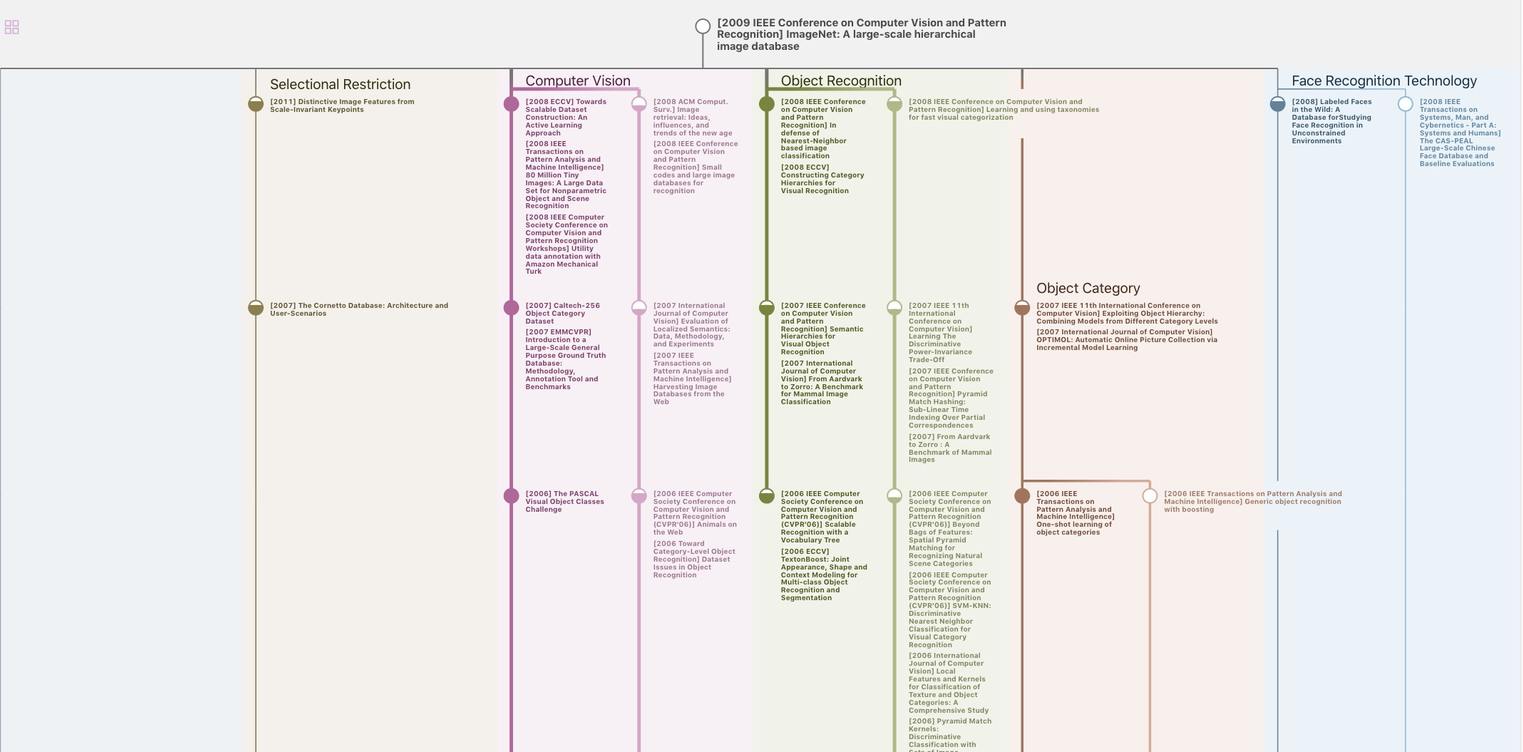
生成溯源树,研究论文发展脉络
Chat Paper
正在生成论文摘要