QVDDPG: QV Learning with Balanced Constraint in Actor-Critic Framework.
IJCNN(2023)
摘要
Actor-critic framework has achieved tremendous success in a great many of decision-making scenarios. Nevertheless, when updating the value of new states and actions in the long-term scene, these methods suffer from misestimate problem and gradient variance problem, significantly reducing convergence speed and robustness of the policy. These problems severely limit the application scope of these methods. In this paper, we first proposed QVDDPG, a deep RL algorithm based on the iterative target value update process. The QV learning method alleviates the problem of misestimate by making use of the guidance of Q value and the fast convergence of V value, thus accelerating the convergence speed. In addition, the actor utilizes a constrained balanced gradient and establishes a hidden state for the continuous action space network for the sake of robustness of the model. We give the update relation among the value functions and the constraint conditions of gradient estimation. We measure our method on the PyBullet and achieved state-of-the-art performance. Moreover, we demonstrate that, our method has higher robustness and convergence speed across different tasks compared to other algorithms.
更多查看译文
关键词
deep learning,reinforcement learning
AI 理解论文
溯源树
样例
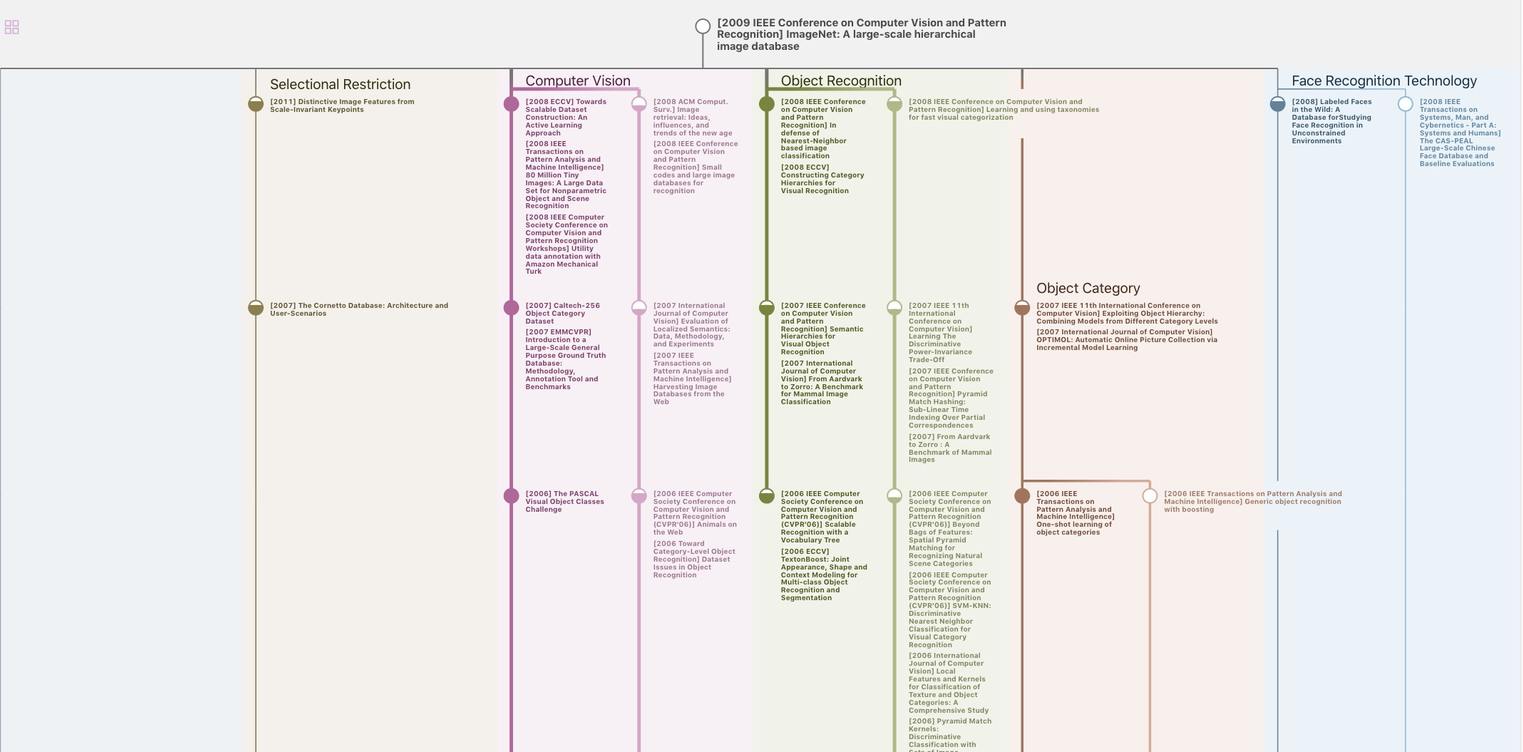
生成溯源树,研究论文发展脉络
Chat Paper
正在生成论文摘要